Predicting 10-2 Visual Field From Optical Coherence Tomography in Glaucoma Using Deep Learning Corrected With 24-2/30-2 Visual Field.
TRANSLATIONAL VISION SCIENCE & TECHNOLOGY(2021)
摘要
Purpose:To investigate whether a correction based on a Humphrey field analyzer (HFA) 24-2/30-2 visual field (VF) can improve the prediction performance of a deep learning model to predict the HFA 10-2 VF test from macular optical coherence tomography (OCT) measurements.
Methods:This is a multicenter, cross-sectional study. The training dataset comprised 493 eyes of 285 subjects (407, open-angle glaucoma [OAG]; 86, normative) who underwent HFA 10-2 testing and macular OCT. The independent testing dataset comprised 104 OAG eyes of 82 subjects who had undergone HFA 10-2 test, HFA 24-2/30-2 test, and macular OCT. A convolutional neural network (CNN) DL model was trained to predict threshold sensitivity (TH) values in HFA 10-2 from retinal thickness measured by macular OCT. The predicted TH values was modified by pattern-based regularization (PBR) and corrected with HFA 24-2/30-2. Absolute error (AE) of mean TH values and mean absolute error (MAE) of TH values were compared between the CNN-PBR alone model and the CNN-PBR corrected with HFA 24-2/30-2.
Results:AE of mean TH values was lower in the CNN-PBR with HFA 24-2/30-2 correction than in the CNN-PBR alone (1.9dB vs. 2.6dB; P = 0.006). MAE of TH values was lower in the CNN-PBR with correction compared to the CNN-PBR alone (4.2dB vs. 5.3 dB; P < 0.001). The inferior temporal quadrant showed lower prediction errors compared with other quadrants.
Conclusions:The performance of a DL model to predict 10-2 VF from macular OCT was improved by the correction with HFA 24-2/30-2.
Translational Relevance:This model can reduce the burden of additional HFA 10-2 by making the best use of routinely performed HFA 24-2/30-2 and macular OCT.
更多查看译文
关键词
deep learning, glaucoma, optical coherence tomography, visual field
AI 理解论文
溯源树
样例
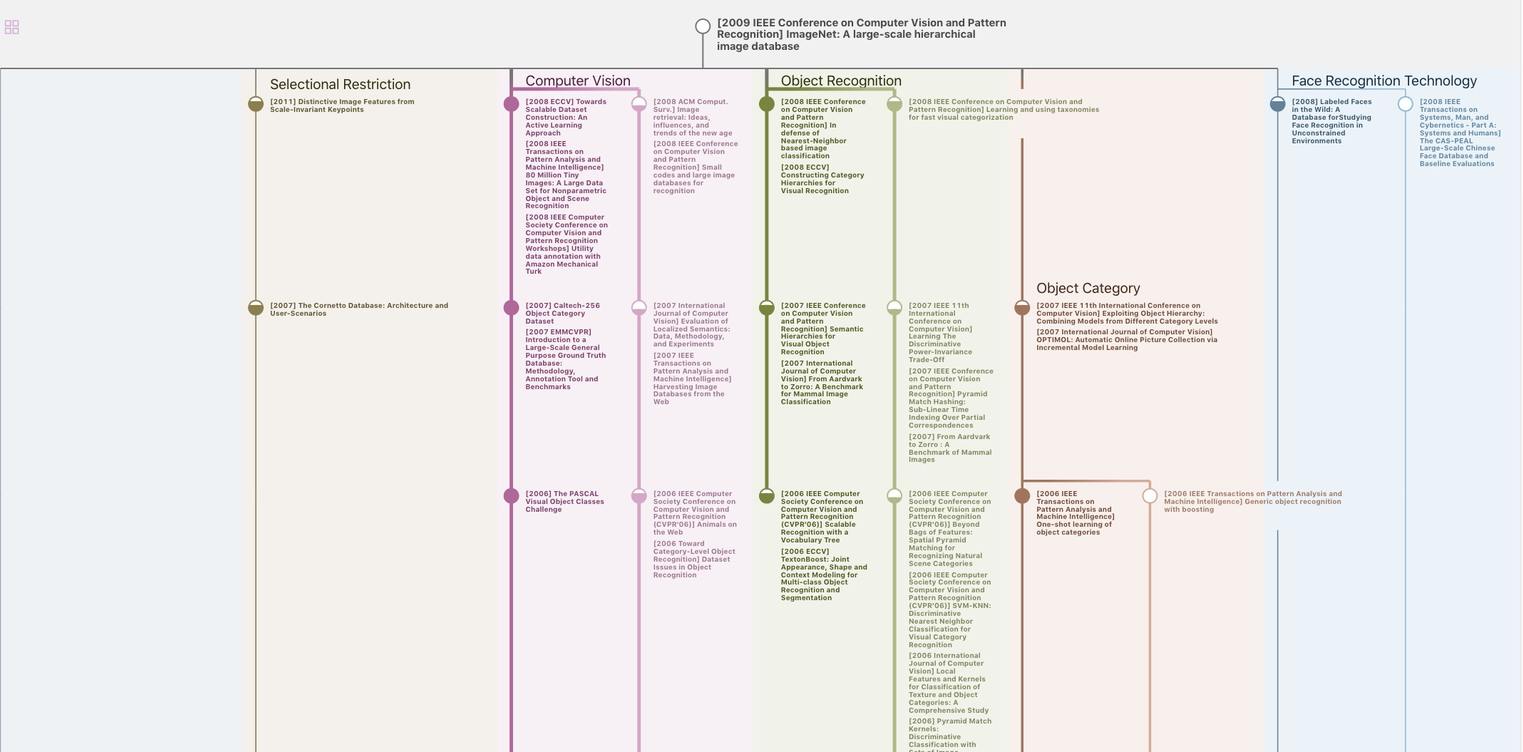
生成溯源树,研究论文发展脉络
Chat Paper
正在生成论文摘要