Automated segmentation of epidermis in high-frequency ultrasound of pathological skin using a cascade of DeepLab v3+networks and fuzzy connectedness
COMPUTERIZED MEDICAL IMAGING AND GRAPHICS(2022)
摘要
This study proposes a novel, fully automated framework for epidermal layer segmentation in different skin diseases based on 75 MHz high-frequency ultrasound (HFUS) image data. A robust epidermis segmentation is a vital first step to detect changes in thickness, shape, and intensity and therefore support diagnosis and treatment monitoring in inflammatory and neoplastic skin lesions. Our framework links deep learning and fuzzy connectedness for image analysis. It consists of a cascade of two DeepLab v3+ models with a ResNet-50 backbone and a fuzzy connectedness analysis module for fine segmentation. Both deep models are pre-trained on the ImageNet dataset and subjected to transfer learning using our HFUS database of 580 images with atopic dermatitis, psoriasis and non-melanocytic skin tumors. The first deep model is used to detect the appropriate region of interest, while the second stands for the main segmentation procedure. We use the softmax layer of the latter twofold to prepare the input data for fuzzy connectedness analysis: as a reservoir of seed points and a direct contribution to the input image. In the experiments, we analyze different configurations of the framework, including region of interest detection, deep model backbones and training loss functions, or fuzzy connectedness analysis with parameter settings. We also use the Dice index and epidermis thickness to compare our results to state-of-the-art approaches. The Dice index of 0.919 yielded by our model over the entire dataset (and exceeding 0.93 in inflammatory diseases) proves its superiority over the other methods.
更多查看译文
关键词
Dermatology, Inflammatory diseases, Skin tumor, High -frequency ultrasound, Image segmentation, Deep learning, Fuzzy connectedness
AI 理解论文
溯源树
样例
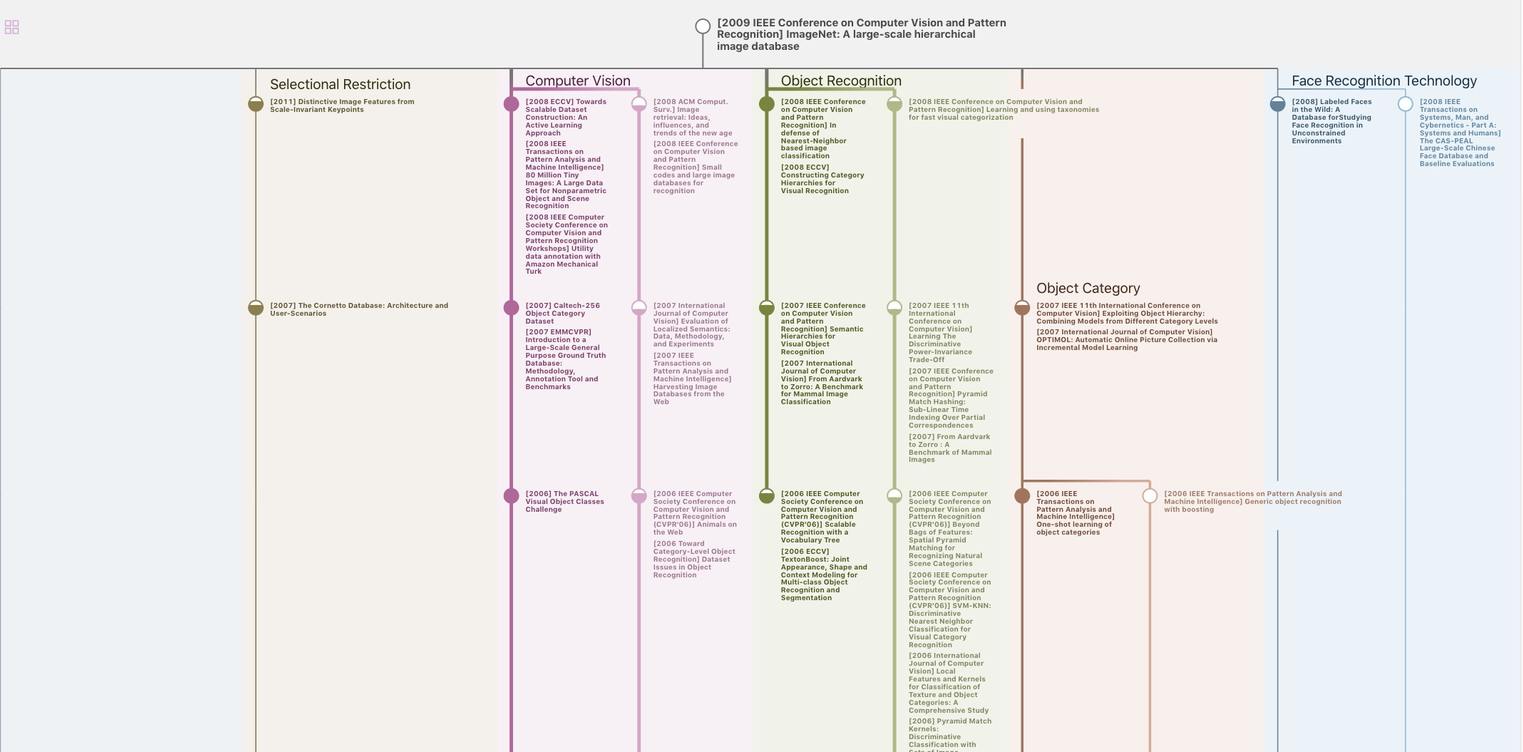
生成溯源树,研究论文发展脉络
Chat Paper
正在生成论文摘要