Investigation of Quantification of Agglomeration Level of Silica Particles in CMP Slurry for Creating the Quality Prediction Formula by AI Technology
2019 IEEE Albany Nanotechnology Symposium (ANS)(2019)
摘要
Artificial Intelligence techniques (AI) offer the possibility of stabilize process control independent of human skills for many industries, including Chemical Mechanical Planarization (CMP) in a silicon wafer manufacturing flow. In this arena, AI is mainly used to develop a formula for predicting the quality of wafers after CMP. In the current stage of AI development, explanatory variables are required to be quantifiable values, and still need to be chosen by a human. Generally, the explanatory variables used are the processing data which is easy to get from CMP equipment in a manufacturing process. However, these values are not directly measuring the conditions of the wafer or the consumables. In this study, we focus on providing more directly relevant data which could serve as inputs for AI algorithms based on the condition of the silica particles in the CMP slurry. In particular, we quantify the agglomeration levels of silica particles (AGL), and investigate the behaviours of AGL at several pressure levels. Further, we explain the relationships between AGL and polishing abilities, as a more relevant input for AI prediction of wafer quality.
更多查看译文
关键词
silica particles,CMP,silicon wafer
AI 理解论文
溯源树
样例
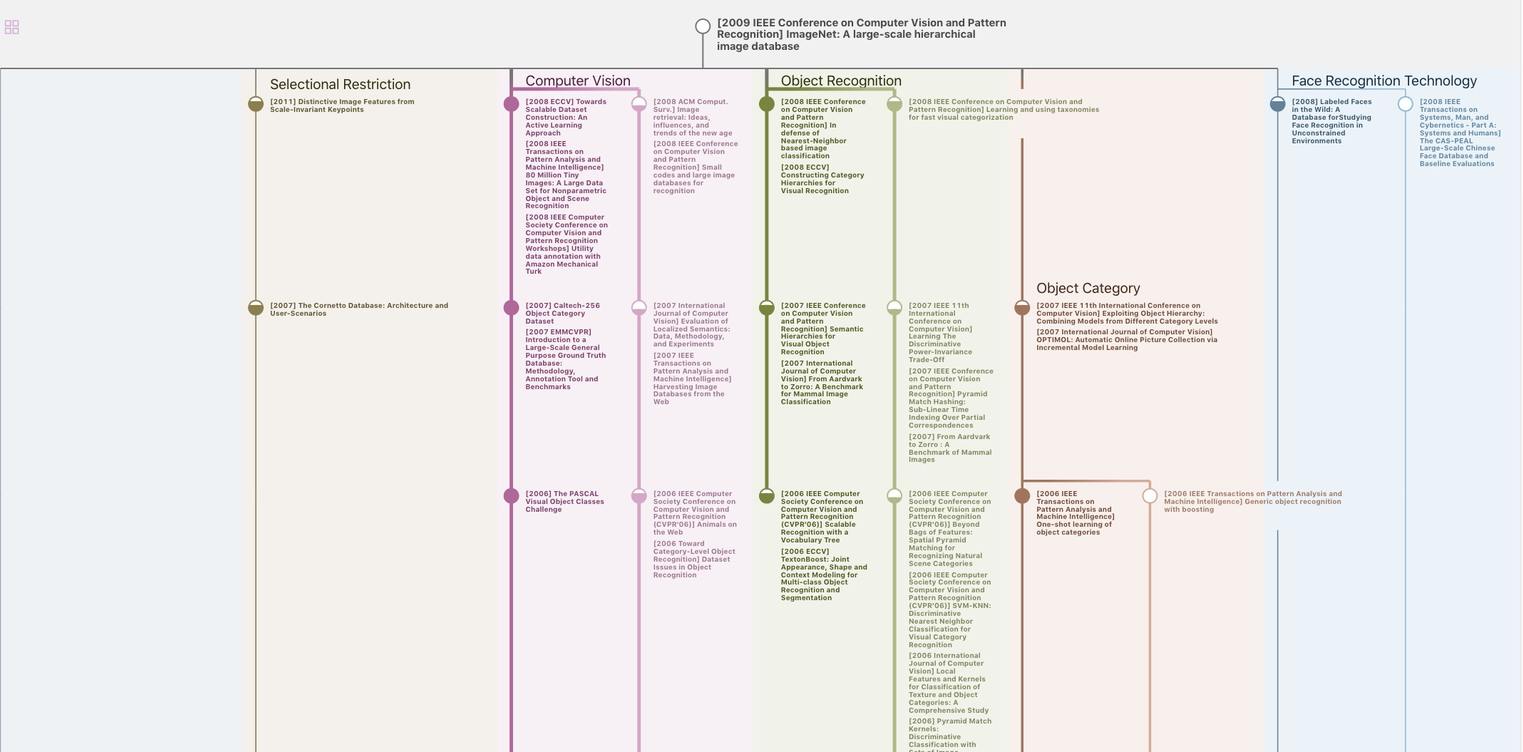
生成溯源树,研究论文发展脉络
Chat Paper
正在生成论文摘要