A Deep Learning Approach To Rapid Regional Post-Event Seismic Damage Assessment Using Time-Frequency Distributions Of Ground Motions
EARTHQUAKE ENGINEERING & STRUCTURAL DYNAMICS(2021)
摘要
Every year, earthquakes result in severe economic losses and a significant number of casualties worldwide. In limiting the losses that occur after these extreme events, timely and accurate assessment of seismic damages and mobilizing proportionate post-event relief efforts play crucial roles. Traditional on-site investigation generally results in prolonged evaluation windows. Several computational alternatives exist that show promise in addressing the downsides of the traditional approach. Damage estimates based on pre-computed fragility libraries can provide near-real time seismic damage quantification, but at present, they are coarse and involve considerable uncertainties. Estimates based on nonlinear time-history analyses simulate the seismic response in greater detail, yet due to the computation and data requirements, their use at the regional scale is challenging. Given this perspective, herein, a rapid regional post-event seismic damage assessment procedure based on convolutional neural network (CNN) is proposed. In this approach, an inventory of buildings, anticipated ground motion datasets, and corresponding damage levels for a region are brought together into a scenario bank. The time-frequency distribution graphs of the ground motions, which serve as detailed visual representations of their frequency-domain as well as time-domain features, are generated. These data are then used to train CNN models, which could predict the damage states. The proposed methodology is verified through two numerical studies-one for an individual building, and the other, regional case, involving the buildings in the Tsinghua University campus. The results confirm that the proposed method offers prediction results with sufficient accuracy in near real-time.
更多查看译文
关键词
convolutional neural network (CNN), real‐, time, seismic damage prediction, time‐, frequency distribution (TFD)
AI 理解论文
溯源树
样例
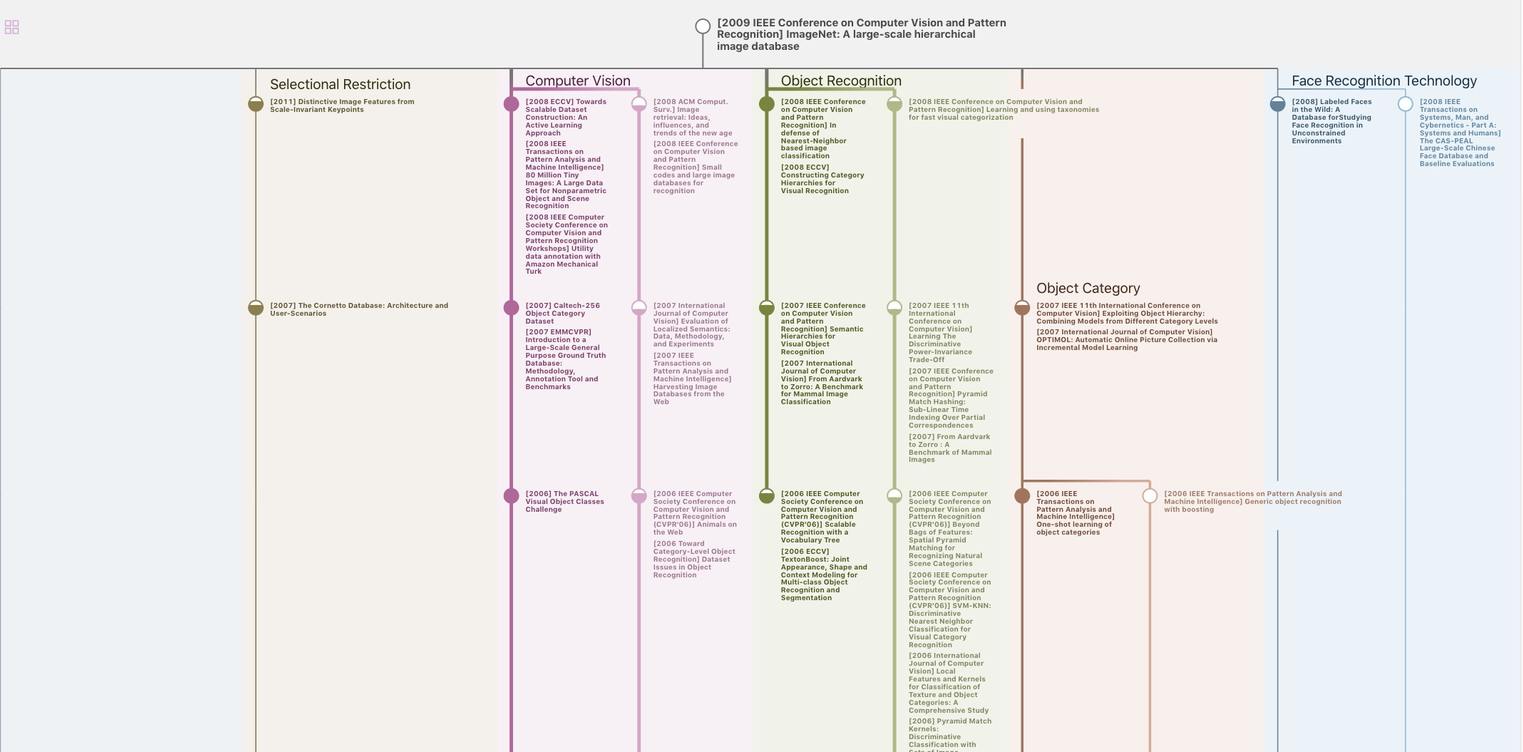
生成溯源树,研究论文发展脉络
Chat Paper
正在生成论文摘要