Probing Spatial Clues: Canonical Spatial Templates for Object Relationship Understanding
IEEE ACCESS(2021)
摘要
Humans often leverage spatial clues to categorize scenes in a fraction of a second. This form of intelligence is very relevant in time-critical situations (e.g., when driving a car) and valuable to transfer to automated systems. This work investigates the predictive power of solely processing spatial clues for scene understanding in 2D images and compares such an approach with the predictive power of visual appearance. To this end, we design the
laboratory
task of predicting the identity of two objects (e.g., “man” and “horse”) and their relationship or predicate (e.g., “riding”) given
exclusively
the ground truth bounding box coordinates of both objects. We also measure the performance attainable in Human Object Interaction (HOI) detection, a
real-world
spatial task, which includes a setting where ground truth boxes are not available at test time. An additional goal is to identify the principles necessary to effectively represent a spatial template, that is, the visual region in which two objects involved in a relationship expressed by a predicate occur. We propose a scale-, mirror-, and translation-invariant representation that captures the spatial essence of the relationship, i.e., a
canonical
spatial representation. Tests in two benchmarks reveal: (1) High performance is attainable by using exclusively spatial information in all tasks. (2) In HOI detection, the
canonical
template outperforms the rest of spatial, visual, and several state-of-the-art baselines. (3) Simple fusion of visual and spatial features substantially improves performance. (4) Our methods fare remarkably well with a small amount of data and rare categories. Our results obtained on the Visual Genome (VG) and the Humans Interacting with Common Objects - Detection (HICO-DET) datasets indicate that great predictive power can be obtained from spatial clues alone, opening up possibilities for performing fast scene understanding at a glance.
更多查看译文
关键词
Layout, Task analysis, Visualization, Semantics, Mirrors, Crops, Training, Spatial understanding, spatial layout, computer vision, vision and scene understanding
AI 理解论文
溯源树
样例
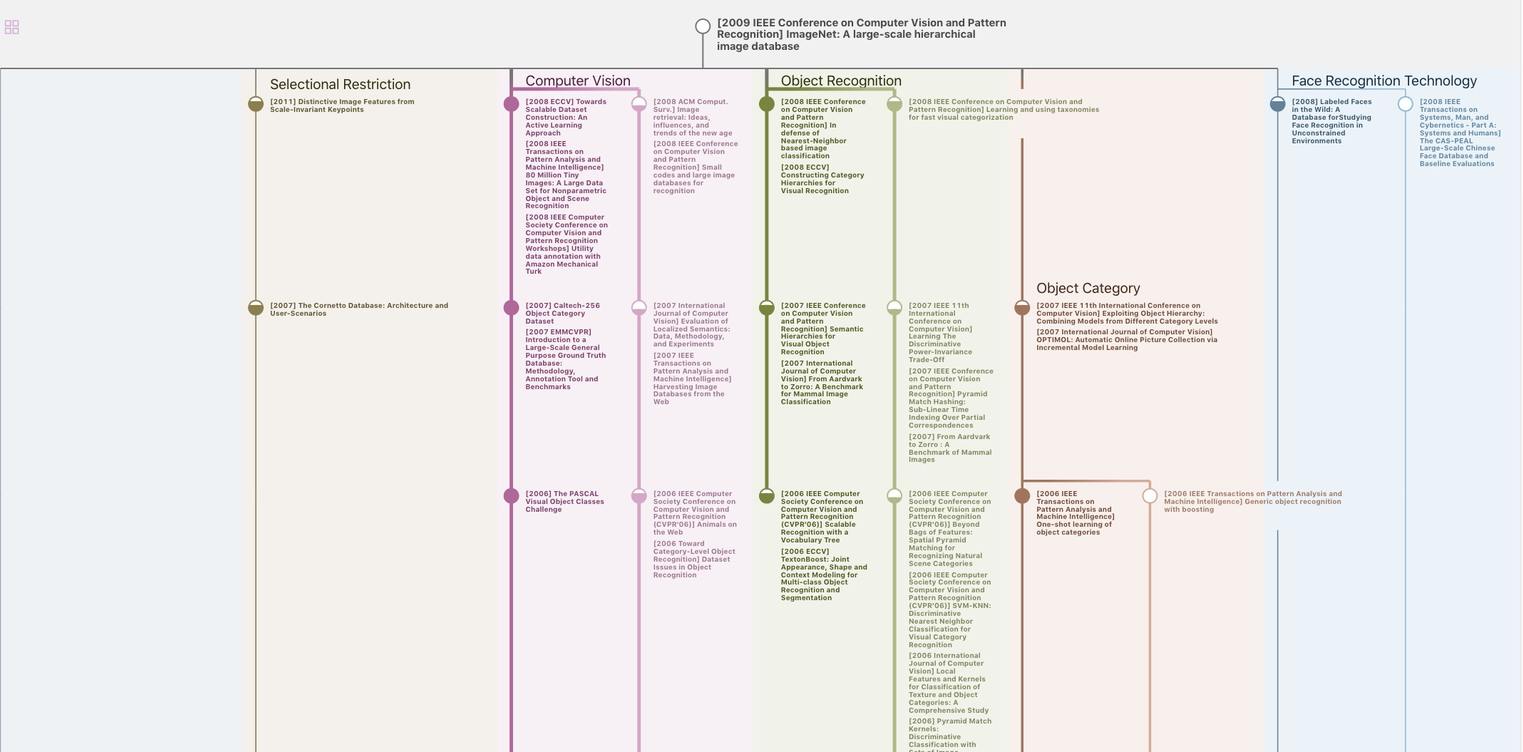
生成溯源树,研究论文发展脉络
Chat Paper
正在生成论文摘要