End-to-end learning of evolutionary models to find coding regions in genome alignments
Bioinformatics (Oxford, England)(2022)
摘要
Motivation: The comparison of genomes using models of molecular evolution is a powerful approach for finding, or toward understanding, functional elements. In particular, comparative genomics is a fundamental building brick in annotating ever larger sets of alignable genomes completely, accurately and consistently. Results: We here present our new program CIaMSA that classifies multiple sequence alignments using a phylogenetic model. It uses a novel continuous-time Markov chain machine learning layer, named CTMC, whose parameters are learned end-to-end and together with (recurrent) neural networks for a learning task. We trained CIaMSA discriminatively to classify aligned codon sequences that are candidates of coding regions into coding or non-coding and obtained four times fewer false positives for this task on vertebrate and fly alignments than existing methods at the same true positive rate. CIaMSA and the CTMC layer are general tools that could be used for other machine learning tasks on tree-related sequence data.
更多查看译文
关键词
genome,evolutionary models,learning,end-to-end
AI 理解论文
溯源树
样例
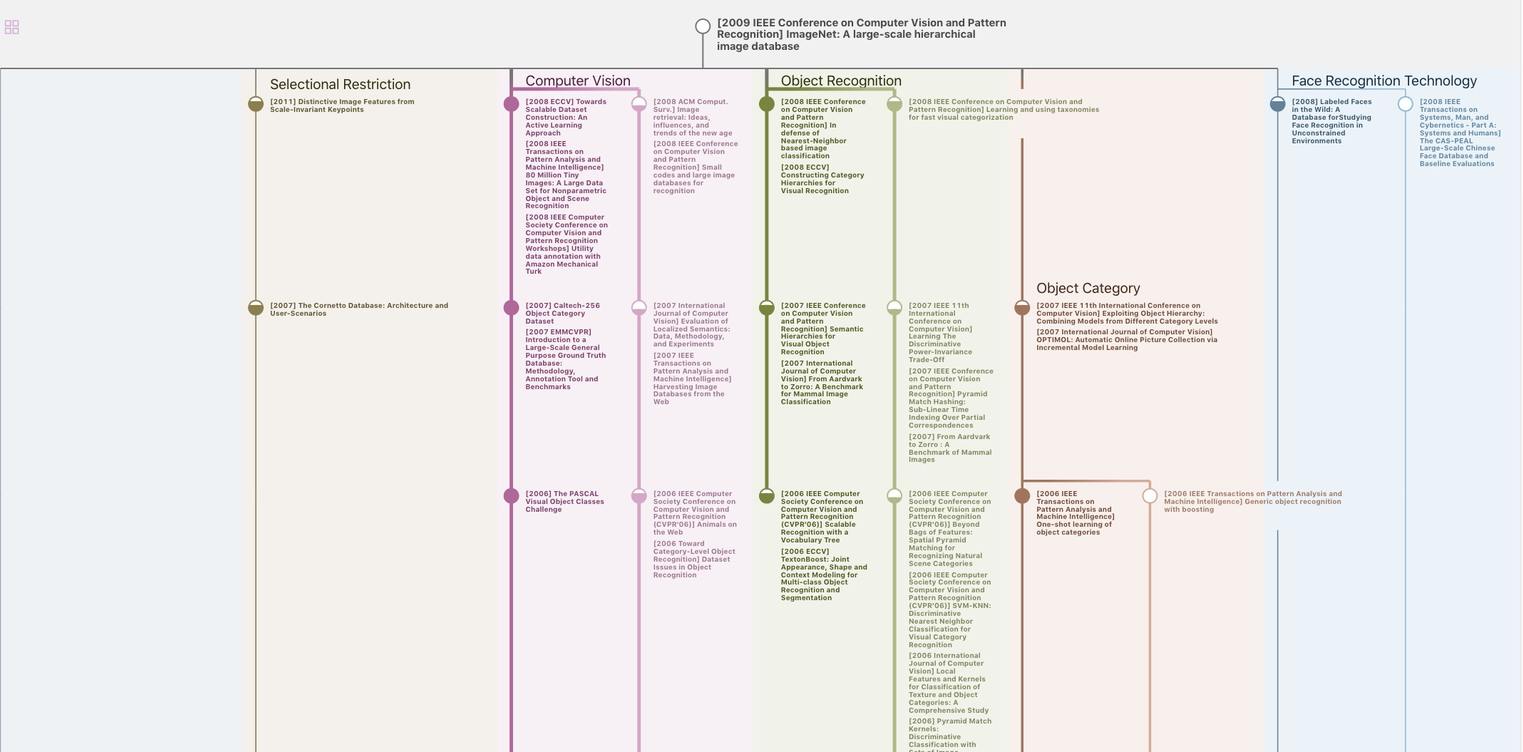
生成溯源树,研究论文发展脉络
Chat Paper
正在生成论文摘要