Neural symbolic reasoning with knowledge graphs: Knowledge extraction, relational reasoning, and inconsistency checking
Fundamental Research(2021)
摘要
Knowledge graphs (KGs) express relationships between entity pairs, and many real-life problems can be formulated as knowledge graph reasoning (KGR). Conventional approaches to KGR have achieved promising performance but still have some drawbacks. On the one hand, most KGR methods focus only on one phase of the KG lifecycle, such as KG completion or refinement, while ignoring reasoning over other stages, such as KG extraction. On the other hand, traditional KGR methods, broadly categorized as symbolic and neural, are unable to balance both scalability and interpretability. To resolve these two problems, we take a more comprehensive perspective of KGR with regard to the whole KG lifecycle, including KG extraction, completion, and refinement, which correspond to three subtasks: knowledge extraction, relational reasoning, and inconsistency checking. In addition, we propose the implementation of KGR using a novel neural symbolic framework, with regard to both scalability and interpretability. Experimental results demonstrate that our proposed methods outperform traditional neural symbolic models.
更多查看译文
关键词
Neural symbolic reasoning,Knowledge graph,Knowledge extraction,Relational reasoning,Inconsistency checking
AI 理解论文
溯源树
样例
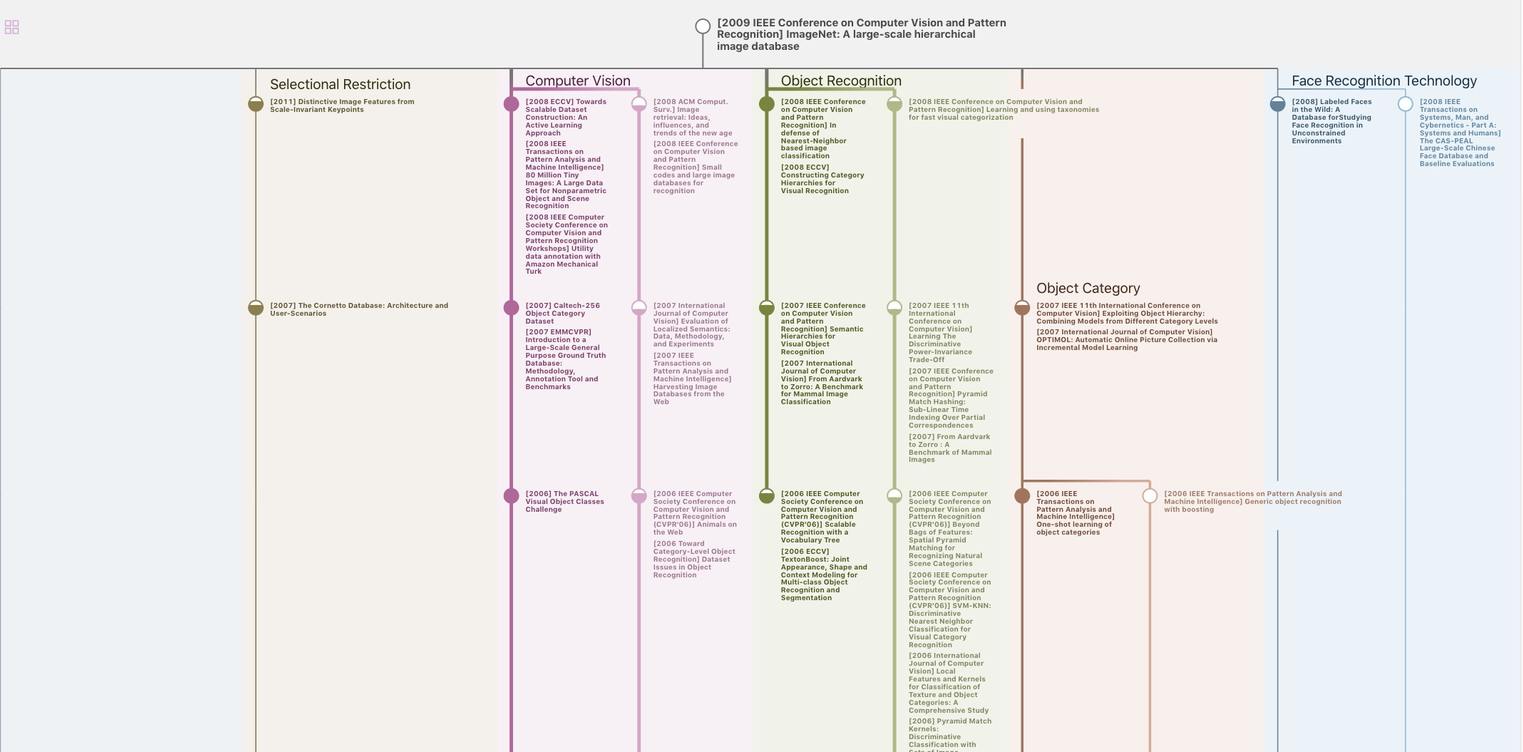
生成溯源树,研究论文发展脉络
Chat Paper
正在生成论文摘要