Quantum speed-ups in reinforcement learning
NanoScience + Engineering(2021)
摘要
As the field of artificial intelligence is pushed forward, the question arises of how fast autonomous machines can learn. Within artificial intelligence, an important paradigm is reinforcement learning, where agents - learning entities capable of decision making - interact with the world they are placed in, called an environment. Thanks to these interactions, agents receive feedback from the environment and thus progressively adjust their behaviour to accomplish a given goal. An important question in reinforcement learning is how fast agents can learn to fulfill their tasks. To answer this question we consider a novel reinforcement learning framework where quantum mechanics is used. In particular, we quantize the agent and the environment and grant them the possibility to also interact quantum-mechanically, that is, by using a quantum channel for their communication. We demonstrate that this feature enables a speed-up in the agent's learning process, and we further show that combining this scenario with classical communication enables the evaluation of such an improvement. This learning protocol is implemented on an integrated re-programmable photonic platform interfaced with photons at telecommunication wavelengths. Thanks to the full tunability of the device, this platform proves the best candidate for the implementation of learning protocols, where a continuous update of the learning process is required.
更多查看译文
AI 理解论文
溯源树
样例
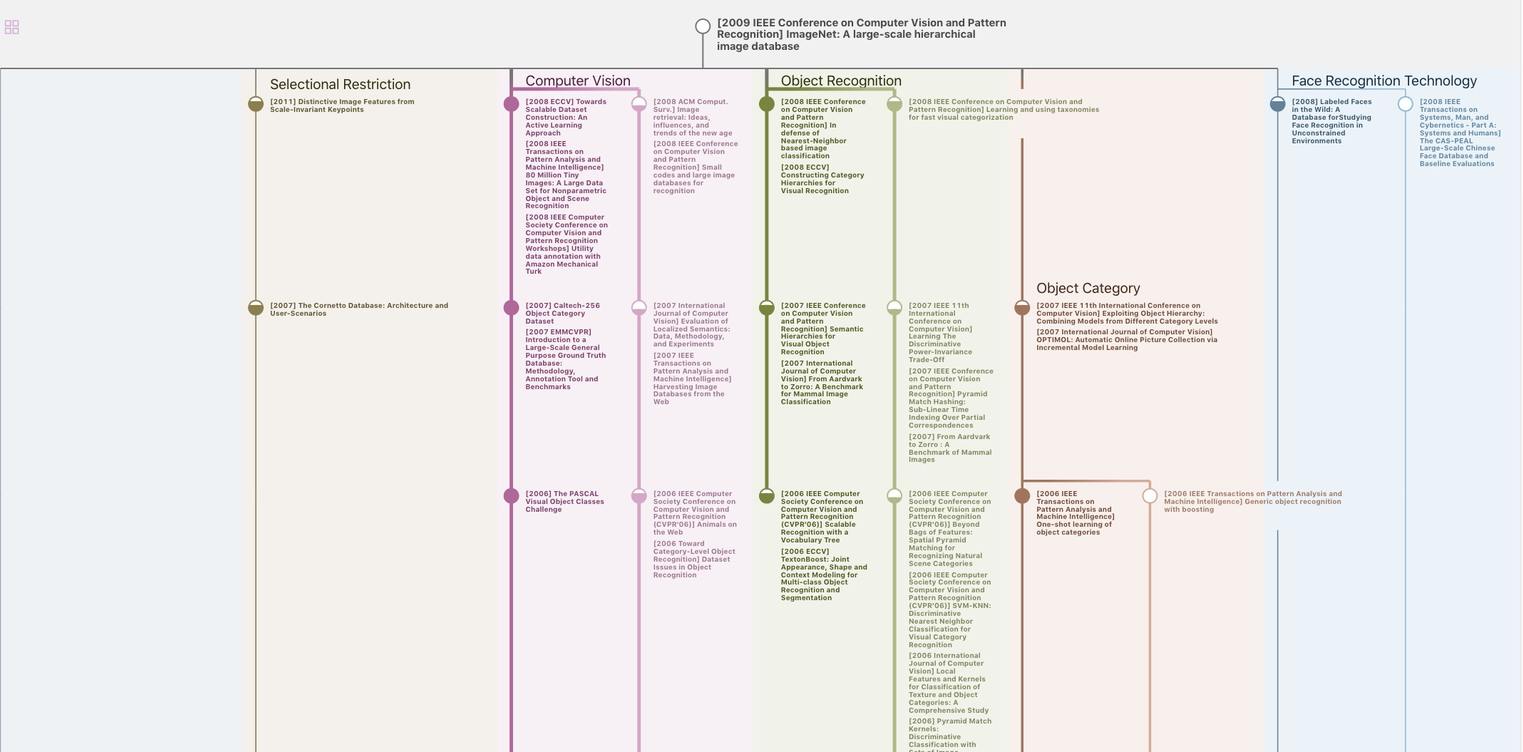
生成溯源树,研究论文发展脉络
Chat Paper
正在生成论文摘要