Machine Learning Investigation Of Supplementary Adsorbate Influence On Copper For Enhanced Electrochemical Co2 Reduction Performance
JOURNAL OF PHYSICAL CHEMISTRY C(2021)
摘要
The electrochemical reduction of CO2 presents a green alternative to the production of hydrocarbons, while mitigating atmospheric CO2; however, the scale-up of this operation is currently limited due to the lack of a high-performing catalyst. While copper has been identified as the only monometal catalyst to exhibit appreciable performance for the production of value-added products, opportunities exist to further enhance its catalytic activity and selectivity. One such strategy is the addition of supplementary adsorbates to the copper surface, which has been found to greatly influence its CO2RR activity and selectivity. However, the mechanism behind this phenomenon remains unclear and its beneficial role is scarcely explored. Herein, we present an accurate and high-throughput screening of 289 adsorbate-pair combinations and their influence on the rate-determining steps for methane and C2+ production on copper using machine learning in conjunction with density functional theory calculations. By implementing a rigorous feature selection process, we successfully reduced the dimensionality of our feature space from 52 to less than 10, resulting in decreased prediction errors and computation time. Using the extreme gradient boosting regressor, we identified 81 and 152 unique supplementary adsorbate combinations to promote methane and C2+ production compared to pristine copper, respectively, while 80 unique combinations are shown to simultaneously improve both methane and C2+ production. Within a model accuracy of 0.082 eV, the improvements in thermodynamics for methane and C2+ production are up to 0.339 and 0.397 eV, respectively. The results of our investigation not only provide new insights into the role of supplementary adsorbates to guide future experimental and theoretical studies but also demonstrate the efficacy of using machine learning as an efficient and accurate screening tool for large combinatory systems in energy-related applications.
更多查看译文
AI 理解论文
溯源树
样例
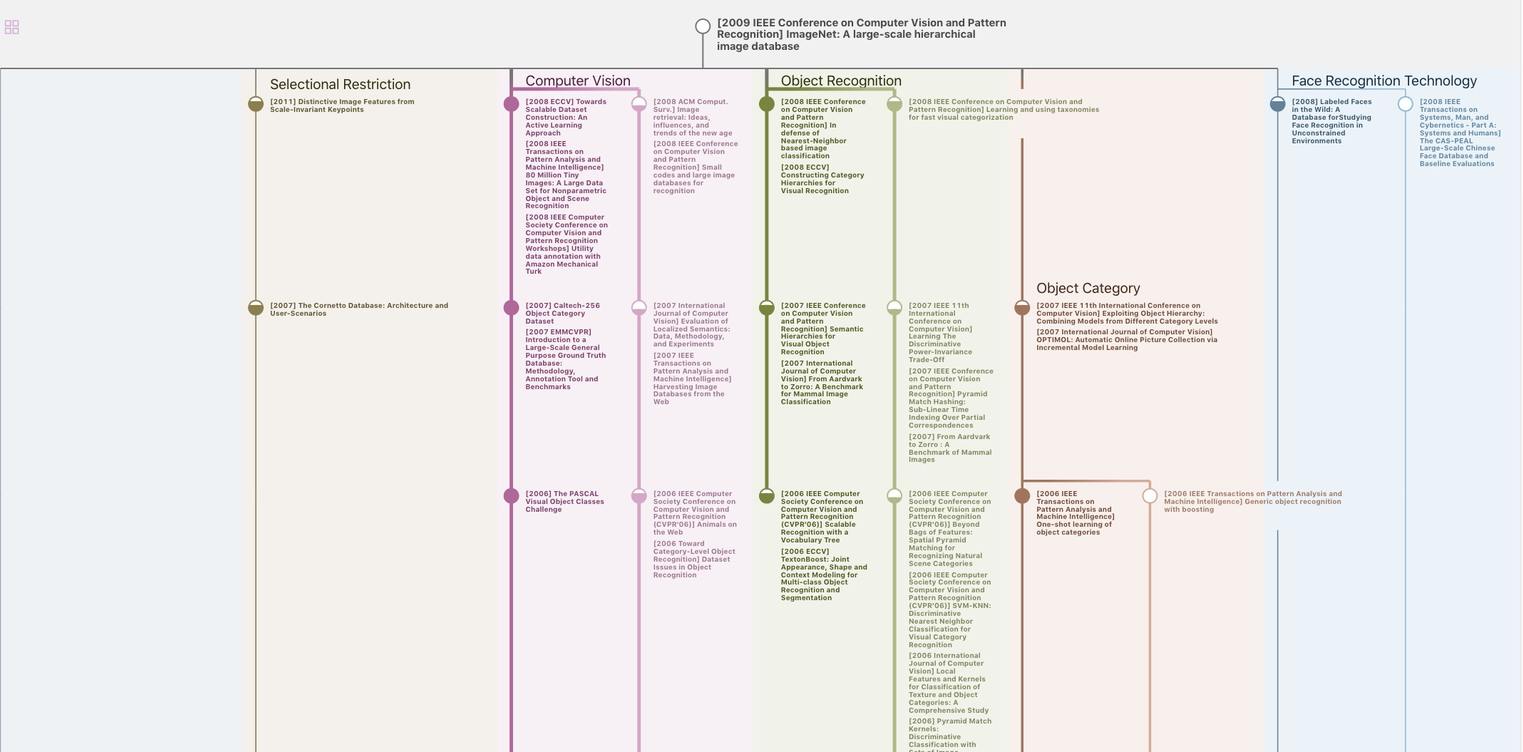
生成溯源树,研究论文发展脉络
Chat Paper
正在生成论文摘要