A Novel Prediction Setup for Online Speed-Scaling.
Scandinavian Workshop on Algorithm Theory (SWAT)(2022)
摘要
Given the rapid rise in energy demand by data centers and computing systems in general, it is fundamental to incorporate energy considerations when designing (scheduling) algorithms. Machine learning can be a useful approach in practice by predicting the future load of the system based on, for example, historical data. However, the effectiveness of such an approach highly depends on the quality of the predictions and can be quite far from optimal when predictions are sub-par. On the other hand, while providing a worst-case guarantee, classical online algorithms can be pessimistic for large classes of inputs arising in practice. This paper, in the spirit of the new area of machine learning augmented algorithms, attempts to obtain the best of both worlds for the classical, deadline based, online speed-scaling problem: Based on the introduction of a novel prediction setup, we develop algorithms that (i) obtain provably low energy-consumption in the presence of adequate predictions, and (ii) are robust against inadequate predictions, and (iii) are smooth, i.e., their performance gradually degrades as the prediction error increases.
更多查看译文
关键词
novel prediction setup,speed-scaling
AI 理解论文
溯源树
样例
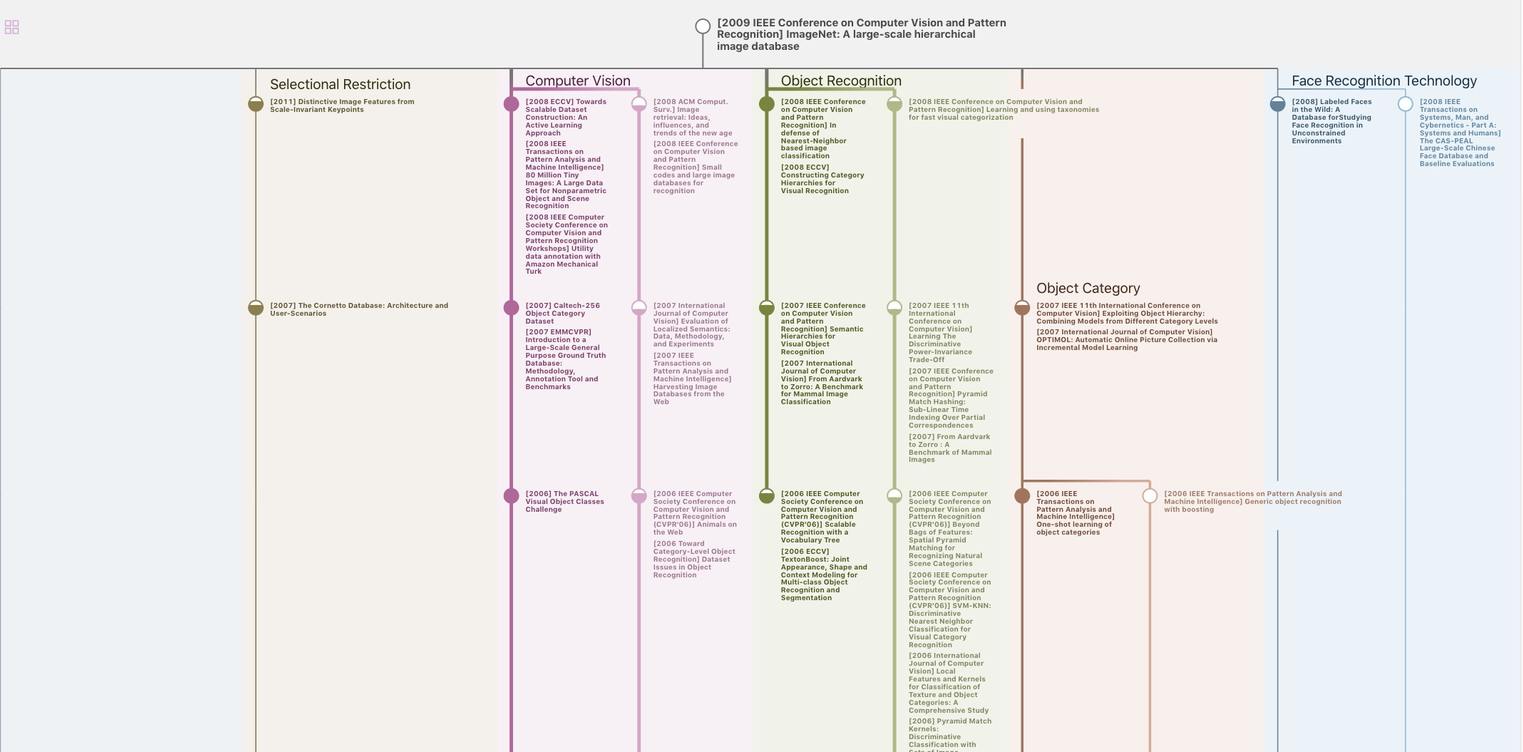
生成溯源树,研究论文发展脉络
Chat Paper
正在生成论文摘要