Native-resolution myocardial principal Eulerian strain mapping using convolutional neural networks and Tagged Magnetic Resonance Imaging
COMPUTERS IN BIOLOGY AND MEDICINE(2022)
摘要
Background: Assessment of regional myocardial function at native pixel-level resolution can play a crucial role in recognizing the early signs of the decline in regional myocardial function. Extensive data processing in existing techniques limits the effective resolution and accuracy of the generated strain maps. The purpose of this study is to compute myocardial principal strain maps ep1 and ep2 from tagged MRI (tMRI) at the native image resolution using deep-learning local patch convolutional neural network (CNN) models (DeepStrain).& nbsp;Methods: For network training, validation, and testing, realistic tMRI datasets were generated and consisted of 53,606 cine images simulating the heart, the liver, blood pool, and backgrounds, including ranges of shapes, positions, motion patterns, noise, and strain. In addition, 102 in-vivo image datasets from three healthy subjects, and three Pulmonary Arterial Hypertension patients, were acquired and used to assess the network's in-vivo performance.& nbsp;Four convolutional neural networks were trained for mapping input tagging patterns to corresponding ground truth principal strains using different cost functions. Strain maps using harmonic phase analysis (HARP) were obtained with various spectral filtering settings for comparison. CNN and HARP strain maps were compared at the pixel level versus the ground-truth and versus the least-loss in-vivo maps using Pearson correlation coefficients (R) and the median error and Inter-Quartile Range (IQR) histograms.& nbsp;Results: CNN-based local patch DeepStrain maps at a phantom resolution of 1.1mm x 1.1 mm and in-vivo resolution of 2.1mm x 1.6 mm were artifact-free with multiple fold improvement with ep1 ground-truth median error of 0.009(0.007) vs. 0.32(0.385) using HARP and ep2 ground-truth error of 0.016(0.021) vs. 0.181(0.08) using HARP. CNN-based strain maps showed substantially higher agreement with the ground-truth maps with correlation coefficients R > 0.91 for ep1 and ep2 compared to R < 0.21 and R < 0.82 for HARP-generated maps, respectively.& nbsp;Conclusion: CNN-generated Eulerian strain mapping permits artifact-free visualization of myocardial function at the native image resolution.
更多查看译文
关键词
Tagging MRI, Deep learning, Convolutional neural network, Myocardial strain mapping, DeepStrain, Harmonic phase
AI 理解论文
溯源树
样例
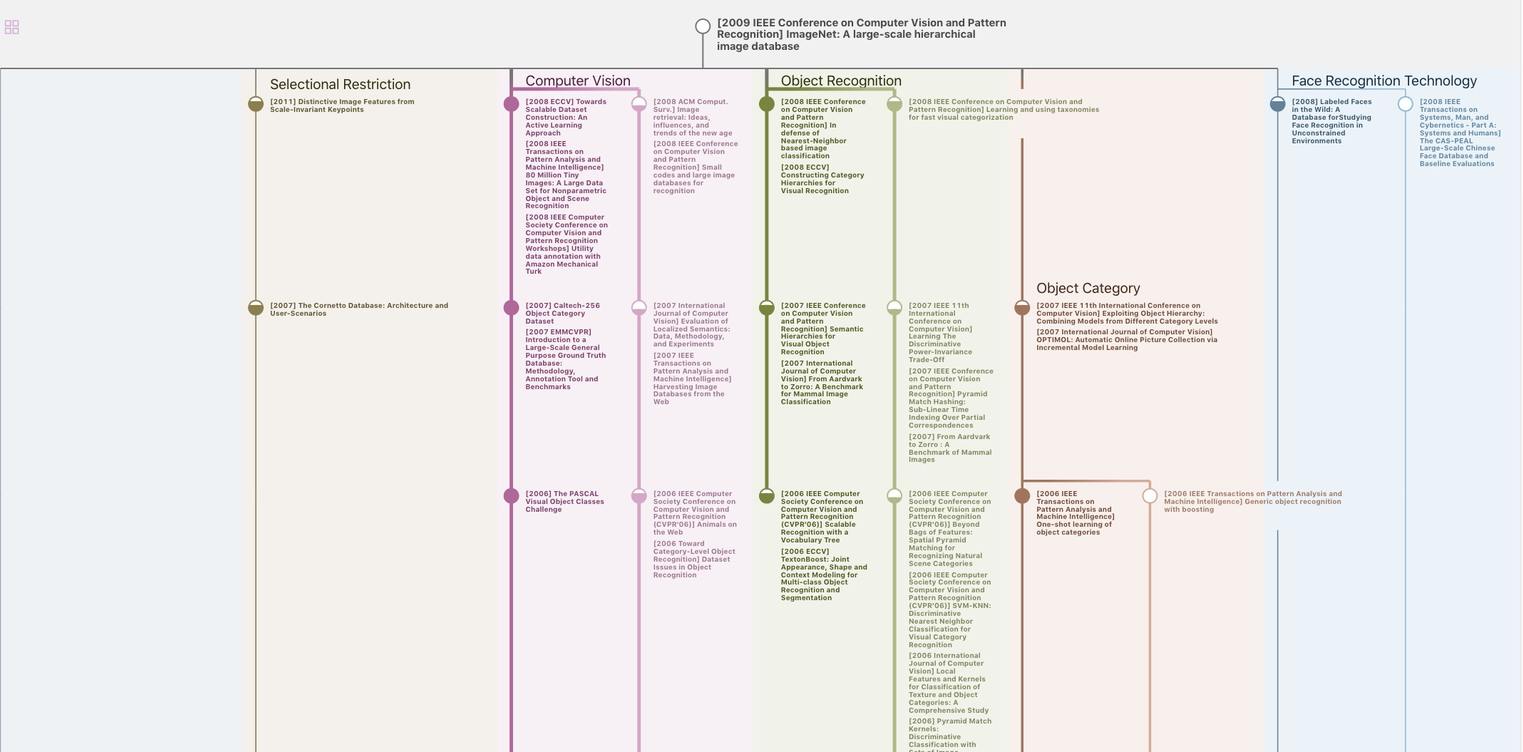
生成溯源树,研究论文发展脉络
Chat Paper
正在生成论文摘要