Learning for Crowdsourcing: Online Dispatch for Video Analytics with Guarantee
IEEE Conference on Computer Communications (INFOCOM)(2022)
摘要
Crowdsourcing enables a paradigm to conduct the manual annotation and the analytics by those recruited workers, with their rewards relevant to the quality of the results. Existing dispatchers fail to capture the resource-quality trade-off for video analytics, since the configurations supported by various workers are different, and the workers' availability is essentially dynamic. To determine the most suitable configurations as well as workers for video analytics, we formulate a non-linear mixed program in a long-term scope, maximizing the profit for the crowdsourcing platform. Based on previous results under various configurations and workers, we design an algorithm via a series of subproblems to decide the configurations adaptively upon the prediction of the worker rewards. Such prediction is based on volatile multi-armed bandit to capture the workers' availability and stochastic changes on resource uses. Via rigorous proof, the regret is ensured upon the Lyapunov optimization and the bandit, measuring the gap between the online decisions and the offline optimum. Extensive trace-driven experiments show that our algorithm improves the platform profit by 37%, compared with other algorithms.
更多查看译文
关键词
video analytics,nonlinear mixed program,crowdsourcing platform,worker rewards,online dispatch,manual annotation,resource-quality trade-off,crowdsourcing learning,rigorous proof,Lyapunov optimization,trace-driven experiments
AI 理解论文
溯源树
样例
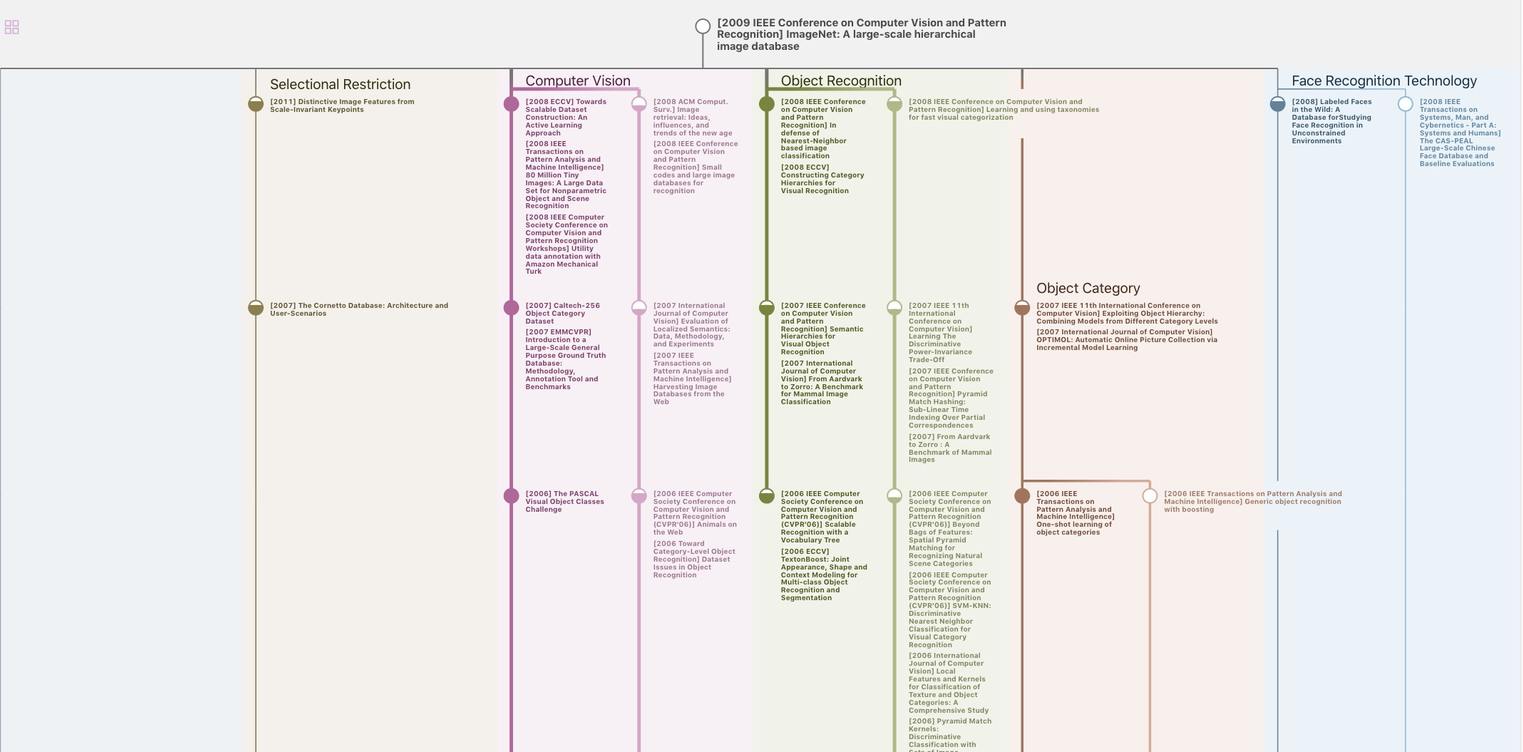
生成溯源树,研究论文发展脉络
Chat Paper
正在生成论文摘要