Unsupervised Feature Selection Method for Mixed Data
semanticscholar(2019)
摘要
In recent years, unsupervised feature selection methods have attracted considerable interest in different areas; this is mainly due to their ability to identify and remove irrelevant and/or redundant features without needing a supervised dataset. However, most of these methods can only process numerical data; so in practical problems in areas such as medicine, economy, business, and social sciences, where it is common that objects are described by numerical and non-numerical features (mixed data), these methods cannot be directly applied. To overcome this limitation, in practice, it is common to apply an encoding method over non-numerical features. Nevertheless, in general, this approach is not a good choice, since by coding data we incorporate a notion of order into the feature values that does not necessarily correspond to the nature of the original dataset. Moreover, the permutation of codes for two values can lead to different distance values, and some mathematical operations do not make sense over the transformed data. For this reason, this Ph.D. research proposal focuses on developing a new unsupervised feature selection method for mixed datasets. Keywords— Feature selection, Unsupervised feature selection, Mixed data
更多查看译文
AI 理解论文
溯源树
样例
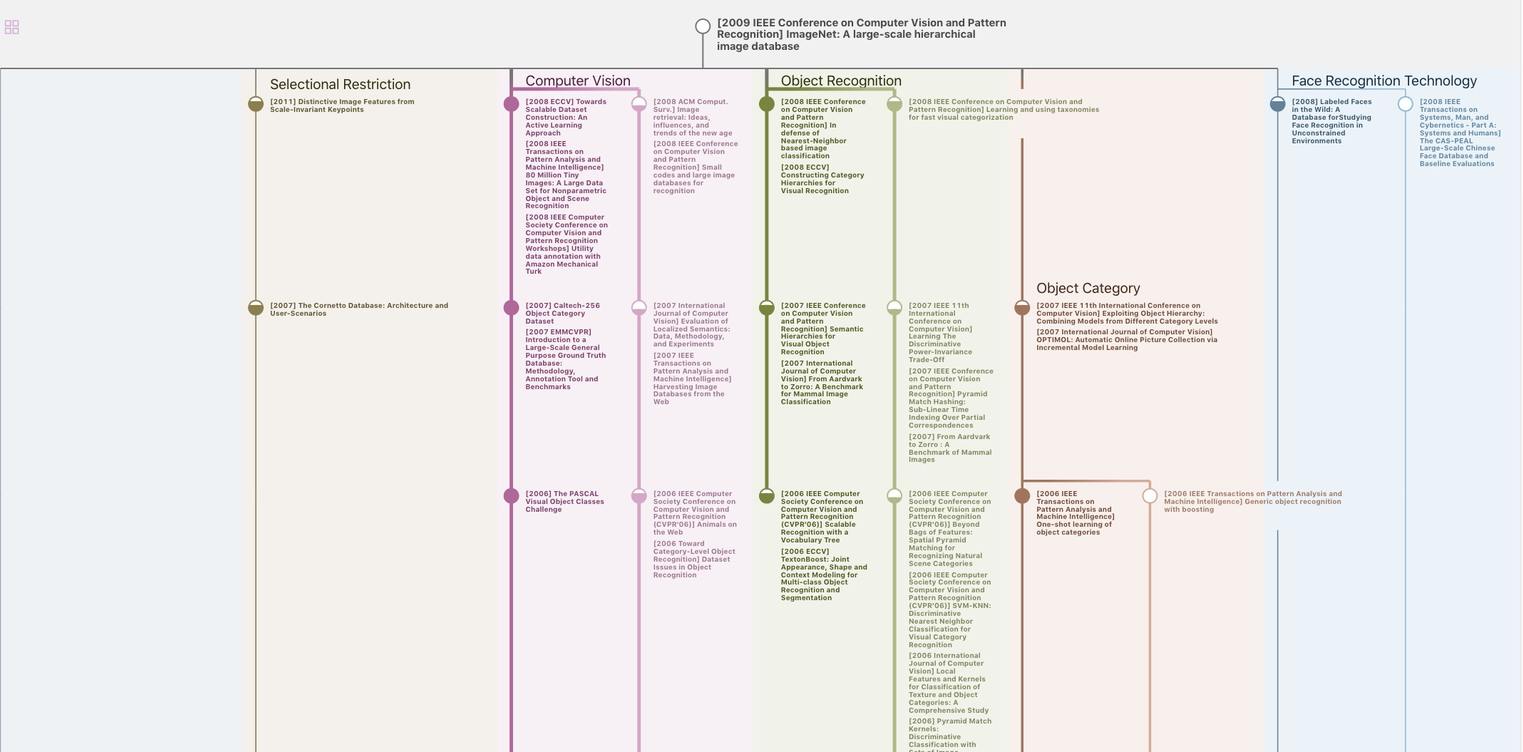
生成溯源树,研究论文发展脉络
Chat Paper
正在生成论文摘要