Global Stock Selection With Hidden Markov Model
RISKS(2021)
摘要
Hidden Markov model (HMM) is a powerful machine-learning method for data regime detection, especially time series data. In this paper, we establish a multi-step procedure for using HMM to select stocks from the global stock market. First, the five important factors of a stock are identified and scored based on its historical performances. Second, HMM is used to predict the regimes of six global economic indicators and find the time periods in the past during which these indicators have a combination of regimes that is similar to those predicted. Then, we analyze the five stock factors of the All country world index (ACWI) in the identified time periods to assign a weighted score for each stock factor and to calculate the composite score of the five factors. Finally, we make a monthly selection of 10% of the global stocks that have the highest composite scores. This strategy is shown to outperform those relying on either ACWI, any single stock factor, or the simple average of the five stock factors.
更多查看译文
关键词
global stocks, trading, machine learning, hidden Markov model, economics, regimes, stock ranking, stocks’, factors, economics indicators
AI 理解论文
溯源树
样例
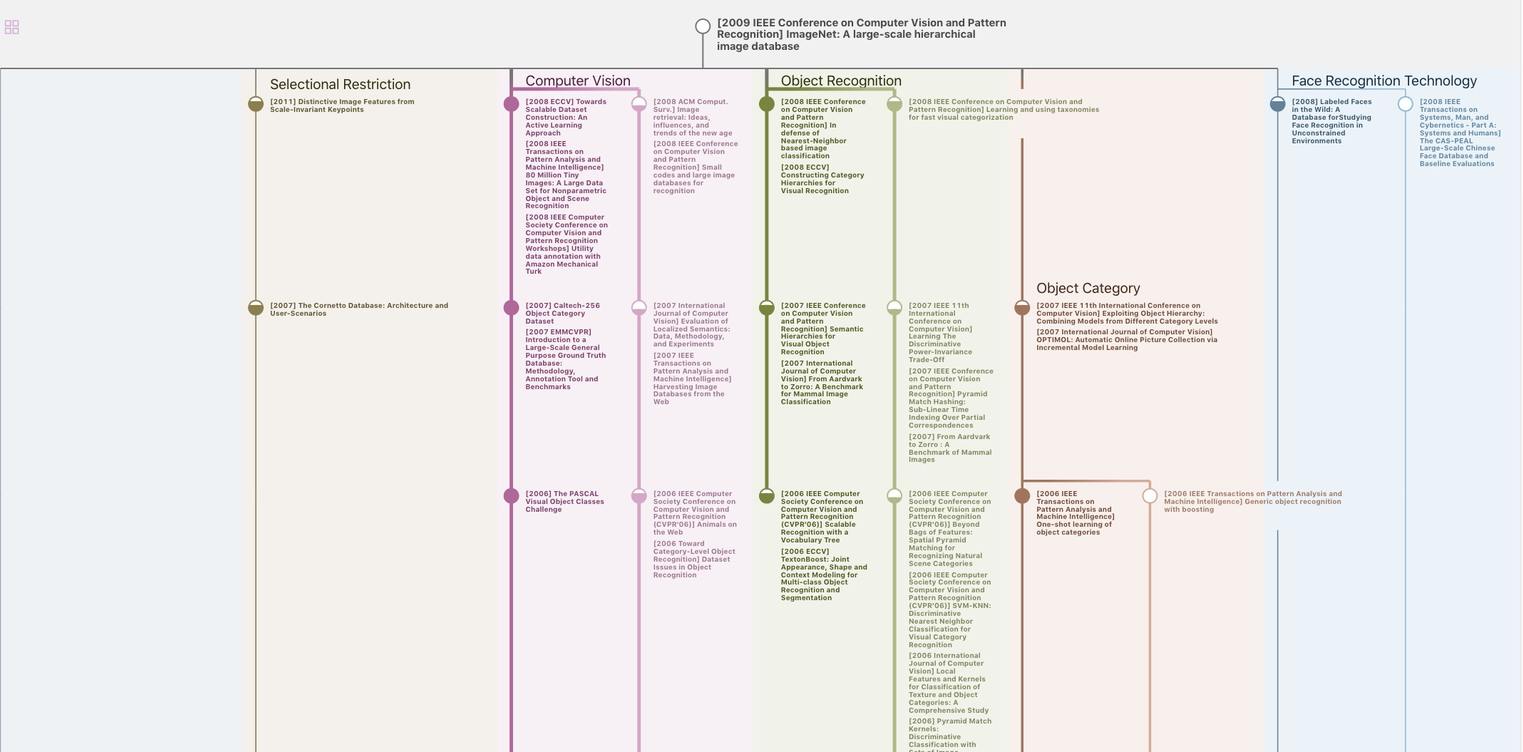
生成溯源树,研究论文发展脉络
Chat Paper
正在生成论文摘要