The development of an AI-based model to predict the location and amount of wax in oil pipelines
Journal of Petroleum Science and Engineering(2022)
摘要
The petroleum that flows within pipelines can contain impurities to form a solid wax which, when aggregated in sufficient qualities within the pipeline, can impair liquid flow and destabilize production. This material buildup is not constant, but rather may be exacerbated by various temperature and pressure conditions. In this study, we designed and tested a system that enables wax diagnosis in oil pipelines. An OLGA simulator was used to build learning data, and the RRR (Ryg, Rydahl, and Ronningen) model was used as a calculation model to describe the molecular diffusion and shear dispersion that most influence wax deposition. A SAE (Stacked Auto-Encoder) was used as the AI (Artificial Intelligence) model after analyzing training loss to select a suitable AI model. The Greedy Layer-Wise method was applied as a learning method to predict various information across all grid sections over time. Our AI model proved to be highly capable by the time a thick layer of wax had formed, with the location of maximum wax buildup being predictable with a great deal of accuracy. But the comparison of predicted wax thickness and actual data is not accurate, which may be the consequence of the small amount of data used for the initial learning. Ultimately, maximum wax volume and the location of maximum wax buildup were predicted with an accuracy of more than 90%. For the maximum wax location, an error of 650 m did appear, however this length corresponds to that of one grid, rendering the error insignificant. This study confirmed that flow assurance can be safeguarded with the application of AI technology, with is both more efficient in terms of time and cost than existing diagnostic methods.
更多查看译文
关键词
Wax deposition,Pipeline flow simulation,Artificial intelligence,Stacked auto-encoder,Greedy Layer-Wise
AI 理解论文
溯源树
样例
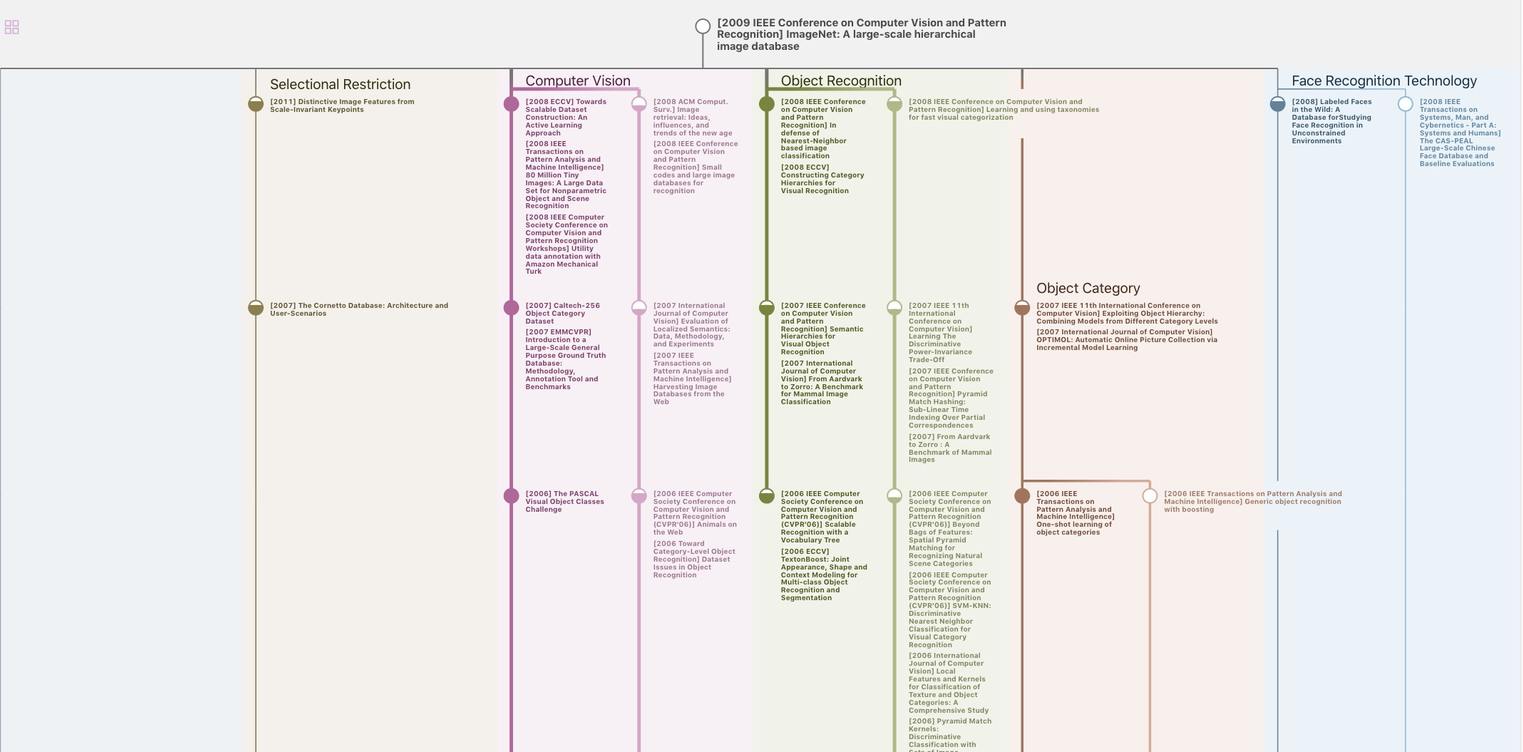
生成溯源树,研究论文发展脉络
Chat Paper
正在生成论文摘要