Explaining Deep Neural Network using Layer-wise Relevance Propagation and Integrated Gradients
2021 IEEE 19th World Symposium on Applied Machine Intelligence and Informatics (SAMI)(2021)
摘要
Machine learning has become an integral part of technology in today's world. The field of artificial intelligence is the subject of research by a wide scientific community. In particular, through improved methodology, the availability of big data, and increased computing power, today's machine learning algorithms can achieve excellent performance that sometimes even exceeds the human level. However, due to their nested nonlinear structure, these models are generally considered to be “Black boxes” that do not provide any information about what exactly leads them to provide a specific output. This raised the need to interpret these algorithms and understand how they work as they are applied even in areas where they can cause critical damage. This article describes Integrated Gradients [1] and Layer-wise Relevance Propagation [2] methods and presents individual experiments with. In experiments we have used well-known datasets like MNIST[3], MNIST-Fashion dataset[4], Imagenette and Imagewoof which are subsets of ImageNet [5].
更多查看译文
关键词
deep neural network,layer-wise relevance propagation,integrated gradients,artificial intelligence,Big Data,machine learning algorithms,human level,nested nonlinear structure,black boxes,MNIST-Fashion dataset,Imagenette,Imagewoof
AI 理解论文
溯源树
样例
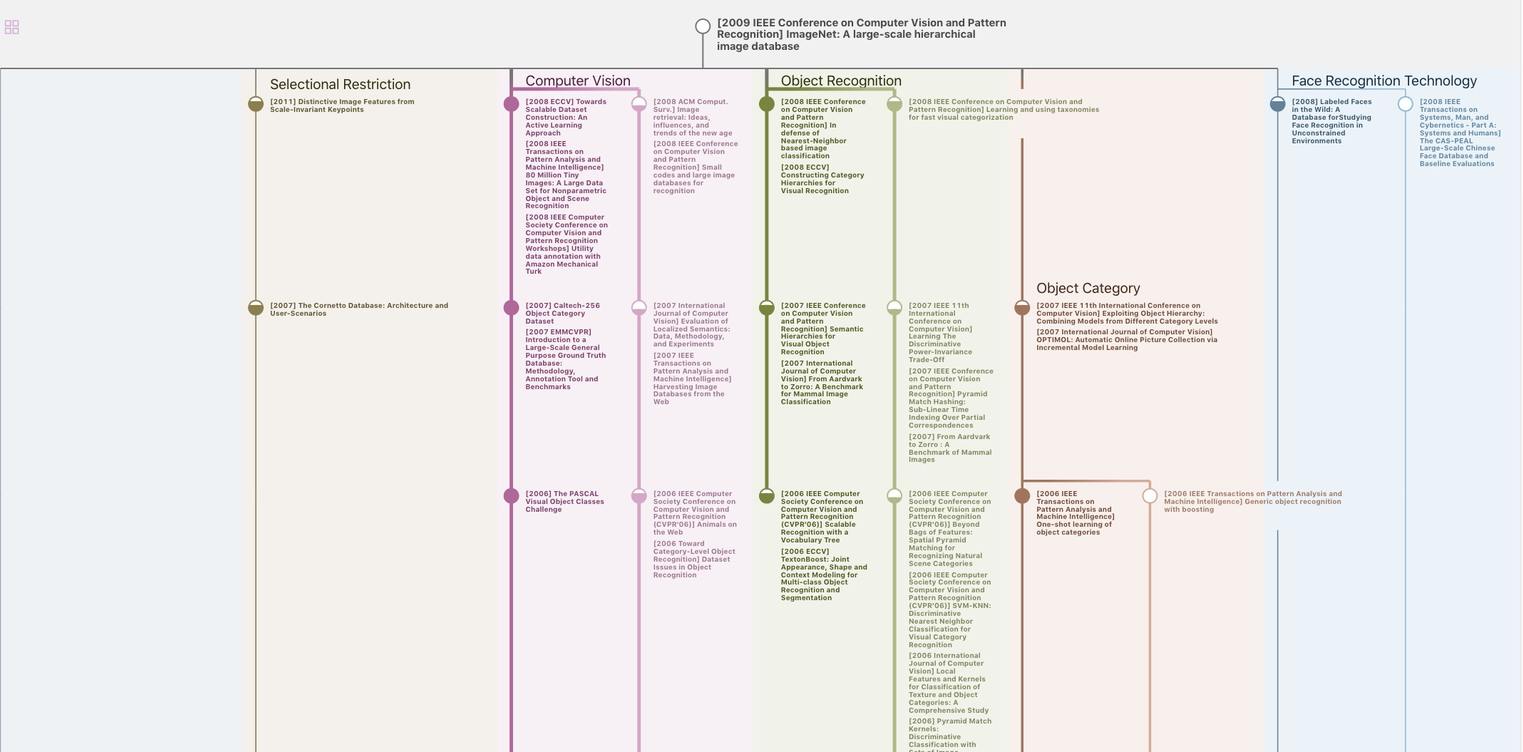
生成溯源树,研究论文发展脉络
Chat Paper
正在生成论文摘要