Sharpening Sparse Regularizers via Smoothing
IEEE Open Journal of Signal Processing(2021)
摘要
Non-convex sparsity-inducing penalties outperform their convex counterparts, but generally sacrifice the cost function convexity. As a middle ground, we propose the sharpening sparse regularizers (SSR) framework to design non-separable non-convex penalties that induce sparsity more effectively than convex penalties such as
l
1
and nuclear norms, but without sacrificing the cost function convexity. The overall problem convexity is preserved by exploiting the data fidelity relative strong convexity. The framework constructs penalties as the difference of convex functions, namely the difference between convex sparsity-inducing penalties and their smoothed versions. We propose a generalized infimal convolution smoothing technique to obtain the smoothed versions. Furthermore, SSR recovers and generalizes several non-convex penalties in the literature as special cases. The SSR framework is applicable to any sparsity regularized least squares ill-posed linear inverse problem. Beyond regularized least squares, the SSR framework can be extended to accommodate Bregman divergence, and other sparsity structures such as low-rankness. The SSR optimization problem can be formulated as a saddle point problem, and solved by a scalable forward-backward splitting algorithm. The effectiveness of the SSR framework is demonstrated by numerical experiments in different applications.
更多查看译文
关键词
Sparsity,low-rank,convex analysis,convex optimization,non-convexity,smoothing
AI 理解论文
溯源树
样例
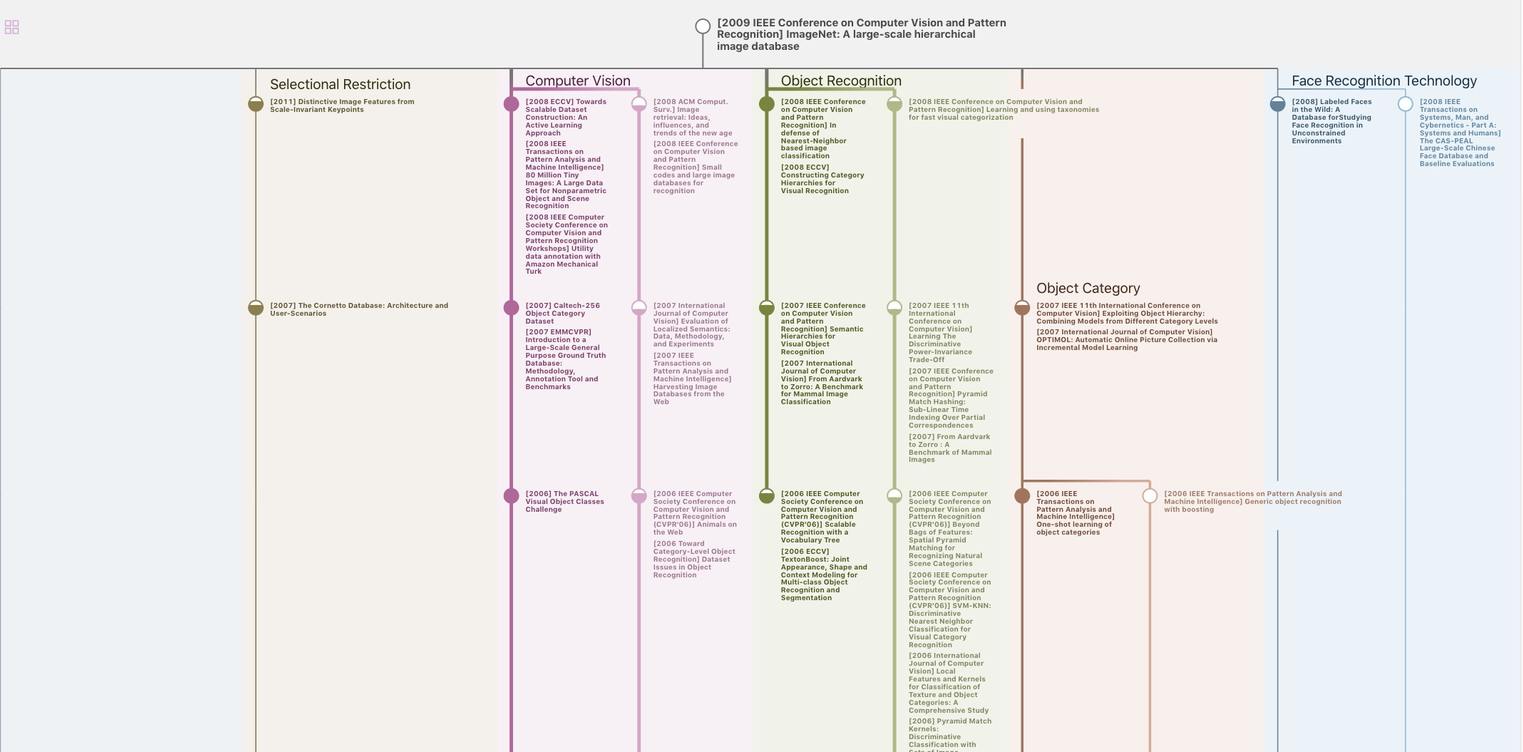
生成溯源树,研究论文发展脉络
Chat Paper
正在生成论文摘要