Turbo Similarity Searching: Effect of Partial Ranking and Fusion Rules on ChEMBL Database
MOLECULAR INFORMATICS(2022)
摘要
Turbo Similarity Searching (TSS) is the simplest and most recent chemical similarity searching (SS) approach, which improves the effectiveness of SS by performing a multi-target searching. TSS has four important elements, namely structural representation, similarity coefficient, number of nearest neighbours (NNs), and fusion rule, and any changes in these elements could affect the TSS results. A previous study suggested the advantage of using large numbers of reference compounds with small fractions of the database structures to obtain a better recall in group fusion. Therefore, this study aims to investigate the effect of partial ranking on TSS utilising different fusion rules and different numbers of NNs on the ChEMBL database and to evaluate whether these observations hold in TSS. Furthermore, the objective is to observe the effect of the indirect relationship feature of TSS on the partial ranking investigation. The results showed that the effect of using partial ranking on TSS was significant. This study also found that the performance of TSS improved as the database proportions used in the fusion process decreased and by using a small number of NNs. In addition, fusion rules based on reciprocal rank positions (RKP), maximum similarity score (sMAX), and sMNZ were superior to all the other fusion rules.
更多查看译文
关键词
ChEMBL database, Drug discovery, Fusion rule, Turbo similarity searching, Virtual screening
AI 理解论文
溯源树
样例
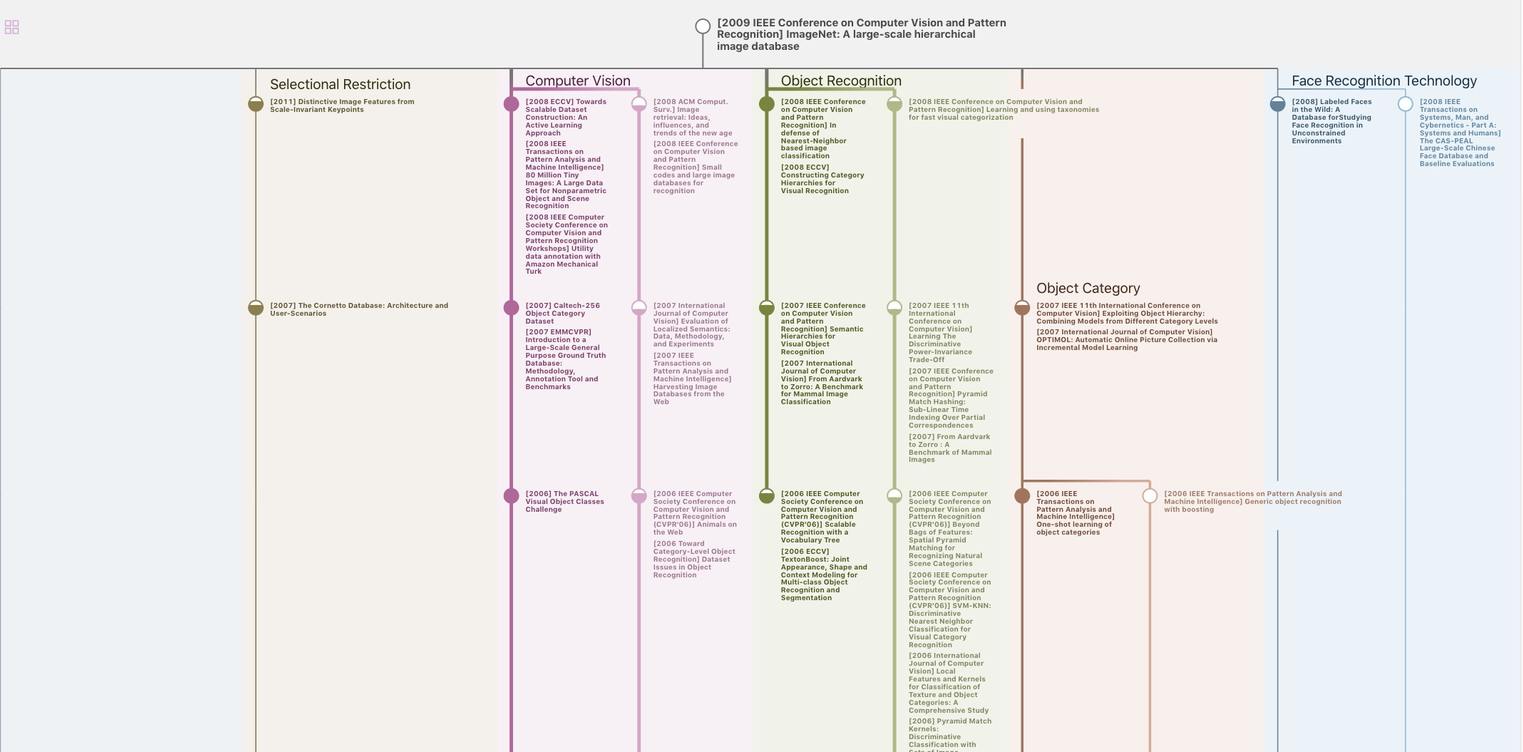
生成溯源树,研究论文发展脉络
Chat Paper
正在生成论文摘要