Improved YOLOv5 network-based object detection for anti-intrusion of gantry crane.
CCRIS(2021)
摘要
In response to the current lack of intelligence and security research on outdoor gantry cranes, the method based on the improved you-only-look-once (YOLO)v5 network for intelligent anti-intrusion detection is proposed. First an overall detection scheme is proposed. Then the following improvement tricks are made to the YOLOv5 network to achieve the highest possible detection accuracy while ensuring speed: incorporate multi-layer receptive fields and fine-grained modules into the backbone network to improve the performance of features; use dilated convolution to replace the pooling operation in the SPP module to reduce the loss of network information; further enrich the fusion of non-adjacent deep and shallow features in the network by using cross-layer connections; then use the K-means algorithm to cluster the target size to improve the positioning accuracy of the model; Finally, the non-maximum suppression algorithm is optimized by the weighting algorithm to effectively alleviate the inaccurate positioning of the YOLO series of bounding boxes. By combining multiple tricks, the improved YOLOv5s model can achieve a better balance between effectiveness (75.81% mAP) and efficiency (83 FPS) in anti-intrusion detection. At the same time, compared with the original YOLOv5s network on the VOC data set, the mAP value of the improved YOLOv5s is increased by 7.05%.
更多查看译文
AI 理解论文
溯源树
样例
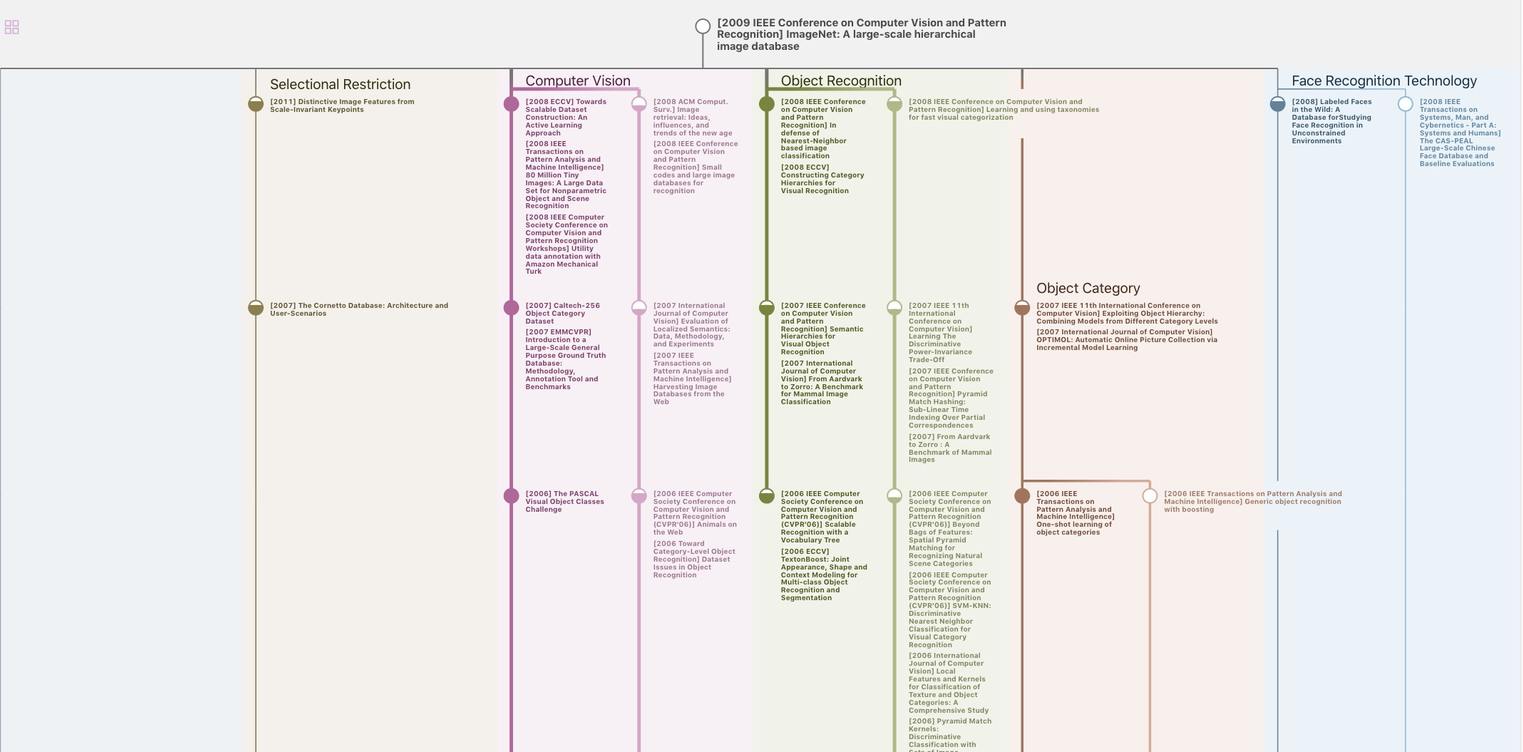
生成溯源树,研究论文发展脉络
Chat Paper
正在生成论文摘要