AdaViT: Adaptive Vision Transformers for Efficient Image Recognition.
IEEE Conference on Computer Vision and Pattern Recognition(2022)
摘要
Built on top of self-attention mechanisms, vision transformers have demonstrated remarkable performance on a variety of vision tasks recently. While achieving excellent performance, they still require relatively intensive computational cost that scales up drastically as the numbers of patches, self-attention heads and transformer blocks increase. In this paper, we argue that due to the large variations among images, their need for modeling long-range dependencies between patches differ. To this end, we introduce AdaViT, an adaptive computation framework that learns to derive usage policies on which patches, self-attention heads and transformer blocks to use throughout the backbone on a per-input basis, aiming to improve inference efficiency of vision transformers with a minimal drop of accuracy for image recognition. Optimized jointly with a transformer backbone in an end-to-end manner, a light-weight decision network is attached to the backbone to produce decisions on-the-fly. Extensive experiments on ImageNet demonstrate that our method obtains more than 2x improvement on efficiency compared to state-of-the-art vision transformers with only 0.8% drop of accuracy, achieving good efficiency/accuracy trade-offs conditioned on different computational budgets. We further conduct quantitative and qualitative analysis on learned usage polices and provide more insights on the redundancy in vision transformers.
更多查看译文
关键词
adaptive vision transformers,recognition,image
AI 理解论文
溯源树
样例
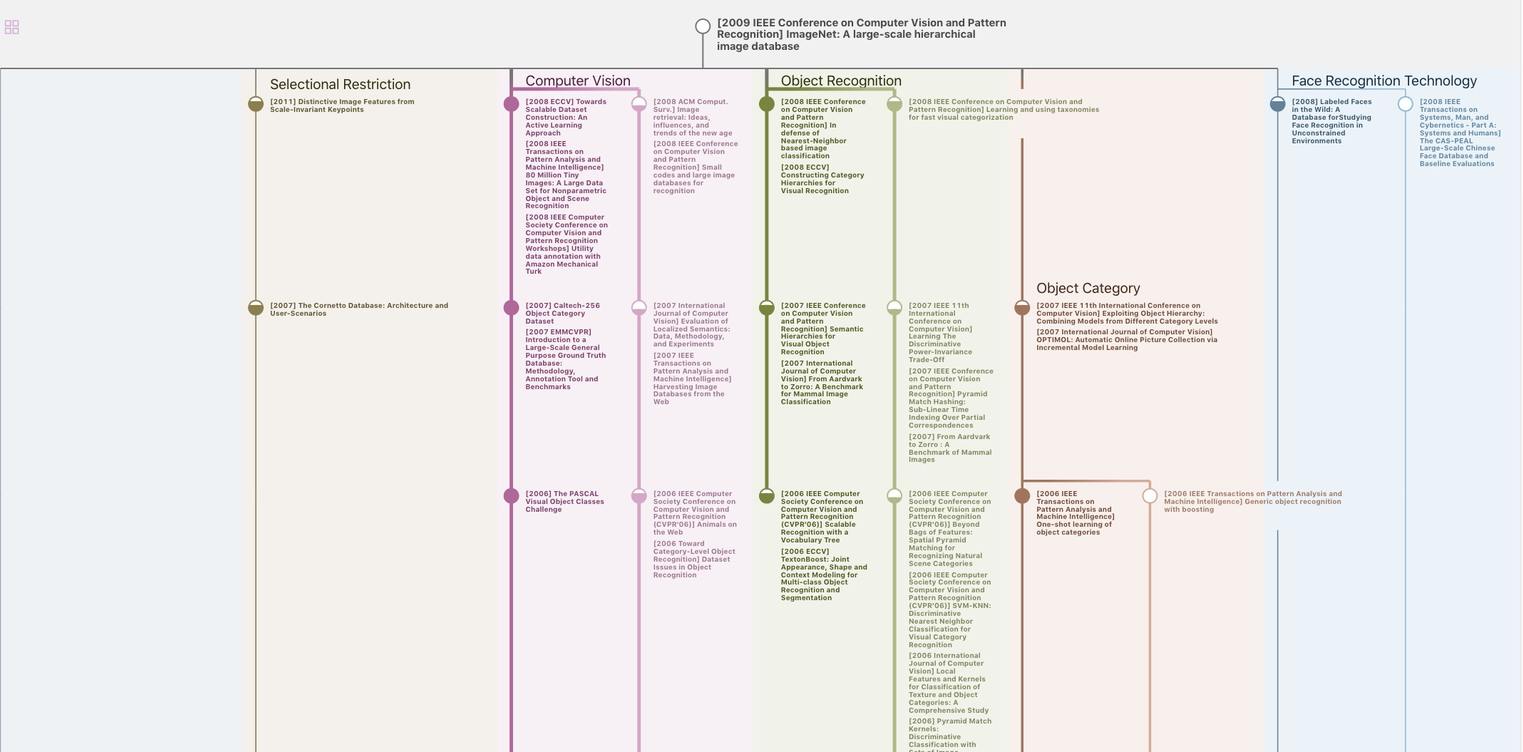
生成溯源树,研究论文发展脉络
Chat Paper
正在生成论文摘要