Reducing and linking spatio-temporal datasets with kD-STR
GIS(2020)
摘要
ABSTRACTWhen linking spatio-temporal datasets, the kD-STR algorithm can be used to reduce the datasets and speed up the linking process. However, kD-STR can sacrifice accuracy in the linked dataset whilst retaining unnecessary information. To overcome this, we propose a preprocessing step that removes unnecessary information and an alternative heuristic for kD-STR that prioritises accuracy in the linked output. These are evaluated in a case study linking a road condition dataset with air temperature, rainfall and road traffic data. In this case study, we found the alternative heuristic achieved a 19% improvement in mean error for the linked air temperature features and an 18% reduction in storage used for the rainfall dataset compared to the original kD-STR heuristic. The results in this paper support our hypothesis that, at worse, our alternative heuristic will yield a similar error and storage overhead for linking scenarios as the original kD-STR heuristic. However, in some cases it can give a reduction that is more accurate when linking the datasets whilst using less storage than the original kD-STR algorithm.
更多查看译文
AI 理解论文
溯源树
样例
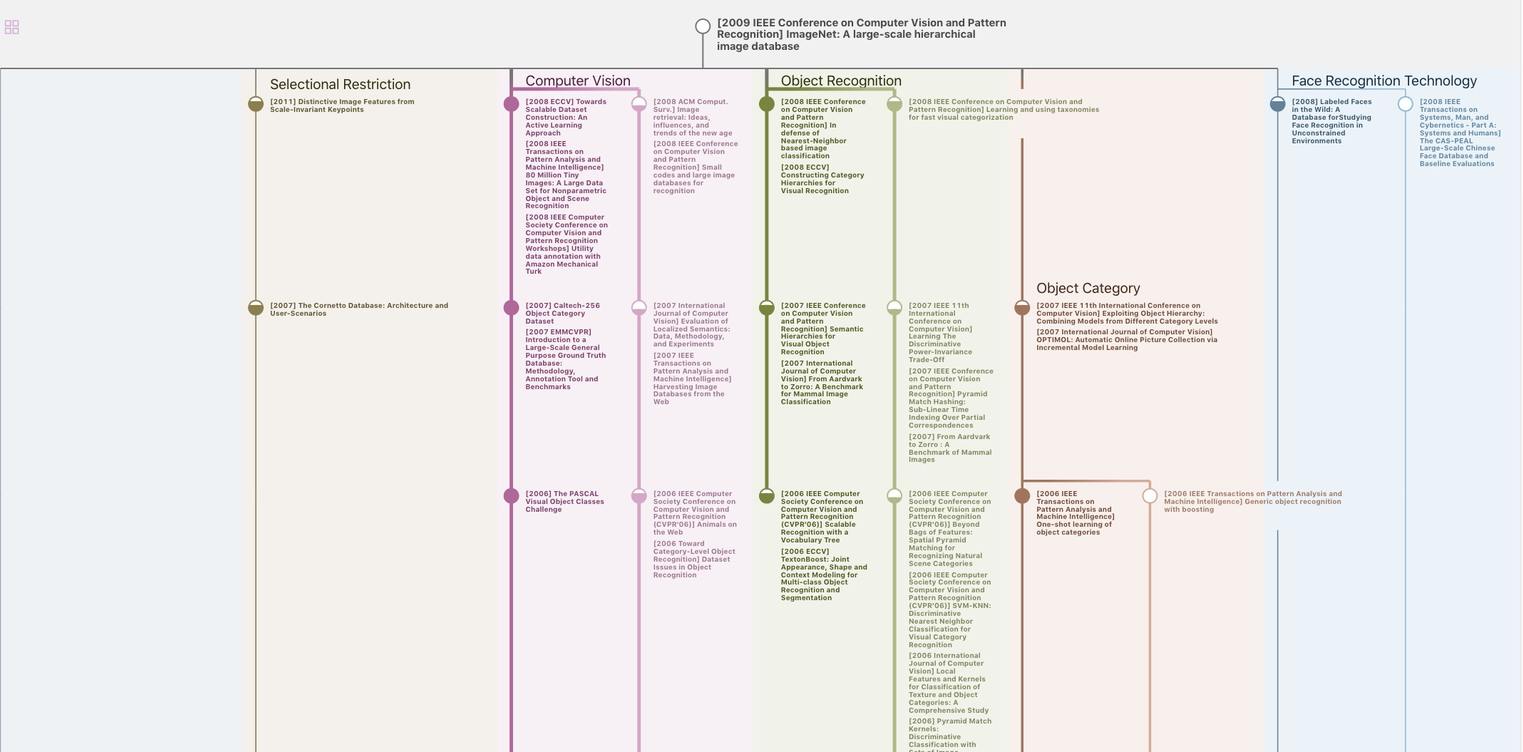
生成溯源树,研究论文发展脉络
Chat Paper
正在生成论文摘要