IntraTomo - Self-supervised Learning-based Tomography via Sinogram Synthesis and Prediction.
ICCV(2021)
摘要
We propose IntraTomo, a powerful framework that combines the benefits of learning-based and model-based approaches for solving highly ill-posed inverse problems in the Computed Tomography (CT) context. IntraTomo is composed of two core modules: a novel sinogram prediction module, and a geometry refinement module, which are applied iteratively. In the first module, the unknown density field is represented as a continuous and differentiable function, parameterized by a deep neural network. This network is learned, in a self-supervised fashion, from the incomplete or/and degraded input sinogram. After getting estimated through the sinogram prediction module, the density field is consistently refined in the second module using local and non-local geometrical priors. With these two core modules, we show that IntraTomo significantly outperforms existing approaches on several ill-posed inverse problems, such as limited angle tomography with a range of 45 degrees, sparse view tomographic reconstruction with as few as eight views, or super-resolution tomography with eight times increased resolution. The experiments on simulated and real data show that our approach can achieve results of unprecedented quality.
更多查看译文
关键词
Computational photography,Image and video synthesis,Optimization and learning methods,Stereo,3D from multiview and other sensors,Transfer/Low-shot/Semi/Unsupervised Learning
AI 理解论文
溯源树
样例
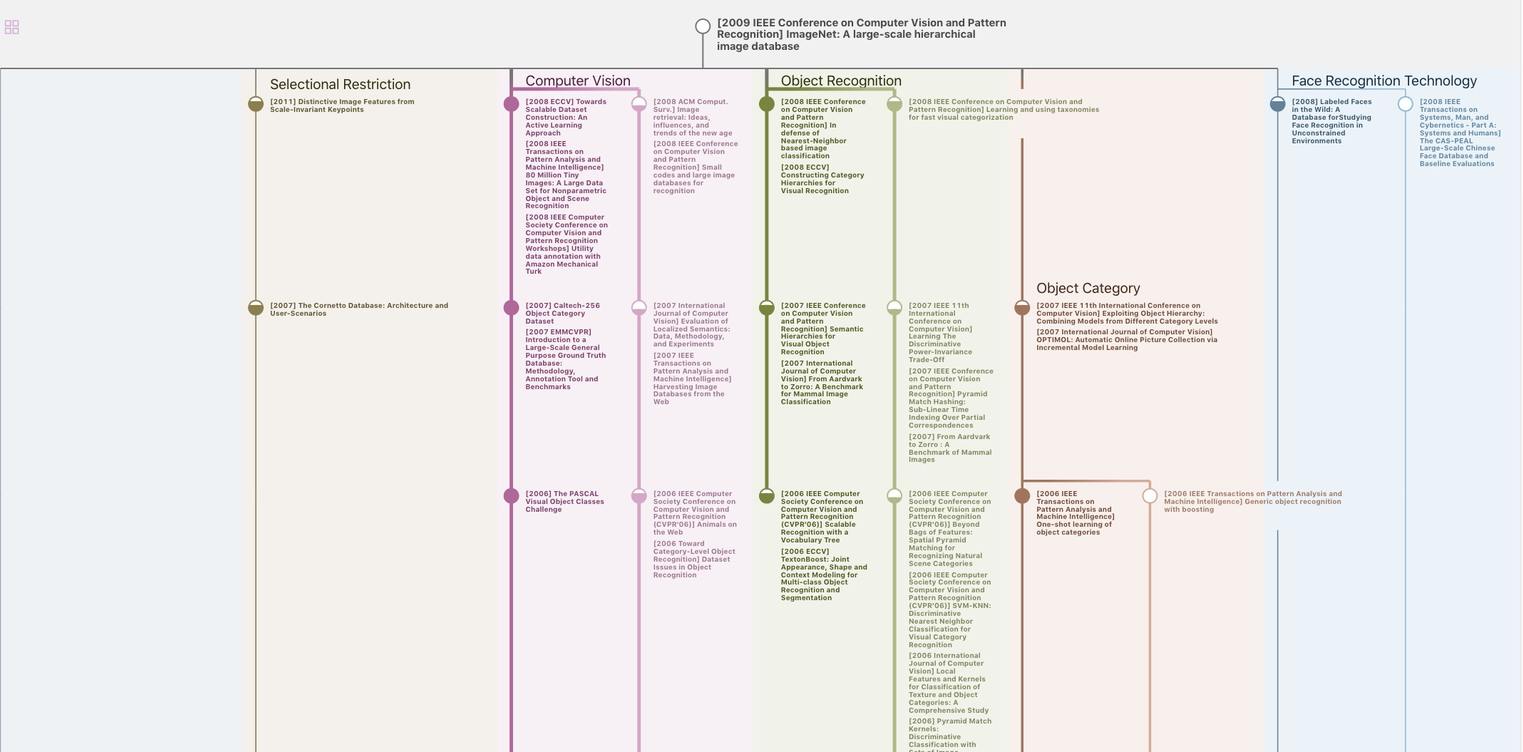
生成溯源树,研究论文发展脉络
Chat Paper
正在生成论文摘要