Relation-Aware Alignment Attention Network for Multi-view Multi-label Learning.
DASFAA (2)(2021)
摘要
Multi-View Multi-Label (MVML) learning refers to complex objects represented by multi-view features and associated with multiple labels simultaneously. Modeling flexible view consistency is recently demanded, yet existing approaches cannot fully exploit the complementary information across multiple views and meanwhile preserve view-specific properties. Additionally, each label has heterogeneous features from multiple views and probably correlates with other labels via common views. Traditional strategy tends to select features that are distinguishable for all labels. However, globally shared features cannot handle the label heterogeneity. Furthermore, previous studies model view consistency and label correlations independently, where interactions between views and labels are not fully exploited. In this paper, we propose a novel MVML learning approach named Relation-aware Alignment attention Network (RAIN), where three types of relationships are considered. Specifically, 1) view interactions: capture diverse and complementary information for deep correlated subspace learning; 2) label correlations: adopt multi-head attention to learn semantic label embedding; 3) label-view dependence: dynamically extracts label-specific representation with the guidance of learned label embedding. Experiments on various MVML datasets demonstrate the effectiveness of RAIN compared with state-of-the-arts. We also experiment on one real-world Herbs dataset, which shows promising results for clinical decision support.
更多查看译文
关键词
Multi-view multi-label,View interactions,Label correlations,Label-view dependence,Alignment attention
AI 理解论文
溯源树
样例
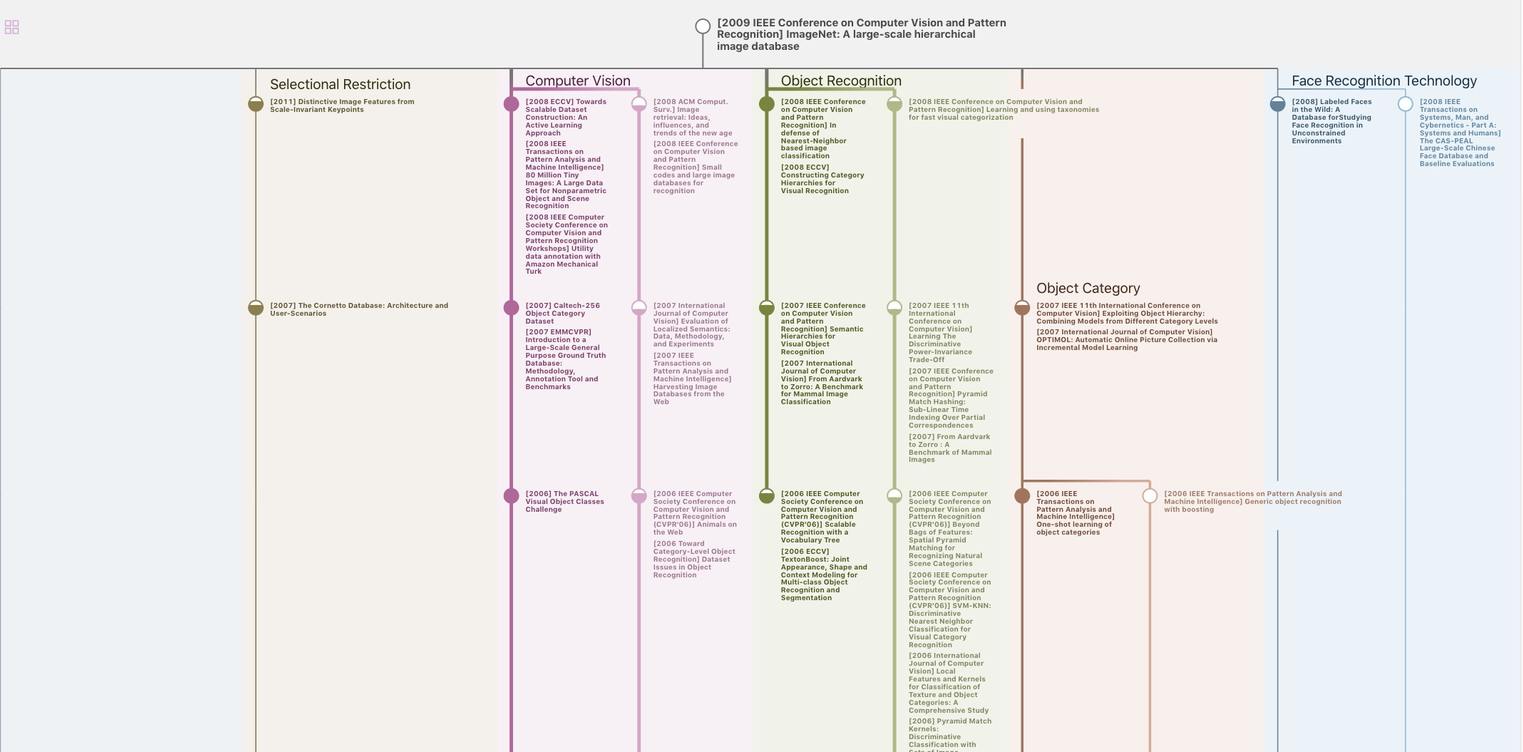
生成溯源树,研究论文发展脉络
Chat Paper
正在生成论文摘要