Nonparametric Mixture MLEs Under Gaussian-Smoothed Optimal Transport Distance
IEEE TRANSACTIONS ON INFORMATION THEORY(2023)
摘要
The Gaussian-smoothed optimal transport (GOT) framework, pioneered by Goldfeld et al. and followed up by a series of subsequent papers, has quickly caught attention among researchers in statistics, machine learning, information theory, and related fields. One key observation made therein is that, by adapting to the GOT framework instead of its unsmoothed counterpart, the curse of dimensionality for using the empirical measure to approximate the true data generating distribution can be lifted. The current paper shows that a related observation applies to the estimation of nonparametric mixing distributions in discrete exponential family models, where under the GOT cost the estimation accuracy of the nonparametric MLE can be accelerated to a polynomial rate. This is in sharp contrast to the classical sub-polynomial rates based on unsmoothed metrics, which cannot be improved from an information-theoretical perspective. A key step in our analysis is the establishment of a new Jackson-type approximation bound of Gaussian-smoothed Lipschitz functions. This insight bridges existing techniques of analyzing the nonparametric MLEs and the new GOT framework.
更多查看译文
关键词
Convergence,Mixture models,Q measurement,Density functional theory,Convolution,Upper bound,Smoothing methods,GOT distance,nonparametric mixture models,nonparametric maximum likelihood estimation,rate of convergence,function approximation
AI 理解论文
溯源树
样例
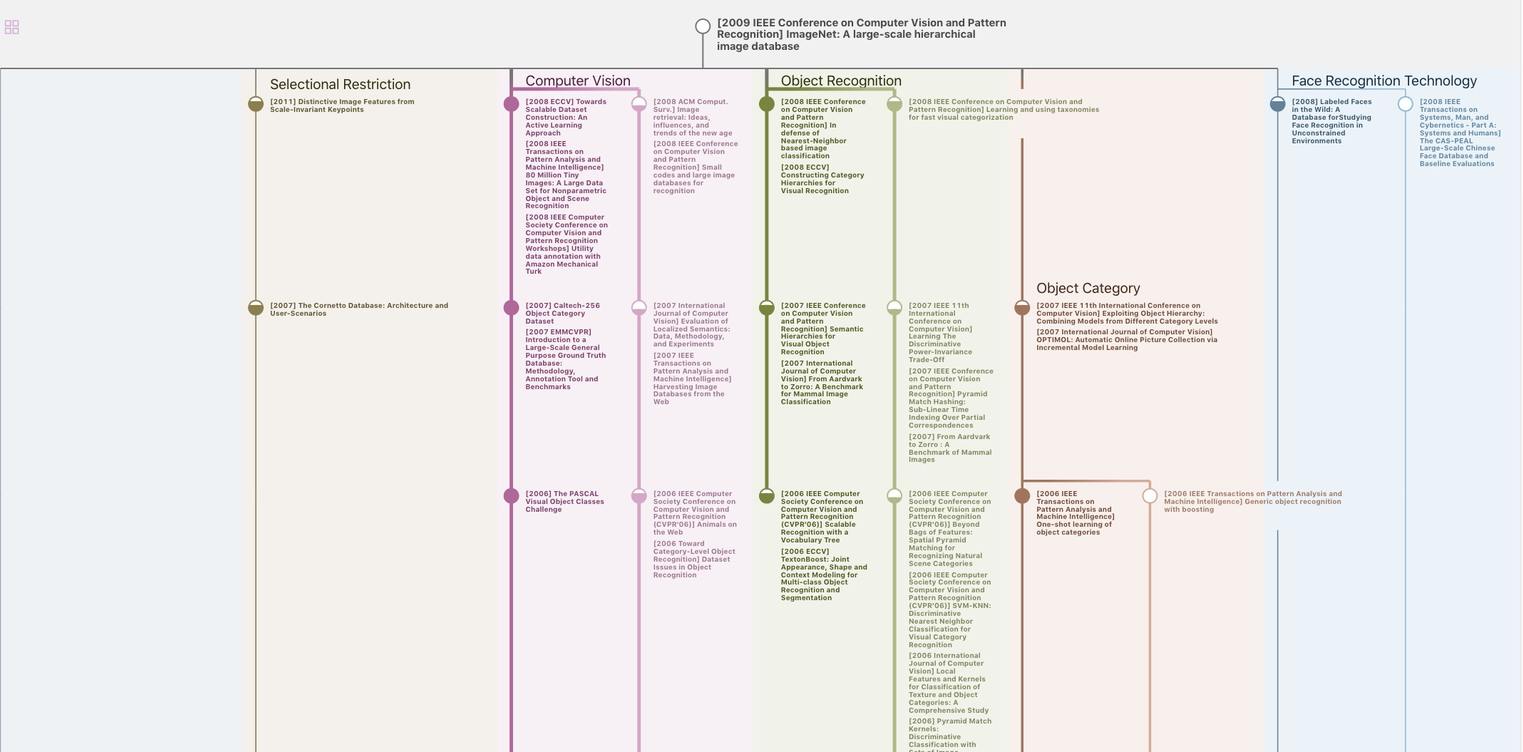
生成溯源树,研究论文发展脉络
Chat Paper
正在生成论文摘要