Dissecting tumor cell programs through group biology estimation in clinical single-cell transcriptomics
biorxiv(2021)
摘要
Given the growing number of clinically integrated cancer single-cell transcriptomic studies, robust differential enrichment methods for gene signatures to dissect tumor cellular states for discovery and translation are critical. Current analysis strategies neither adequately represent the hierarchical structure of clinical single-cell transcriptomic datasets nor account for the variability in the number of recovered cells per sample, leading to results potentially confounded by sample-driven biology with high false positives instead of accurately representing true differential enrichment of group-level biology (e.g., treatment responders vs. non-responders). This problem is especially prominent for single-cell analyses of the tumor compartment, because high intra-patient similarity (as opposed to inter-patient similarity) results in stricter hierarchical structured data that confounds enrichment analysis. Furthermore, to identify signatures which are truly representative of the entire group, there is a need to quantify the robustness of otherwise statistically significant signatures to sample exclusion. Here, we present a new nonparametric statistical method, BEANIE, to account for these issues, and demonstrate its utility in two cancer cohorts stratified by clinical groups to reduce biological hypotheses and guide translational investigations. Using BEANIE, we show how the consideration of sample-specific versus group biology greatly decreases the false positive rate and guides identification of robust signatures that can also be corroborated across different cell type compartments.
### Competing Interest Statement
The authors have declared no competing interest.
更多查看译文
关键词
tumor cell programs,group biology estimation,single-cell
AI 理解论文
溯源树
样例
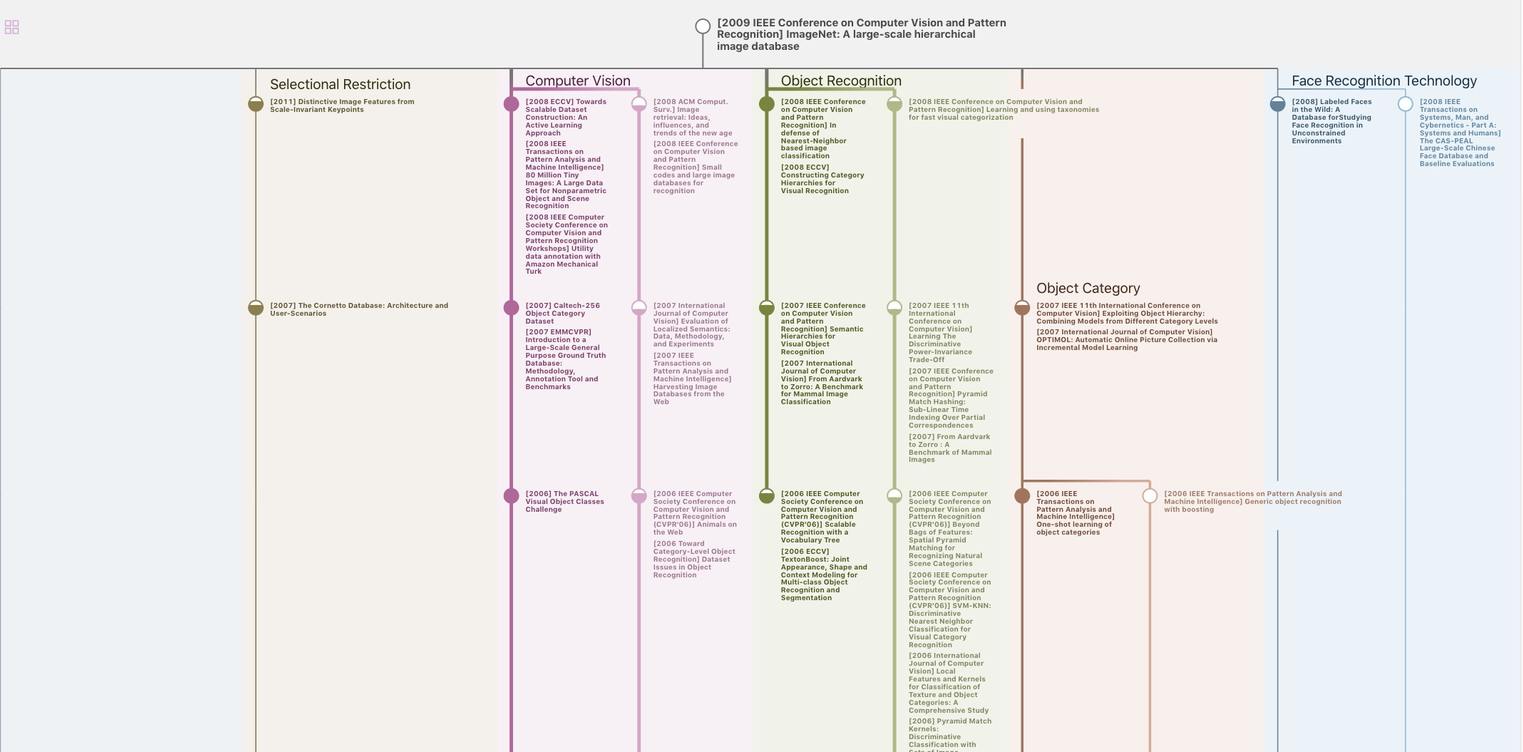
生成溯源树,研究论文发展脉络
Chat Paper
正在生成论文摘要