Gaussian-guided feature alignment for unsupervised cross-subject adaptation
Pattern Recognition(2022)
摘要
•Human activities recognition (HAR) and human intent recognition (HIR) are important for medical diagnosis and human-robot interaction. HAR and HIR usually rely on the signals of some wearable sensors, such as inertial measurement unit (IMU), but these signals may be user-dependent, which degrades the performance of the recognition algorithm on new subjects. Traditional supervised learning methods require labeling signals and training specific classifiers for each new subject, which is burdensome. To deal with this problem, this paper proposes a novel non-adversarial cross-subject adaptation method called Gaussian-guided feature alignment (GFA). The proposed GFA metric quantifies the discrepancy between the labeled features of source subjects and the unlabeled features of target subjects so that minimizing the GFA metric leads to the alignment of the source and target features. The GFA metric is estimated by calculating the divergence between the feature distribution and Gaussian distribution, as well as the mean squared error of the mean and variance between source and target features. This paper analytically proves the effect of the GFA metric and validates its performance using three public human activity datasets. Experimental results show that the proposed GFA achieves 1% higher target classification accuracy and 0.5% lower variance than state-of-the-art methods in case of cross-subject validation. These results indicate that the proposed GFA is feasible for improving the generalization of the HAR and HIR.•The key contributions of the present paper include: The cross-subject validation problems are resolved by proposing a novel Gaussian-guided Feature Alignment (GFA) metric to extract the features of the two domains in a Gaussian distribution space without projecting the features to another vector space, e.g., Hilbert space. A better alignment is achieved by adjusting the mean and variance of the Gaussian distribution based on the mean and variance of the corresponding feature distribution and sorting the data sampled from the feature and Gaussian distributions. The effect of the GFA metric on aligning the target distribution with the source distribution and decreasing the upper bound of the target error is analytically proved. Moreover, the feature extractor is optimized to decrease the variance of the target classification accuracy on the training set to further improve the performance of the cross-subject adaptation. The experimental results show that the proposed method outperforms state-of-the-art methods in the case of the cross-subject validation on one motion-sensor-based HIR dataset, one motionsensor- based HAR dataset, and one skeleton-based HAR dataset.
更多查看译文
关键词
Domain adaptation,Feature alignment,Human activity recognition,Human intent recognition,Sensor fusion
AI 理解论文
溯源树
样例
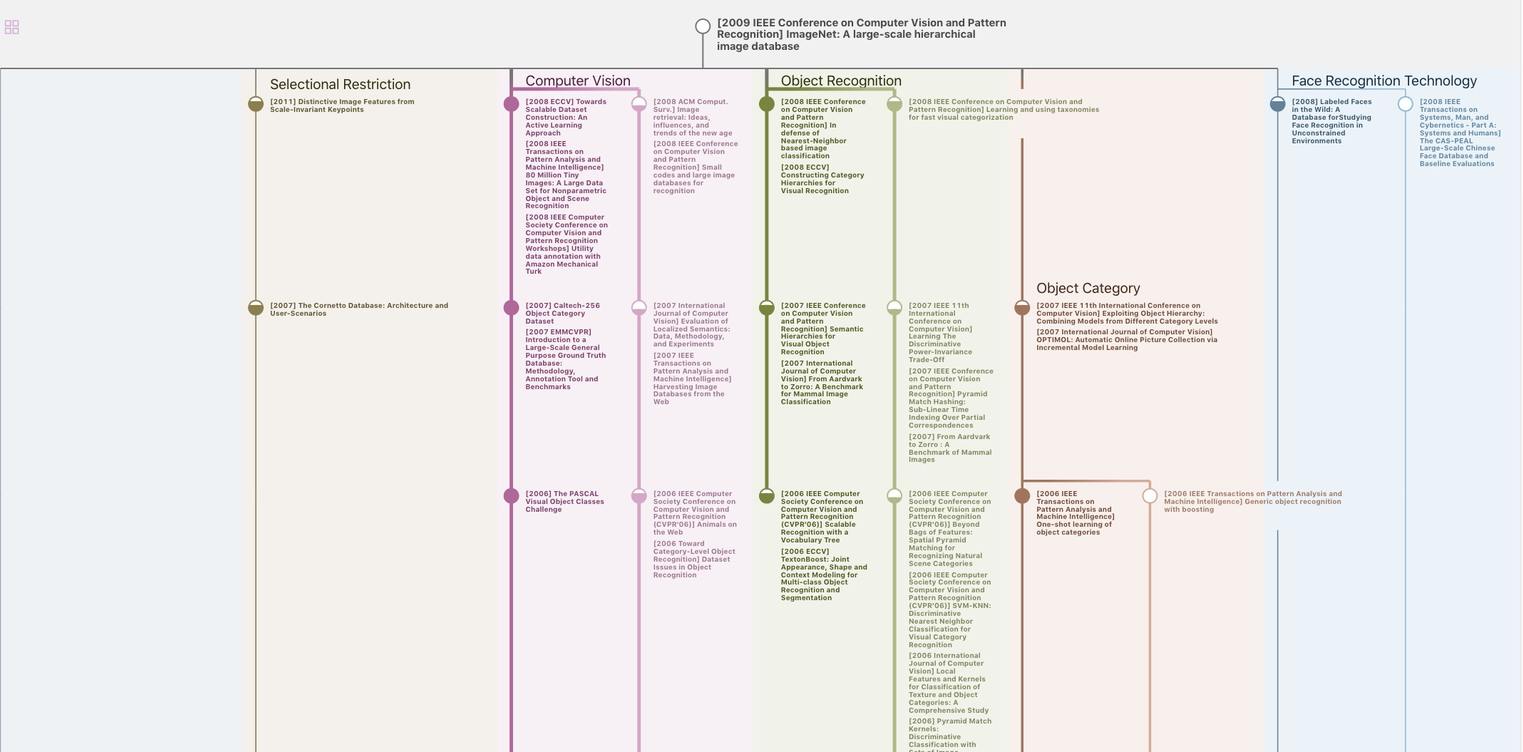
生成溯源树,研究论文发展脉络
Chat Paper
正在生成论文摘要