Toward Meaningful Explanations
semanticscholar(2021)
摘要
ions of the world’s entities we come to experience and know. Facts, in this view are occurrences or states of affairs and may be a descriptive part of an explanation, but not the deep Why. Aristotle’s view, such as in Posterior Analytics provides a more familiar view of explanation as part of a logical, deductive, process using reason to reach conclusions. Aristotle proposed 4 types of causes (αι’τία) to explain things. These were from either the thing’s matter, form, end, or changeinitiator (efficient cause) (Falcon, 2006). Following Descartes, Leibniz and especially Newton, modern deterministic causality using natural mechanisms became central to causal explanations. To know what causes an event means to employ natural laws as the central means to understand and explain why it happened. As this makes clear, some notions of the nature of knowledge, namely, how we come to know something and the nature of reality, are parts of explanation. For example, John Stuart Mill provides a deductivist account of explanation as evidenced by these two quotes: “An individual fact is said to be explained, by pointing out its cause, that is by stating the law or laws of causation, of which its production is an instance,” and “a law or uniformity of nature is said to be explained, when another law or laws are pointed out, of which that law is but a case, and from which it could be deduced (Mill 1843).” While explainability has always be a concern of computer systems, the issue has became especially relevant with the success of artificial intelligence (AI) algorithms, such as deep neural networks, whose functioning is too opaque and complex to be understood easily even by those who developed them. This could limit general acceptance of and trust in these algorithms in spite of their advantages and wide range of applicability. Explainable AI (XAI) is an active research area whose goal is to provide AI systems with some degree of explainability. In “Explainable Artificial Intelligence: An Overview,” Sargur N. Srihari surveys the field of XAI. Explanations provided by XAI methods take a variety of forms, ranging from traditional feature-based explanations to “heat-map” visualizations, from illustrative examples to probabilistic modeling. Clearly, XAI is an exciting new area at the frontiers of AI. When computers were developed, one of the earliest questions was whether they might eventually be as intelligent as humans. The field of AI was created not only to investigate this question but also to actually develop systems that achieved it. A fundamental aspect of human intelligence is that we have “common sense,” and the study of this aspect of intelligence has been a part of AI from the beginning. AI has also always emphasized the benefits of providing explanations for system reasoning While commonsense knowledge (CSK) and its associated reasoning processes would seem to be useful for explainability, CSK research has, until recently, been more concerned with knowledge representation than with explainability. In “Commonsense and Explanation: Synergy and Challenges in the Era of Deep Learning Systems” by Gary Berg-Cross, the connections between CSK and explanations are discussed, including the challenges and opportunities. The goal is to achieve fluid explanations that are responsive to changing circumstances, based on commonsense knowledge about the world. The healthcare enterprise involves many different stakeholders – consumers, healthcare professionals and providers, researchers, and insurers. Sources of health related data are highly diverse and have many levels of granularity. As a result of the COVID-19 pandemic, healthcare issues that were previously only discussed by specialists are now part of the everyday discourse of the average individual. In “Applied Ontologies for Global Health Surveillance and Pandemic Intelligence,” Christopher J. O. Baker, Mohammad Sadnan Al Manir, Jon Hael Brenas, Kate Zinszer, and Arash Shaban-Nejad use Malaria surveillance as a use case to highlight the contribution of applied ontologies for enhancing enhanced interoperability, interpretability and explainability. These technologies are relevant for ongoing pandemic preparedness initiatives. Financial institutions are very complex entities that play many roles and have many kinds of stakeholders, ranging from customers, to regulators, to shareholders, and to the society as a whole. Given these many responsibilities, it is no surprise that financial institutions “have a lot of explaining to do,” as Michael Bennett so deftly begins his article “Financial Industry Explanation” where he presents some of the challenges of providing meaningful explanation in this domain. Explanations are a special case of the more general requirement of accountability which is becoming an issue for many other domains as well. The lessons learned by the financial industry explainability are likely to be valuable for other domains as well. Ontologies play a significant role in all of the many research projects referenced by papers in this special issue. However, the ontologies for explainability in XAI, commonsense reasoning, health surveillance, and finance do not seem to have much in common with one another. The final paper, “Decision Rationales as Models for Explanations” by Kenneth Baclawski, attempts to weave the various strands of ontologies for explainability together in a single reference ontology by focusing on the observation that the purpose of most of the systems is to make decisions, and that it is the decisions that need to be explained. Processes today, whether they are based on software or human activities or a combination of them, or whether they use legacy systems or newly developed systems seldom include explainability. In nearly all cases, explanations are neither recorded nor can be easily generated. Unfortunately, explainability cannot simply be added as another module. Rather it should drive every process from the earliest stages of planning, analysis and design. Explainability requirements must be empirically discovered during these stages (Clancey 2019). Unfortunately, currently there is little sensitivity to the need for explainability and little experience with addressing it. It is hoped that this special issue will assist stakeholders to develop their systems so that they provide meaningful explanations.
