LOP-OCR: A Language-Oriented Pipeline for Large-chunk Text OCR
semanticscholar(2019)
摘要
Optical character recognition (OCR) for largechunk texts (e.g., annuals, legal contracts, research reports, scientific papers) is of growing interest. It serves as a prerequisite for further text processing. Standard Scene Text Recognition tasks in computer vision mostly focus on detecting text bounding boxes, but rarely explore how NLP models can be of help. It is intuitive that NLP models can significantly help large-chunk text OCR. In this paper, we propose LOP-OCR, a languageoriented pipeline tailored to this task. The key part of LOP-OCR is an error correction model that specifically captures and corrects OCR errors. The correction model is based on SEQ2SEQ models with auxiliary image information to learn the mapping between OCR errors and supposed output characters, and is able to significantly reduce OCR error rate. LOP-OCR is able to significantly improve the performance of the CRNN-based OCR models, increasing sentence-level accuracy from 77.9 to 88.9, position-level accuracy from 91.8 to 96.5 and BLEU scores from 88.4 to 93.3.1
更多查看译文
AI 理解论文
溯源树
样例
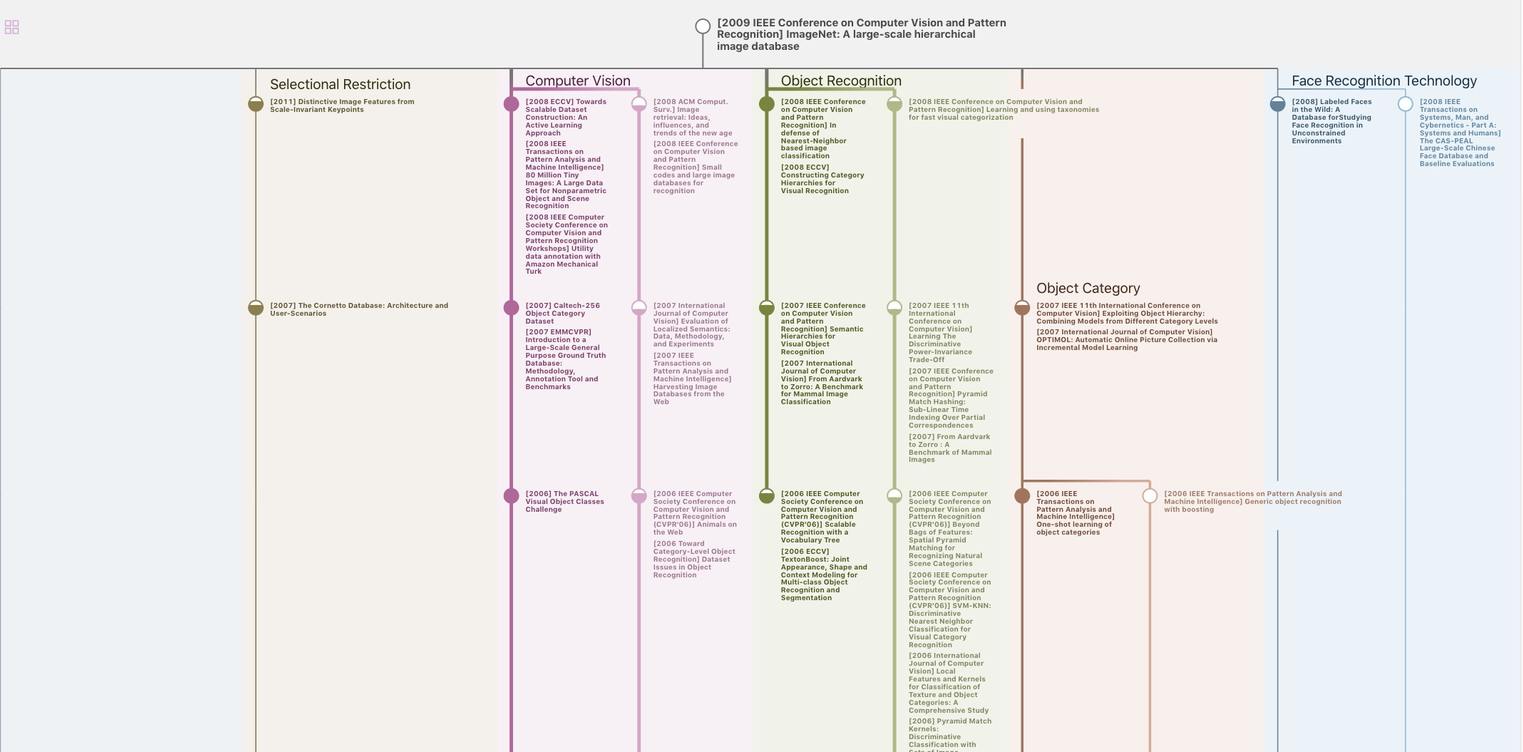
生成溯源树,研究论文发展脉络
Chat Paper
正在生成论文摘要