Federated learning optimization techniques for non-iid data: a review
semanticscholar(2020)
摘要
Federated learning is a distributed machine learning setting that can effectively assist multiple clients (e.g. mobile phones, IoT devices and organizations) to conduct isolated data use and machine learning modeling in accordance with user privacy protection, data security and government regulations. The participants can benefit from the federated learning training process or outcomes without sharing any local raw data. However, this special structure also determines that federated learning is bound to encounter statistical challenge which principally performs as non-independently and identically distributed (non-IID) data problem. This issue can directly affect the performance of federated learning, resulting in inferior performance of machine learning models, like poor prediction accuracy, additional communication overhead and low convergence rate. Therefore, it is of great significance for federated learning to study this non-IID data issue. At present, many optimization techniques have been proposed to tackle this problem, nonetheless they are various and lack a unified classification standard. To provide that standard, this paper reviews and classifies the optimization approaches which tackle this issue of federated learning, and summarizes the characteristics of each type of method based on several major influencing factors.
更多查看译文
AI 理解论文
溯源树
样例
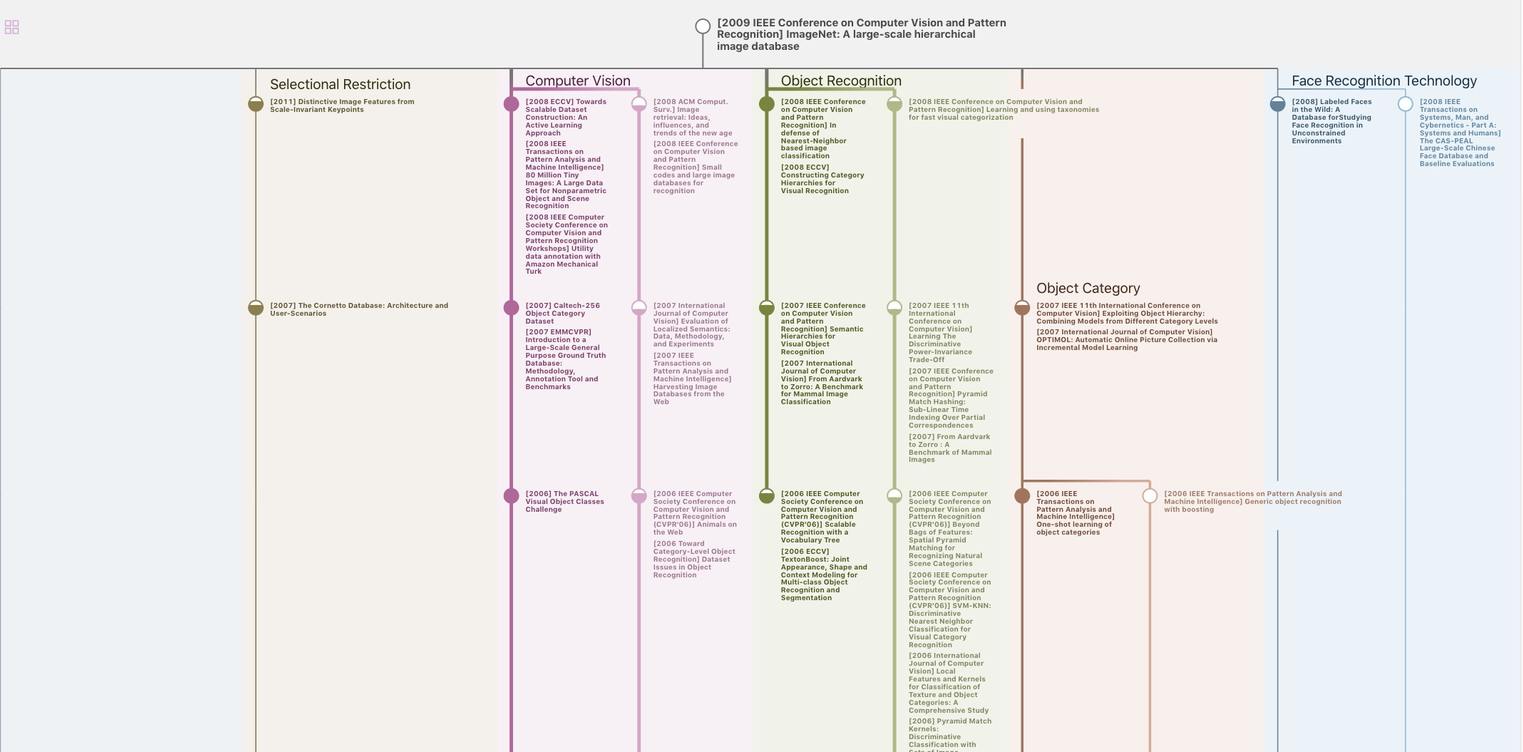
生成溯源树,研究论文发展脉络
Chat Paper
正在生成论文摘要