更多查看译文
AI 理解论文
溯源树
样例
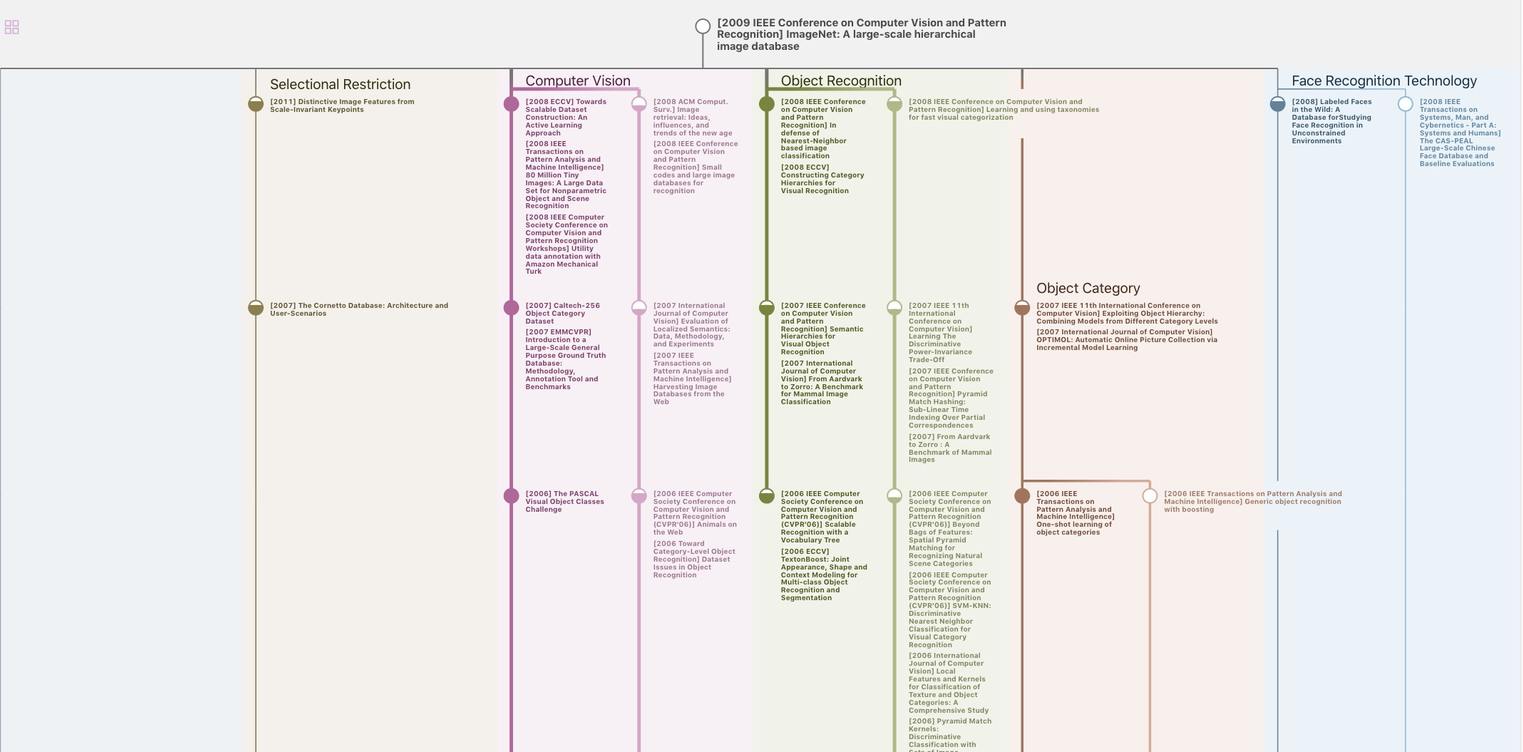
生成溯源树,研究论文发展脉络
Chat Paper
正在生成论文摘要