Assessing computational variant effect predictors via a prospective human cohort
biorxiv(2021)
摘要
Computational predictors can help interpret pathogenicity of human genetic variants, especially for the majority of variants where no experimental data are available. However, because we lack a high-quality unbiased test set, identifying the best-performing predictors remains a challenge. To address this issue, we evaluated missense variant effect predictors using genotypes and traits from a prospective cohort. We considered 139 gene-trait combinations with rare-variant burden association based on at least one of four systematic studies using phenotypes and whole-exome sequences from ~200K UK Biobank participants. Using an evaluation set of 35,525 rare missense variants and the relevant associated traits, we assessed the correlation of participants’ traits with scores derived from 20 computational variant effect predictors. We found that two predictors—VARITY and REVEL—outperformed all others according to multiple performance measures. We expect that this study will help in selecting variant effect predictors, for both research and clinical purposes, while providing an unbiased benchmarking strategy that can be applied to additional cohorts and predictors.
### Competing Interest Statement
F.P.R.is a scientific advisor and shareholder for Constantiam Biosciences and BioSymetrics, and a Ranomics shareholder. The authors declare no other competing interests.
更多查看译文
AI 理解论文
溯源树
样例
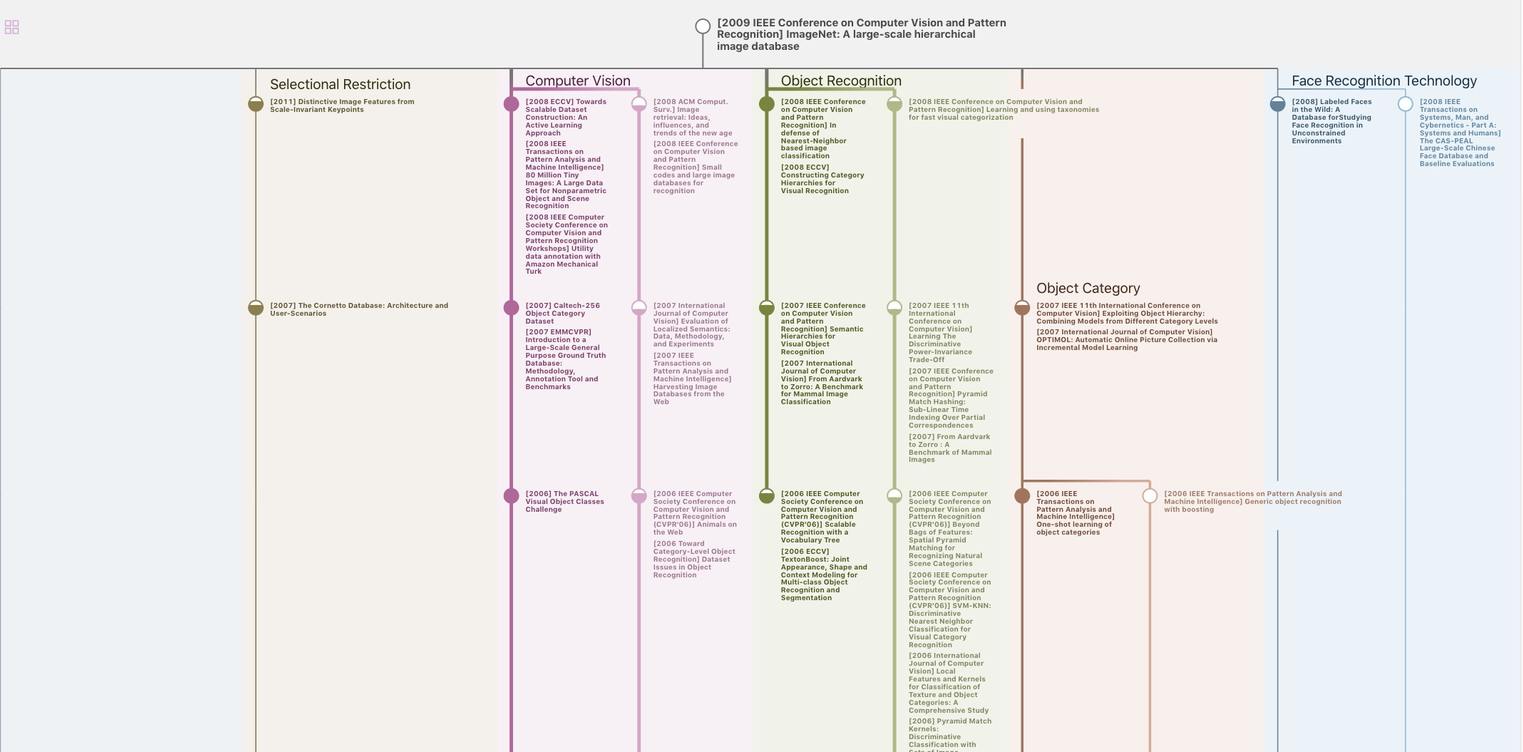
生成溯源树,研究论文发展脉络
Chat Paper
正在生成论文摘要