A new clustering mining algorithm for multi-source imbalanced location data
Information Sciences(2022)
摘要
In the era of big data, clustering based on multi-source data fusion has become a hot topic in data mining field. Existing studies mainly focus on fusion models and algorithms of data sets in the same domain, but few studies consider imbalanced data sets from different domains. Furthermore, studies on imbalanced data sets mostly focus on classification and less on clustering problems. Therefore, we propose a novel clustering algorithm for mining fused location data. This algorithm can deal with imbalanced data sets with large density differences, find clusters generated by the minority class data, and reduce the time complexity of the clustering process. Since current evaluation indices are not suitable for evaluating clustering results of imbalanced data sets, we present a new comprehensive evaluation metric used in the clustering validity judgment. Urban hotspots mining is used as an example, and the effectiveness of the proposed method is validated using GPS trajectory data from the transport domain and check-in data from the social network. The experimental results demonstrate that the performance of the proposed algorithm outperforms that of the state-of-the-art clustering algorithms, and it can simultaneously discover urban hotspots formed by the majority and minority class data.
更多查看译文
关键词
Imbalanced data set,Data fusion,Clustering algorithm,Clustering quality,Adaptive grid partition,Urban hotspots
AI 理解论文
溯源树
样例
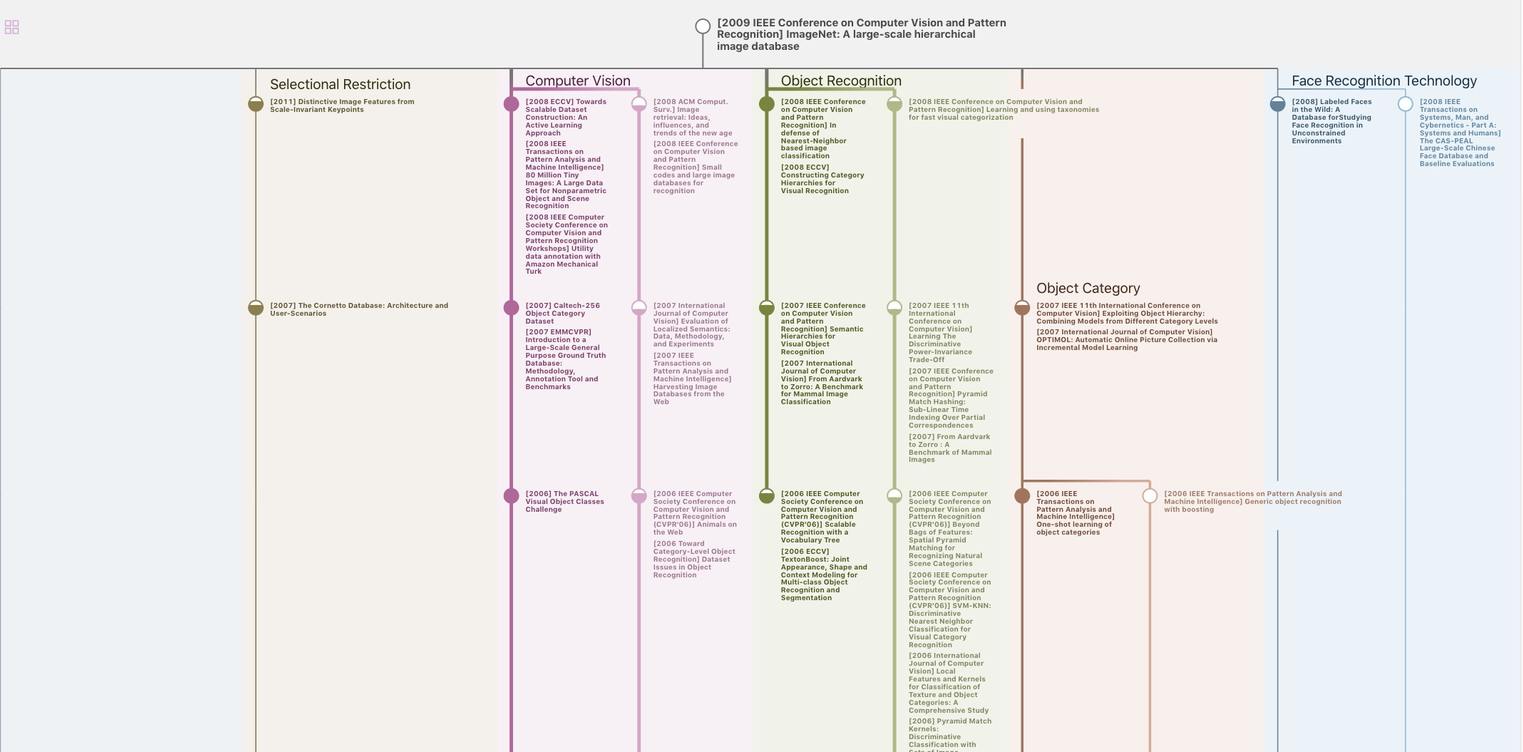
生成溯源树,研究论文发展脉络
Chat Paper
正在生成论文摘要