Fast topological clustering with Wasserstein distance
International Conference on Learning Representations (ICLR)(2022)
摘要
The topological patterns exhibited by many real-world networks motivate the development of topology-based methods for assessing the similarity of networks. However, extracting topological structure is difficult, especially for large and dense networks whose node degrees range over multiple orders of magnitude. In this paper, we propose a novel and computationally practical topological clustering method that clusters complex networks with intricate topology using principled theory from persistent homology and optimal transport. Such networks are aggregated into clusters through a centroid-based clustering strategy based on both their topological and geometric structure, preserving correspondence between nodes in different networks. The notions of topological proximity and centroid are characterized using a novel and efficient approach to computation of the Wasserstein distance and barycenter for persistence barcodes associated with connected components and cycles. The proposed method is demonstrated to be effective using both simulated networks and measured functional brain networks.
更多查看译文
关键词
Topological data analysis,cluster analysis,persistent homology,Wasserstein distance,Wasserstein barycenter,brain networks,intracranial electrophysiology,consciousness
AI 理解论文
溯源树
样例
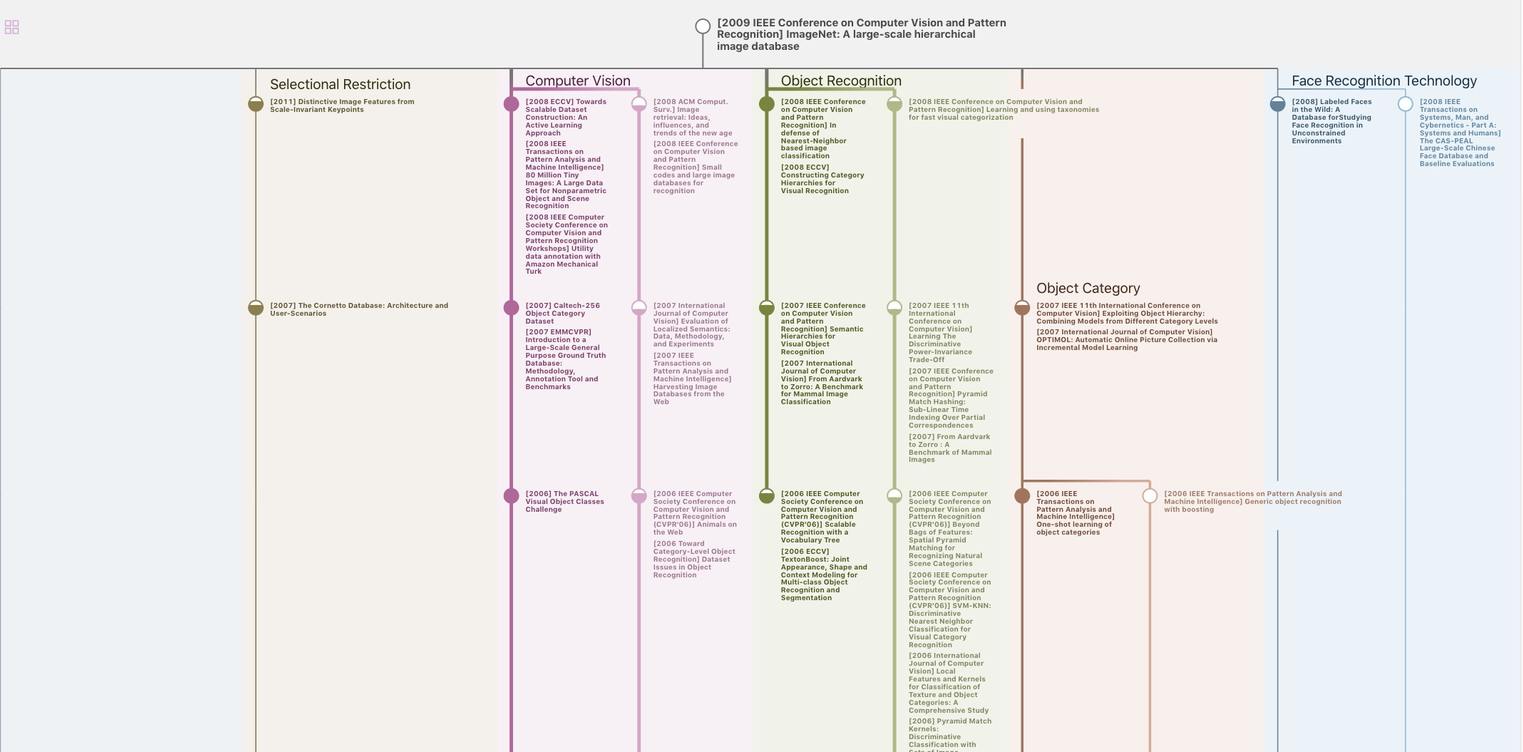
生成溯源树,研究论文发展脉络
Chat Paper
正在生成论文摘要