Single cell analysis of population-wide nuclear and cytosolic drug responses using high-content FRET imaging: measuring protein kinase activation in rat primary striatal neurons
biorxiv(2021)
摘要
Genetically-encoded biosensors are used to track biochemical activities in living cells by measuring changes in fluorescence emitted by one or more fluorescent proteins. In the present article, we describe the application of genetically-encoded FRET biosensors with high content microscopy to image the signaling responses of thousands of neurons in response to drug treatments. We applied this approach to reveal intercellular variation in signaling responses among cultured striatal neurons stimulated with multiple drugs. The striatum is largely composed of medium-spiny GABAergic neurons which are divided into two broad sub-types based in part on their expression of dopamine D1 vs. D2 receptors. Using high content FRET imaging and immunofluorescence, we identified neuronal sub-populations with unique responses to pharmacological manipulation. Focusing on dopamine- and glutamate-regulated PKA and ERK1/2 signaling in both the cytoplasm and nucleus, we identified pronounced intercellular differences, in both the magnitude and kinetics of signaling responses to drug application. Importantly, we found that a conventional “bulk” analysis that included all cells in culture yielded a different rank order of drug potency than that revealed by our single-cell analysis. The high degree of heterogeneity that we observed at the single cell level would not have been detectable using common population-level analyses, derived for example from western blotting or plate reader-based measurements. In conclusion, our single-cell analytical approach highlights the limitations of population-level analyses, and provides a novel way to study signaling biology.
### Competing Interest Statement
The authors have declared no competing interest.
更多查看译文
关键词
cytosolic drug responses,single cell analysis,protein kinase activation,neurons,population-wide,high-content
AI 理解论文
溯源树
样例
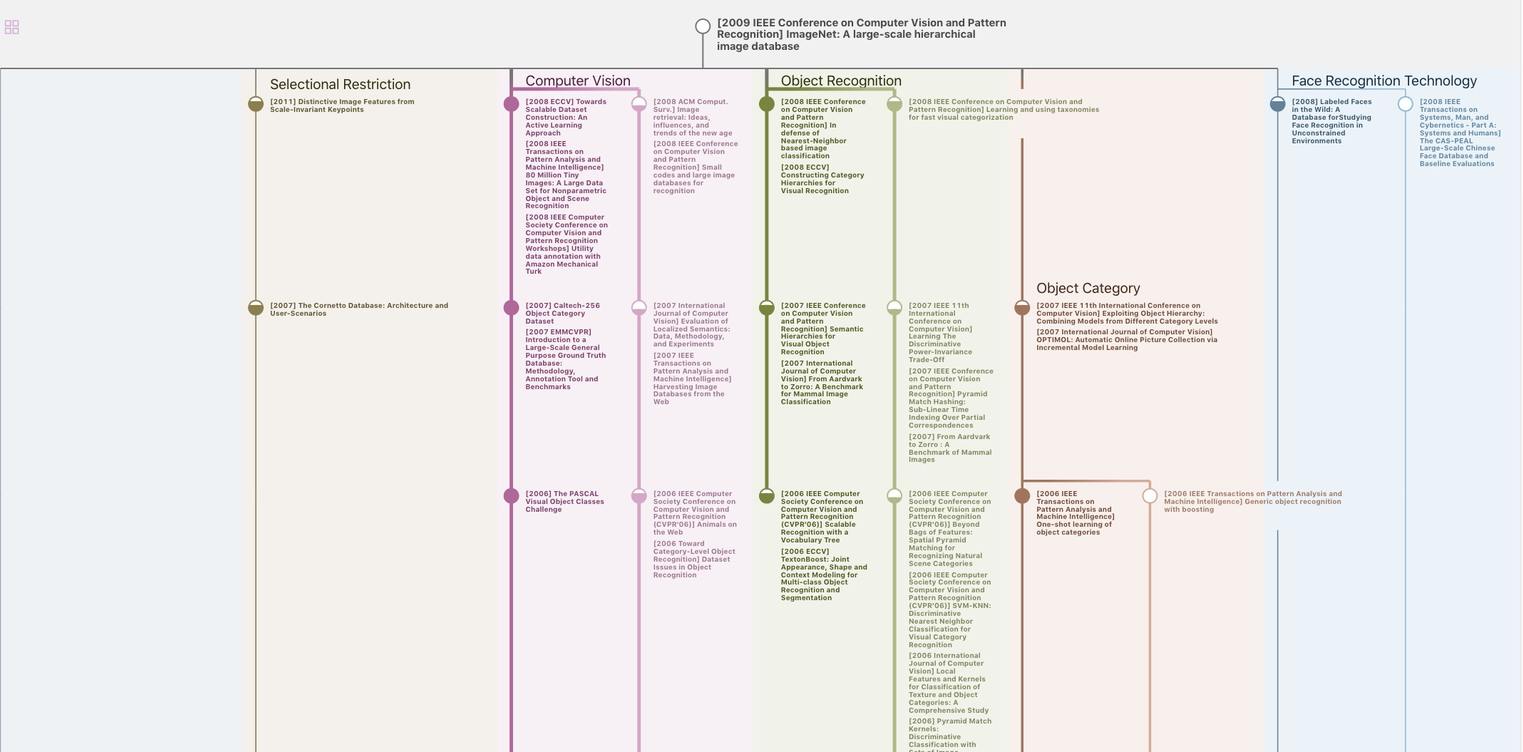
生成溯源树,研究论文发展脉络
Chat Paper
正在生成论文摘要