Error mitigation in variational quantum eigensolvers using tailored probabilistic machine learning
arxiv(2021)
摘要
Quantum computing technology has the potential to revolutionize the
simulation of materials and molecules in the near future. A primary challenge
in achieving near-term quantum advantage is effectively mitigating the noise
effects inherent in current quantum processing units (QPUs). This challenge is
also decisive in the context of quantum-classical hybrid schemes employing
variational quantum eigensolvers (VQEs) that have attracted significant
interest in recent years. In this work, we present a novel method that employs
parametric Gaussian process regression (GPR) within an active learning
framework to mitigate noise in quantum computations, focusing on VQEs. Our
approach, grounded in probabilistic machine learning, exploits a custom prior
based on the VQE ansatz to capture the underlying correlations between VQE
outputs for different variational parameters, thereby enhancing both accuracy
and efficiency. We demonstrate the effectiveness of our method on a 2-site
Anderson impurity model and a 8-site Heisenberg model, using the IBM
open-source quantum computing framework, Qiskit, showcasing substantial
improvements in the accuracy of VQE outputs while reducing the number of direct
QPU energy evaluations. This work contributes to the ongoing efforts in quantum
error mitigation and optimization, bringing us a step closer to realizing the
potential of quantum computing in quantum matter simulations.
更多查看译文
关键词
variational quantum eigensolvers,probabilistic machine learning,machine learning
AI 理解论文
溯源树
样例
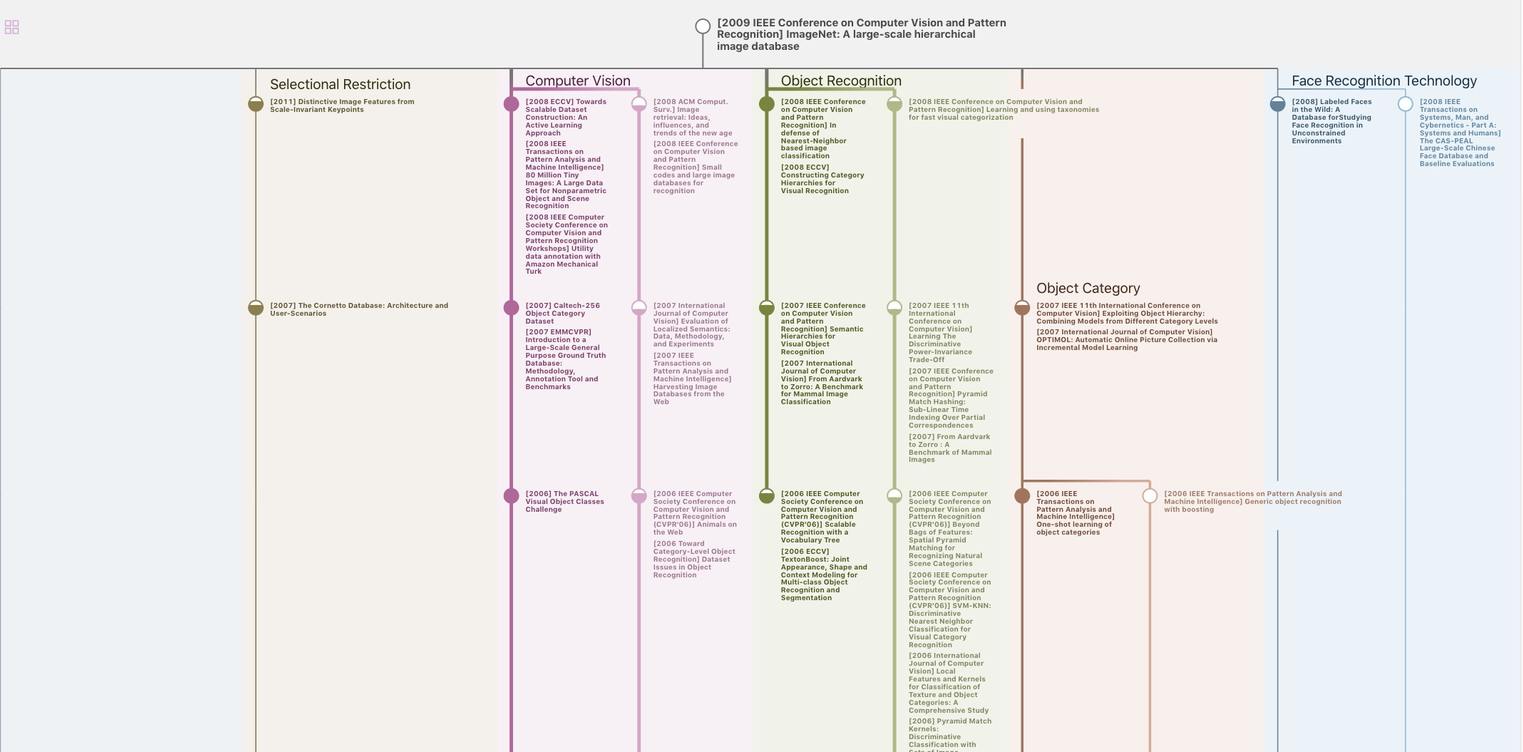
生成溯源树,研究论文发展脉络
Chat Paper
正在生成论文摘要