Rule-Based Eeg Classifier Utilizing Local Entropy Of Time-Frequency Distributions
MATHEMATICS(2021)
摘要
Electroencephalogram (EEG) signals are known to contain signatures of stimuli that induce brain activities. However, detecting these signatures to classify captured EEG waveforms is one of the most challenging tasks of EEG analysis. This paper proposes a novel time-frequency-based method for EEG analysis and characterization implemented in a computer-aided decision-support system that can be used to assist medical experts in interpreting EEG patterns. The computerized method utilizes EEG spectral non-stationarity, which is clearly revealed in the time-frequency distributions (TFDs) of multicomponent signals. The proposed algorithm, which is based on the modification of the Renyi entropy, called local or short-term Renyi entropy (STRE), was upgraded with a blind component separation procedure and instantaneous frequency (IF) estimation. The method was applied to EEGs of both forward and backward movements of the left and right hands, as well as to EEGs of imagined hand movements, which were captured by a 19-channel EEG recording system. The obtained results show that in a given virtual instrument, the proposed methods efficiently distinguish between real and imagined limb movements by considering their signatures in terms of the dominant EEG component's IFs at the specified subset of EEG channels (namely, F3, F4, F7, F8, T3, and T4). Furthermore, computing the number of EEG signal components, their extraction, and IF estimation provide important information that shows potential to enhance existing clinical diagnostic techniques for detecting the intensity, location, and type of brain function abnormalities in patients with neurological motor control disorders.
更多查看译文
关键词
Ré, nyi entropy, short-term Ré, nyi entropy, instantaneous frequency (IF) estimation, EEG signals, time–, frequency signal analysis
AI 理解论文
溯源树
样例
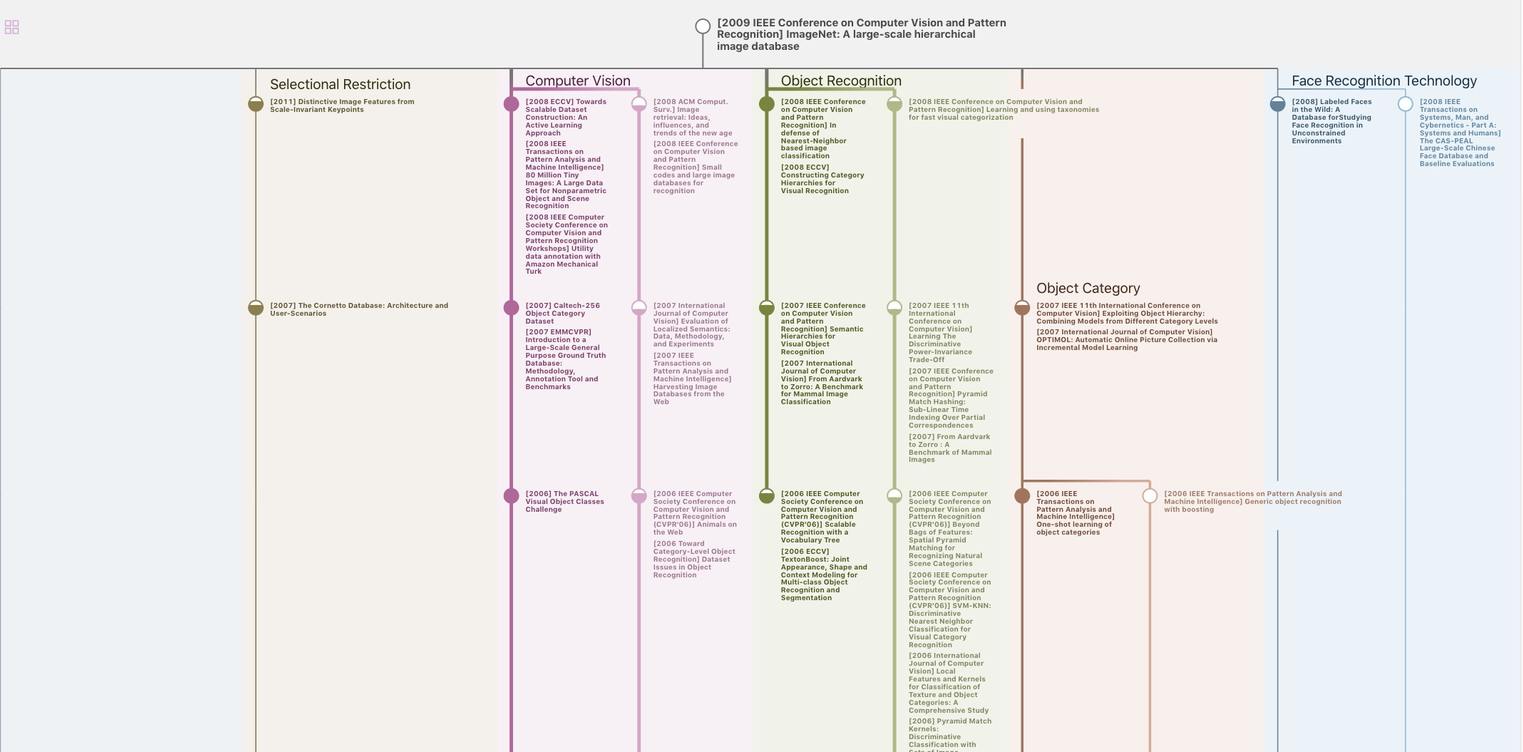
生成溯源树,研究论文发展脉络
Chat Paper
正在生成论文摘要