Evaluating Adoption, Impact, and Factors Driving Adoption for TREWS, a Machine Learning-Based Sepsis Alerting System
medRxiv(2021)
摘要
Machine learning-based clinical decision support tools for sepsis create opportunities to identify at-risk patients and initiate treatments earlier, an important step in improving sepsis outcomes. Increasing use of such systems means quantifying and understanding provider adoption is critical. Using real-time provider interactions with a sepsis early detection tool (Targeted Real-time Early Warning System) deployed at five hospitals over a two-year period (469,419 screened patient encounters, 9,805 (2.1%) of which were retrospectively identified as having sepsis), we found high adoption rates (89% of alerts were evaluated by a physician or advanced practice provider) and an association between use of the tool and earlier treatment of sepsis patients (1.85 (95% CI: 1.66 - 2.00) hour reduction in median time to first antibiotics order). Further, we found that provider-related factors had the strongest association with alert adoption and that case complexity and atypical presentation were associated with dismissal of alerts on sepsis patients. Beyond improving the performance of the system, efforts to improve adoption should focus on provider knowledge, experience, and perceptions of the system.
更多查看译文
AI 理解论文
溯源树
样例
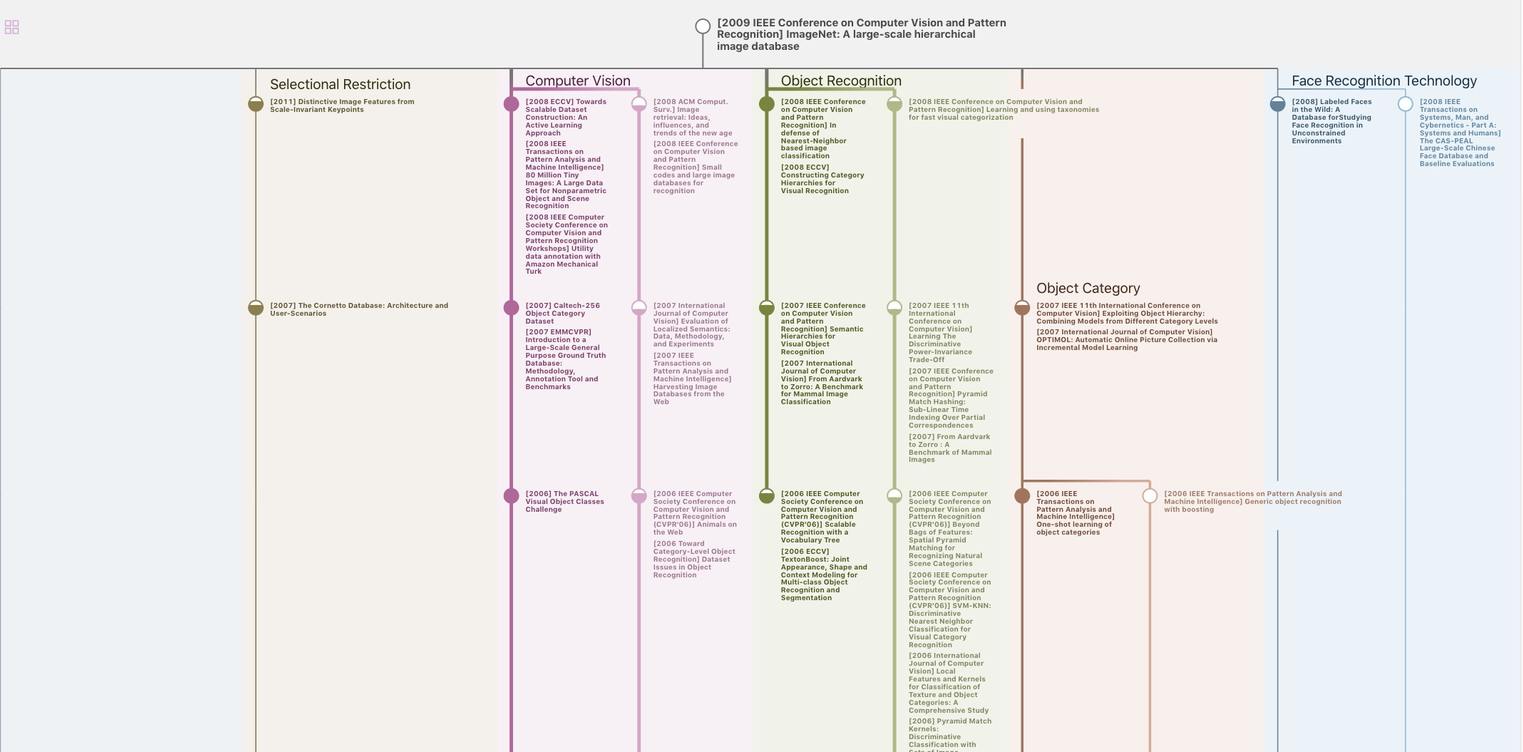
生成溯源树,研究论文发展脉络
Chat Paper
正在生成论文摘要