Individual Level Differential Expression Analysis for Single Cell RNA-seq data
Genome Biology(2021)
摘要
Bulk RNA-seq data quantify the expression of a gene in an individual by one number (e.g., fragment count). In contrast, single cell RNA-seq (scRNA-seq) data provide much richer information: the distribution of gene expression across many cells. To assess differential expression across individuals using scRNA-seq data, a straightforward solution is to create “pseudo” bulk RNA-seq data by adding up the fragment counts of a gene across cells for each individual, and then apply methods designed for differential expression using bulk RNA-seq data. This pseudo-bulk solution reduces the distribution of gene expression across cells to a single number and thus loses a good amount of information. We propose to assess differential expression using the gene expression distribution measured by cell level data. We find denoising cell level data can substantially improve the power of this approach. We apply our method, named IDEAS (Individual level Differential Expression Analysis for scRNA-seq), to study the gene expression difference between autism subjects and controls. We find neurogranin-expressing neurons harbor a high proportion of differentially expressed genes, and ERBB signals in microglia are associated with autism.
### Competing Interest Statement
The authors have declared no competing interest.
更多查看译文
AI 理解论文
溯源树
样例
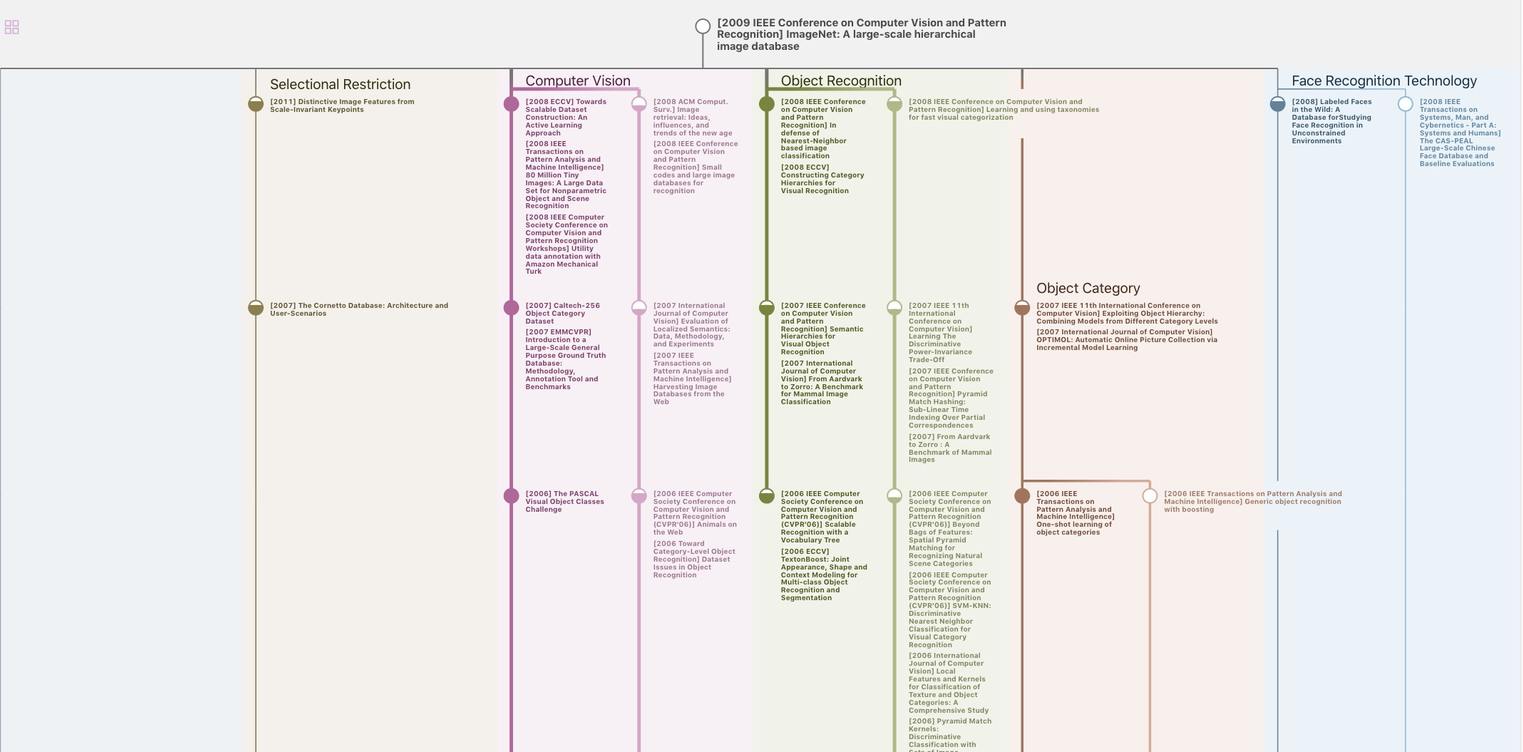
生成溯源树,研究论文发展脉络
Chat Paper
正在生成论文摘要