An Experimental Comparison Of Metaheuristic Frameworks For Multi-Objective Optimization
EXPERT SYSTEMS(2021)
摘要
Multi-objective optimization problems frequently appear in many diverse research areas and application domains. Metaheuristics, as efficient techniques to solve them, need to be easily accessible to users with different expertise and programming skills. In this context, metaheuristic optimization frameworks are helpful, as they provide popular algorithms, customizable components and additional facilities to conduct experiments. Due to the broad range of available tools, this paper presents a systematic evaluation and experimental comparison of 10 frameworks, covering from multi-purpose, consolidated tools to recent libraries specifically designed for multi-objective optimization. The evaluation is organized around seven characteristics: search components and techniques, configuration, execution, utilities, external support and community, software implementation and performance. An analysis of code metrics and a series of experiments serves to assess the last two features. Lesson learned and open issues are also discussed as part of the comparative study. The outcomes of the evaluation process reveal a contrasted support to recent advances in multi-objective optimization, with a lack of novel algorithms and variety of metaheuristics other than evolutionary algorithms. The experimental comparison also reports significant differences in terms of both execution time and memory usage under demanding configurations.
更多查看译文
关键词
evolutionary algorithms, metaheuristic optimization framework, metaheuristics, multi-objective optimization, swarm intelligence
AI 理解论文
溯源树
样例
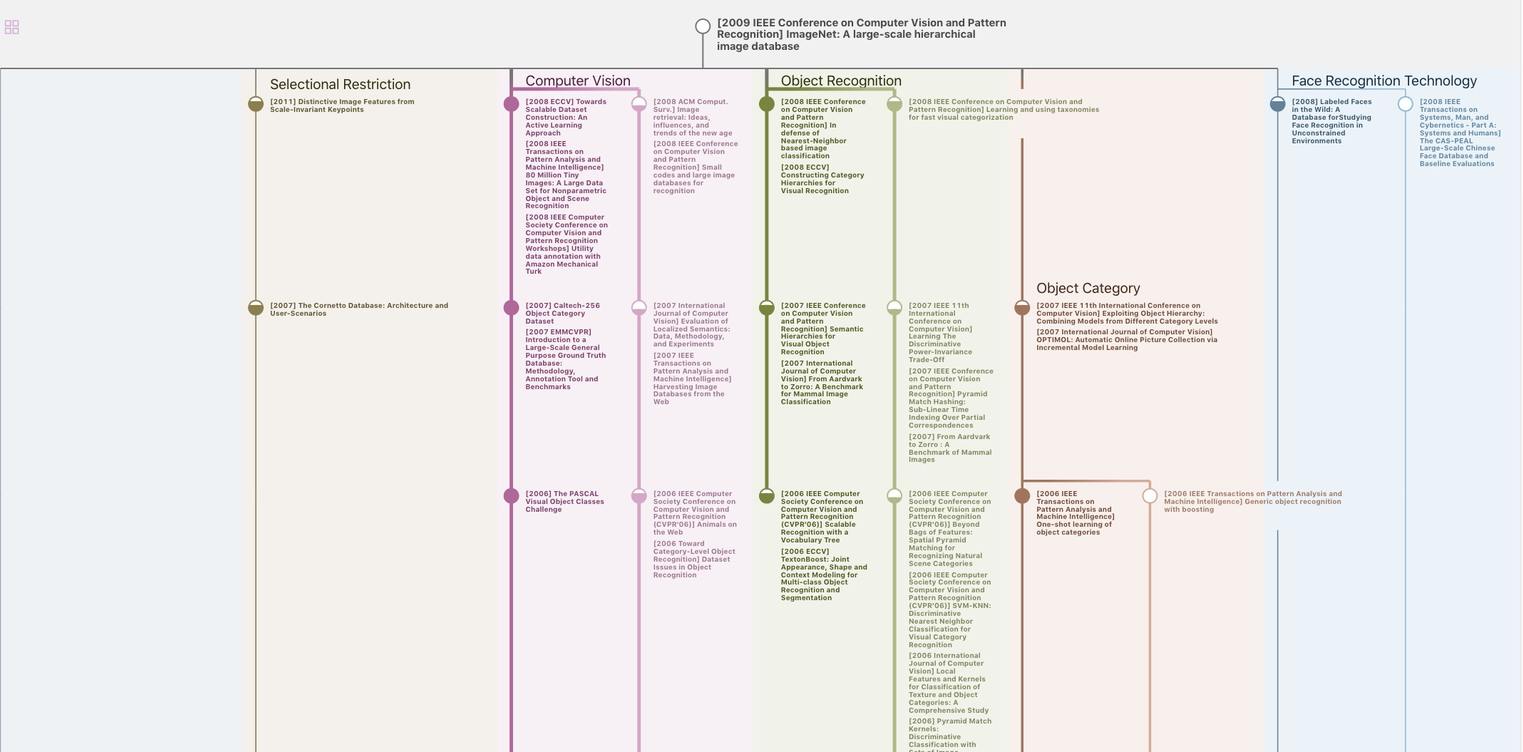
生成溯源树,研究论文发展脉络
Chat Paper
正在生成论文摘要