Bayesian Continuous-Time Hidden Markov Models With Covariate Selection for Intensive Longitudinal Data With Measurement Error
Psychological methods(2023)
摘要
Translational Abstract Continuous-time hidden Markov models enable researchers to study the relations between risk factors and outcomes repeatedly measured at unbalanced, unequally spaced assessment times while accommodating measurement error. Despite extensive implementation of variable selection methods designed to identify potential relations in exploratory settings for hypothesis generation, currently none have been applied to this class of models commonly found in psychological research. To fill this gap, we develop a Bayesian continuous-time hidden Markov model with variable selection priors and provide a flexible R package to facilitate the application of our method in practice. We showcase the variable selection performance and estimation accuracy of our method on simulated data and apply it to intensive longitudinal data collected in a smoking cessation trial to identify potential risk factors associated with smoking behaviors after a quit attempt. Intensive longitudinal data collected with ecological momentary assessment methods capture information on participants' behaviors, feelings, and environment in near real-time. While these methods can reduce recall biases typically present in survey data, they may still suffer from other biases commonly found in self-reported data (e.g., measurement error and social desirability bias). To accommodate potential biases, we develop a Bayesian hidden Markov model to simultaneously identify risk factors for subjects transitioning between discrete latent states as well as risk factors potentially associated with them misreporting their true behaviors. We use simulated data to demonstrate how ignoring potential measurement error can negatively affect variable selection performance and estimation accuracy. We apply our proposed model to smartphone-based ecological momentary assessment data collected within a randomized controlled trial that evaluated the impact of incentivizing abstinence from cigarette smoking among socioeconomically disadvantaged adults.
更多查看译文
关键词
Bayesian variable selection,hidden Markov model,measurement error,mHealth,smoking cessation
AI 理解论文
溯源树
样例
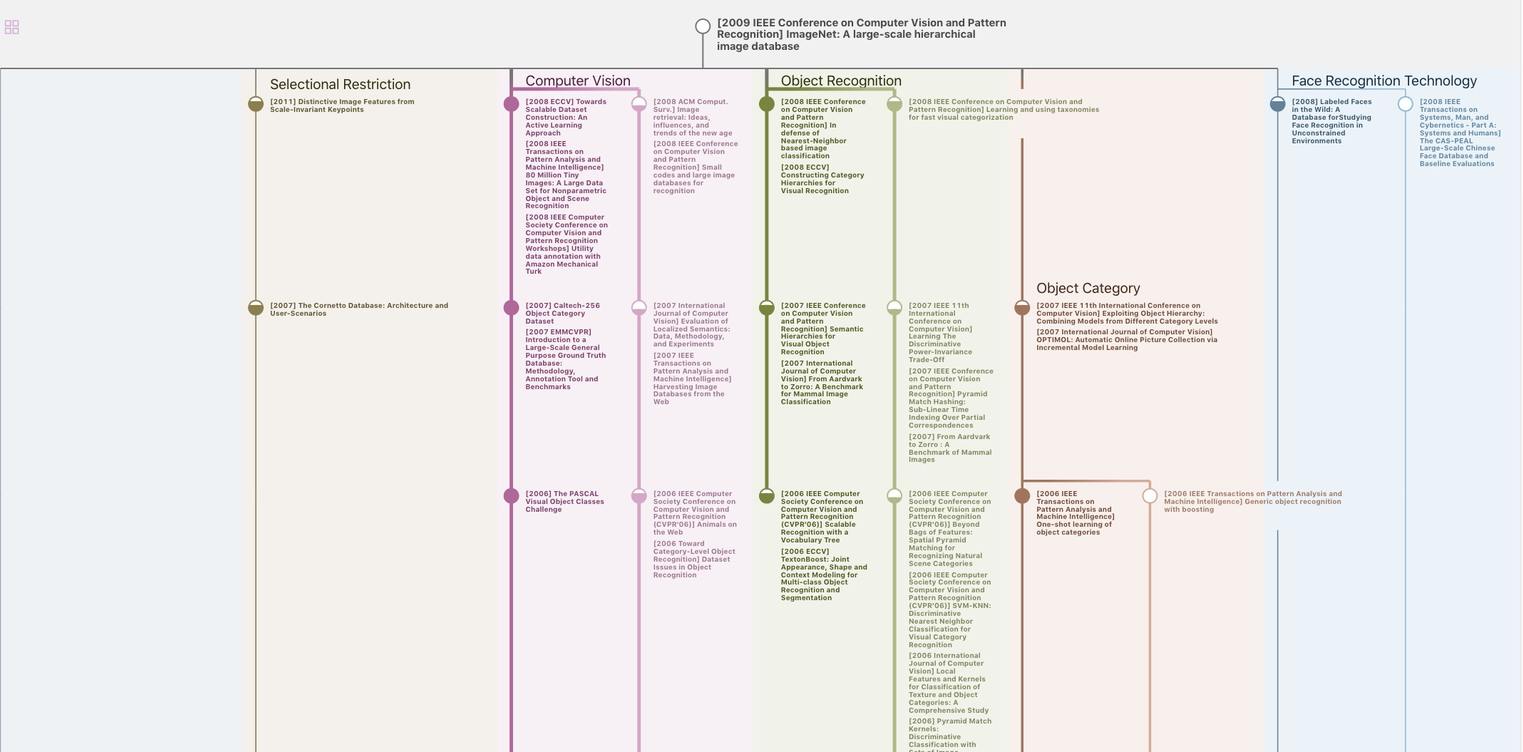
生成溯源树,研究论文发展脉络
Chat Paper
正在生成论文摘要