Supplementary material RFNet: Region-aware Fusion Network for Incomplete Multi-modal Brain Tumor Segmentation
semanticscholar(2021)
摘要
This document supplements the main paper. Firstly, comparisons with the state-of-the-art methods under three other criteria on BRATS2020 are reported in Table 1. Secondly, more comparisons with the state-of-the-art methods (i.e., HeMIS [3], U-HVED [2] and RobustSeg [1]) on BRATS2015 and BRATS2018 are reported in Table 2 and Table 3, respectively. Thirdly, more visual results of RFNet are illustrated in Fig. 1. Furthermore, more qualitative comparisons with the state-of-the-art are illustrated in Fig. 2. Fourthly, illustrations of probability maps of the combinations of different modalities are shown in Fig. 3. Fifthly, more examples of generated attention weights are illustrated in Fig. 4 and Fig. 5. Finally, Figure 6 illustrates the detailed architecture of the encoder and decoder in RFNet. Comparisons under other criteria on BRATS2020: Table 1 report the specificity, sensitivity and Hausdorff distance (95%) on BRATS2020. As shown in Table 1, our RFNet also outperforms the state-of-the-art in terms of the three criteria on BRATS2020. Comparisons on BRATS2015 and BRATS2018: Table 2 and Table 3 report the segmentation accuracy from all modal combinations on BRATS2015 and BRATS2018, respectively. As visible in Table 2 and Table 3, our RFNet is able to improve the accuracy for all the modal combinations significantly on the two datasets. This demonstrates the superiority of our method. Visualization of the predicted segmentation masks: As seen in Fig. 1, our RFNet is able to segment brain tumors in various combinations of modalities. Furthermore, we also compare our RFNet with three state-of-the-art methods qualitatively, as shown in Fig. 2. By comparing the predictions of our RFNet and other methods, we can see that our method predicts more accurate segmentation maps. Visualization of the estimated probability maps: As shown in Fig. 3, our RFNet is able to generate accurate probability maps from various combinations of image modalities. Thus, the probability maps significantly facilitate our region-aware fusion and improve the accuracy of Table 1. Comparisons under three testing criteria on BRATS2020. ’Com’, ’Cor’ and ’Enh’ denotes the whole tumor, the tumor core and the enhancing tumor. Methods specificity (%) ↑ sensitivity (%) ↑ Hausdorff95 (mm) ↓ Com Cor Enh Com Cor Enh Com Cor Enh HeMIS [14] 82.72 66.88 62.40 99.55 99.79 99.77 12.73 13.30 32.84 U-HVED [10] 81.73 68.16 64.09 99.60 99.78 99.78 13.13 16.01 32.69 RobustSeg [3] 88.52 75.70 72.22 99.48 99.78 99.77 18.37 21.34 33.98 Ours 89.42 80.91 73.65 99.60 99.81 99.81 7.88 8.80 25.85
更多查看译文
AI 理解论文
溯源树
样例
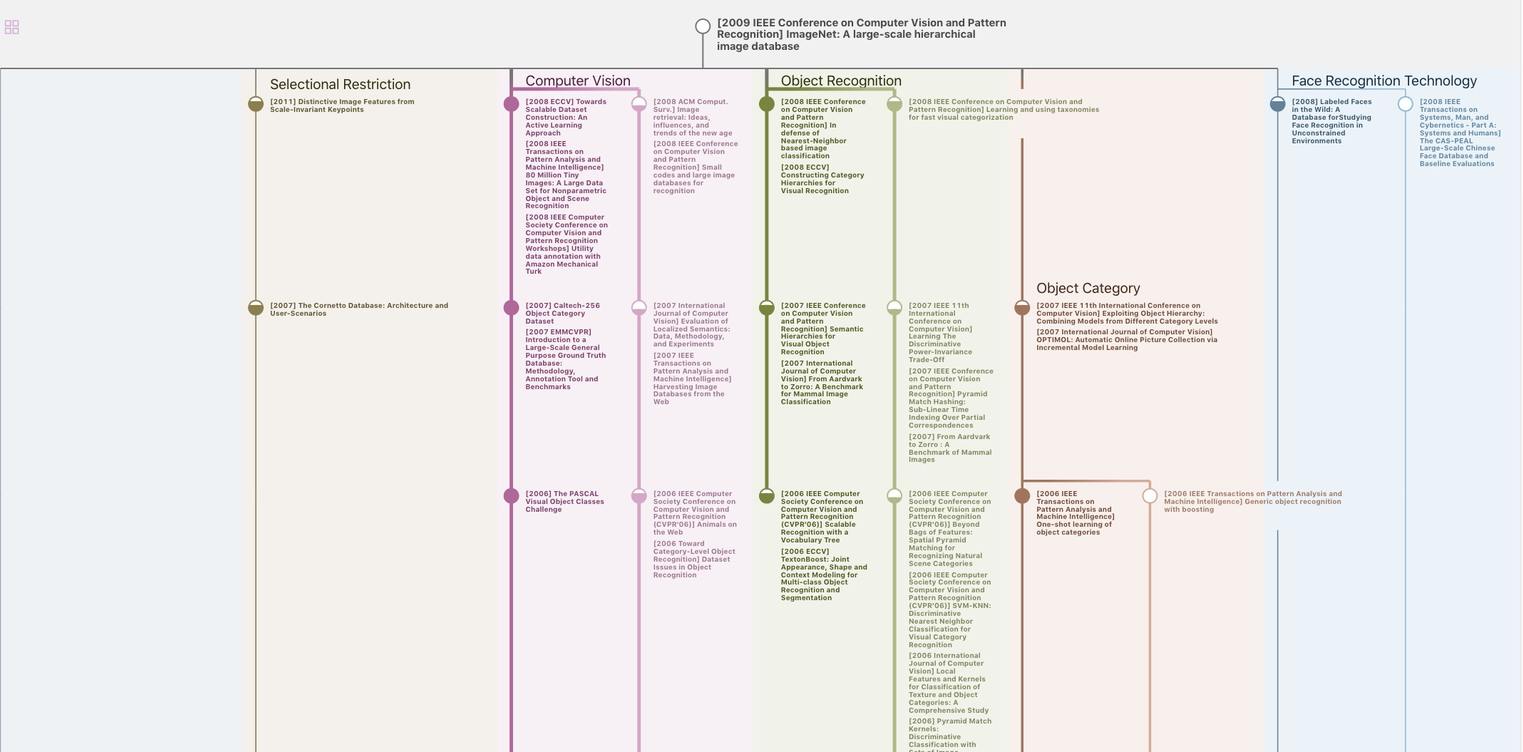
生成溯源树,研究论文发展脉络
Chat Paper
正在生成论文摘要