Kynurenine and Hemoglobin as Sex-Specific Variables in COVID-19 Patients: A Machine Learning and Genetic Algorithms Approach
DIAGNOSTICS(2021)
摘要
Differences in clinical manifestations, immune response, metabolic alterations, and outcomes (including disease severity and mortality) between men and women with COVID-19 have been reported since the pandemic outbreak, making it necessary to implement sex-specific biomarkers for disease diagnosis and treatment. This study aimed to identify sex-associated differences in COVID-19 patients by means of a genetic algorithm (GALGO) and machine learning, employing support vector machine (SVM) and logistic regression (LR) for the data analysis. Both algorithms identified kynurenine and hemoglobin as the most important variables to distinguish between men and women with COVID-19. LR and SVM identified C10:1, cough, and lysoPC a 14:0 to discriminate between men with COVID-19 from men without, with LR being the best model. In the case of women with COVID-19 vs. women without, SVM had a higher performance, and both models identified a higher number of variables, including 10:2, lysoPC a C26:0, lysoPC a C28:0, alpha-ketoglutaric acid, lactic acid, cough, fever, anosmia, and dysgeusia. Our results demonstrate that differences in sexes have implications in the diagnosis and outcome of the disease. Further, genetic and machine learning algorithms are useful tools to predict sex-associated differences in COVID-19.
更多查看译文
关键词
COVID-19, sex, machine learning, metabolomics
AI 理解论文
溯源树
样例
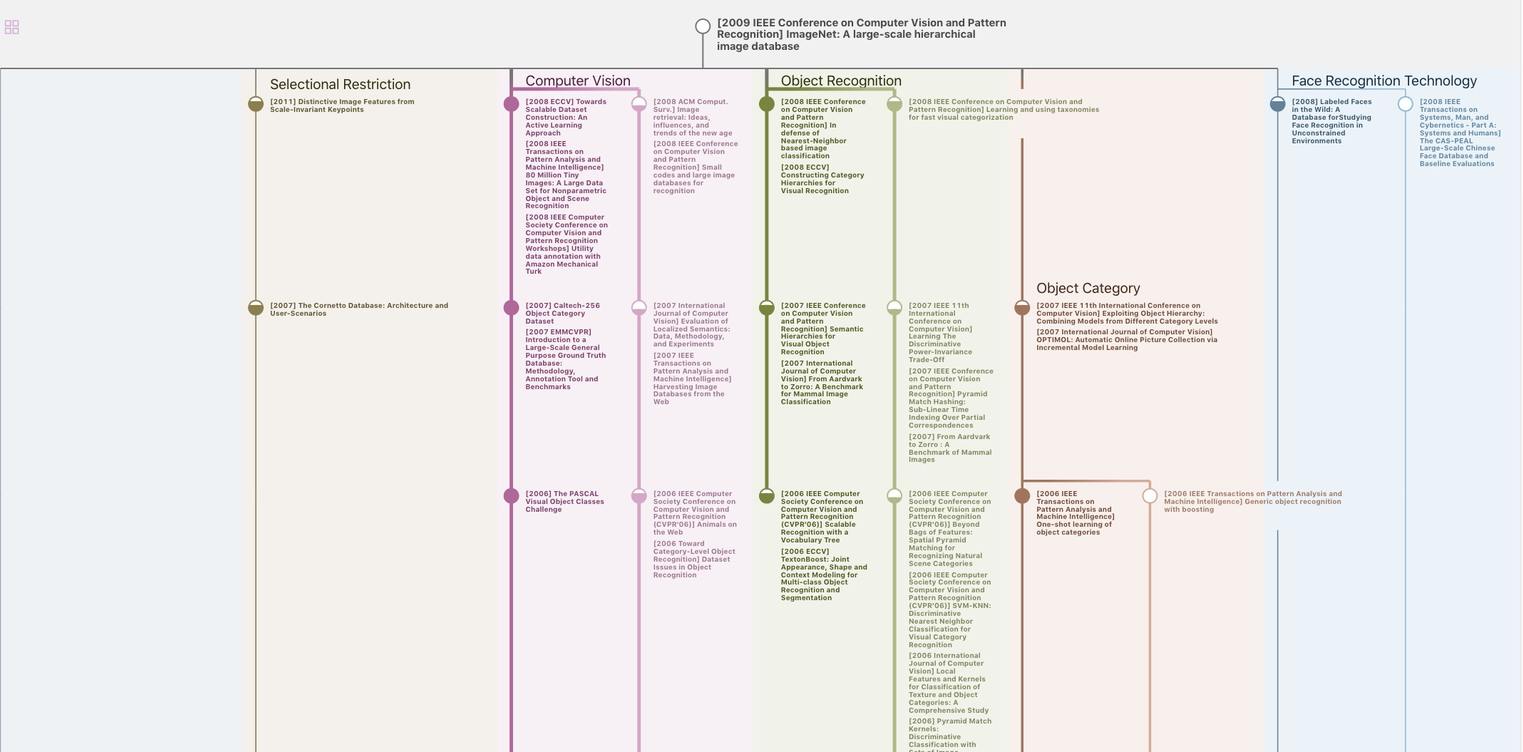
生成溯源树,研究论文发展脉络
Chat Paper
正在生成论文摘要