The Designed Bootstrap for Causal Inference in Big Observational Data
JOURNAL OF STATISTICAL THEORY AND PRACTICE(2021)
摘要
The combination of modern machine learning algorithms with the nonparametric bootstrap can enable effective predictions and inferences on Big Observational Data. An increasingly prominent and critical objective in such analyses is to draw causal inferences from the Big Observational Data. A fundamental step in addressing this objective is to design the Big Observational Data prior to the application of machine learning algorithms. The design step directly helps to reduce biases in the causal inferences that arise due to the non-randomized treatment assignment. In particular, performing the design step prior to implementing a machine learning algorithm ensures that subjects in different treatment groups with comparable covariates are subclassified or matched together, which reduces biases due to the confounding of covariates with treatment. However, the application of the traditional nonparametric bootstrap on Big Observational Data requires excessive computational efforts. This is because every bootstrap sample would need to be re-designed under the traditional approach, which can be prohibitive in practice. We propose a design-based bootstrap for deriving causal inferences with reduced bias from the application of machine learning algorithms on Big Observational Data. Our bootstrap procedure operates by resampling from the original designed observational data. It eliminates the need for additional, costly design steps on each bootstrap sample that are performed under the standard nonparametric bootstrap. We demonstrate the computational efficiency of this procedure compared to the traditional nonparametric bootstrap, and its equivalency in terms of confidence interval coverage rates for the average treatment effects, by means of simulation studies and a real-life case study. Ultimately, our procedure enables researchers to effectively use straightforward design procedures to obtain valid causal inferences with reduced computational efforts from the application of machine learning algorithms on Big Observational Data.
更多查看译文
关键词
Causal inference, Design of observational study, Matching, Bootstrap, Machine learning
AI 理解论文
溯源树
样例
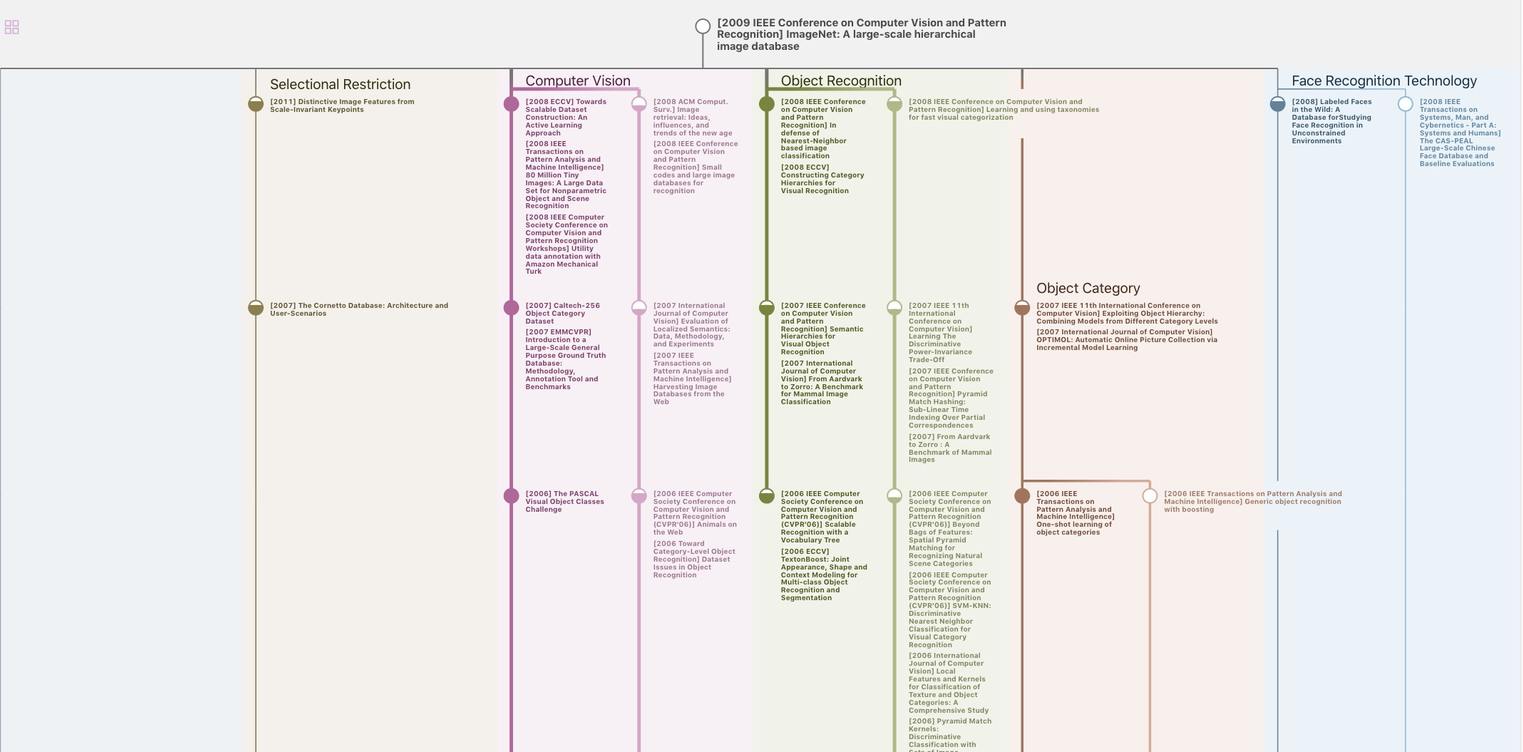
生成溯源树,研究论文发展脉络
Chat Paper
正在生成论文摘要