Towards fully-predictive transport modelling in ASDEX Upgrade H-modes
NUCLEAR FUSION(2021)
摘要
The common way to predict energy confinement in future devices such as ITER is to use scaling laws, based on parameters regression of large cross-machine databases. However, this approach is limited: the regression variables are not purely engineering parameters, physics quantities such as the plasma density n (e) are also input; power regressions fail to capture important physics such as regime transitions; profile effects such as T (i)/T (e) or reverse magnetic shear are not retained. As a consequence, the scatter is large, but even some dependences are known to be of limited validity, such as the n (e) or P dependences of the IPB98(y, 2) scaling. Dimensionless physics, if validated, provides a reliable basis for predicting confinement in a future device. However, there are no experimental data to use as boundary condition, nor empirical knowledge based on that device. Also, the use of full gyro-kinetic codes with all relevant ingredients is computationally unfeasible for a full-radius modelling. Integrated modelling with the recently developed IMEP workflow allows to simulate from the separatrix to the plasma centre, without making use of any experimental input. Although physics-based models are used to predict core transport, core transport is shown to be predicted with less accuracy than the pedestal energy. Therefore, we validate the most established quasi-linear models TGLF and QuaLiKiZ in different regimes and experimental conditions, assessing their strengths and limits. The quasi-linear models are shown to predict transport accurately in a variety of experimental conditions, including dominant ion and electron heating, respectively. The well-known shortcoming near the pedestal top is solved, while a new collision operator in the QuaLiKiZ model improves the electron temperature simulation significantly. Also the Neural Network version of QuaLiKiZ, with very fast computation time, appears to be well applicable in ion heated H-modes.
更多查看译文
关键词
transport,confinement,stiffness,integrated modelling,ITG,TEM,quasi-linear
AI 理解论文
溯源树
样例
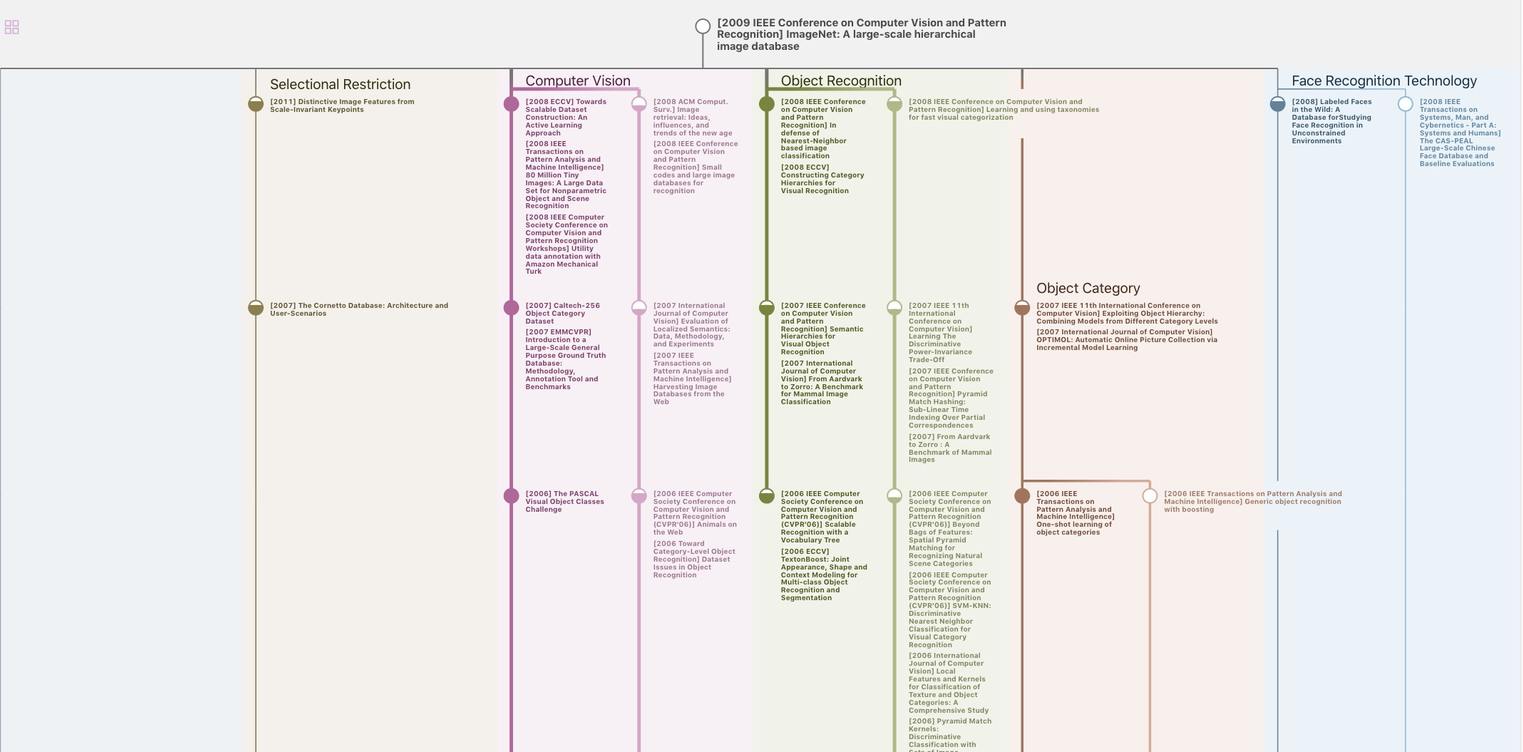
生成溯源树,研究论文发展脉络
Chat Paper
正在生成论文摘要