The Effect of Hyperparameter Tuning on the Comparative Evaluation of Unsupervised Anomaly Detection Methods
semanticscholar(2021)
摘要
Anomaly detection aims at finding observations in a dataset that do not conform to expected behavior. Researchers have proposed a large variety of anomaly detection algorithms and their performance is greatly affected by how a user sets each algorithm’s hyperparameters. However, the anomaly detection literature does not agree on how to set these hyperparameters when experimentally comparing different algorithms. Most papers compare either performance using “default” settings, or maximal performance under optimal settings. In this paper, we argue that both strategies fail to capture what practitioners are actually interested in: how well does the algorithm perform in practice? They are either too pessimistic, assuming no tuning, or unrealistically optimistic, assuming optimal tuning; and they often result in methodologically unsound and irreproducible comparisons between algorithms. We therefore propose to use a small validation set to tune an anomaly detector’s hyperparameters on a per dataset basis. We argue this is realistic, striking the balance between keeping the cost of acquiring labeled data low and selecting the hyperparameters in a fair, sound, and reproducible manner. We provide a theoretical lower bound on the validation set size based on probability of an anomaly detector achieving a higher area under the ROC curve than a random detector. Using a benchmark of 16 datasets, we experimentally show that different ∗Both authors contributed equally to the paper Permission to make digital or hard copies of all or part of this work for personal or classroom use is granted without fee provided that copies are not made or distributed for profit or commercial advantage and that copies bear this notice and the full citation on the first page. Copyrights for components of this work owned by others than ACM must be honored. Abstracting with credit is permitted. To copy otherwise, or republish, to post on servers or to redistribute to lists, requires prior specific permission and/or a fee. Request permissions from permissions@acm.org. ODD ’21, August 15, 2021, Virtual © 2021 Association for Computing Machinery. ACM ISBN 978-x-xxxx-xxxx-x/YY/MM. . . $15.00 https://doi.org/10.1145/nnnnnnn.nnnnnnn hyperparameter selection strategies lead to different conclusions about which algorithms perform better than others, and that using a small validation set is a practically feasible and principled way of tuning the hyperparameters for a given dataset.
更多查看译文
AI 理解论文
溯源树
样例
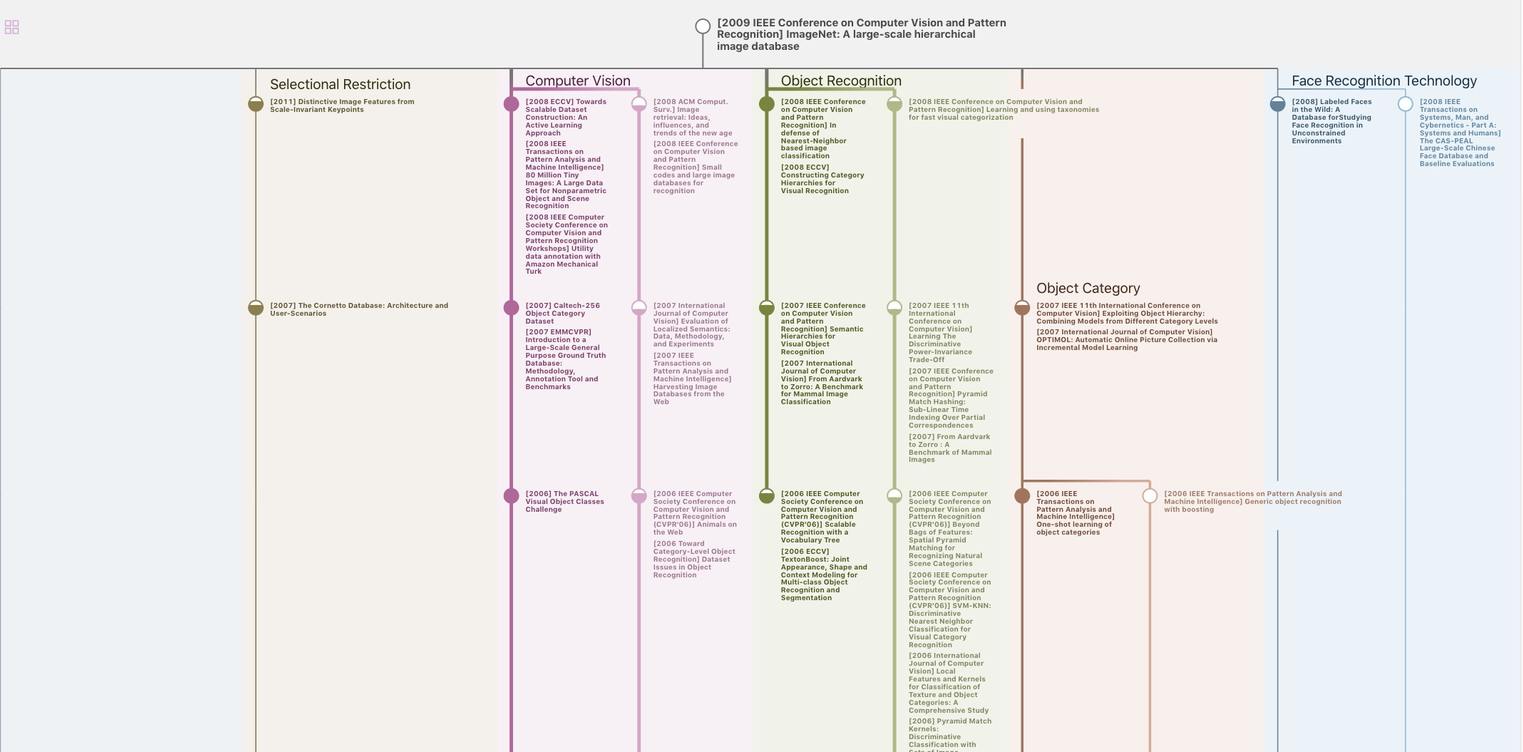
生成溯源树,研究论文发展脉络
Chat Paper
正在生成论文摘要