Dosimetric Planning Tradeoffs to Reduce Heart Dose Using Machine Learning-Guided Decision Support Software in Patients with Lung Cancer.
International journal of radiation oncology, biology, physics(2021)
摘要
PURPOSE:Cardiac toxicity is a well-recognized risk after radiation therapy (RT) in patients with non-small cell lung cancer (NSCLC). However, the extent to which treatment planning optimization can reduce mean heart dose (MHD) without untoward increases in lung dose is unknown.
METHODS AND MATERIALS:Retrospective analysis of RT plans from 353 consecutive patients with locally advanced NSCLC treated with intensity modulated RT (IMRT) or 3-dimensional conformal RT. Commercially available machine learning-guided clinical decision support software was used to match RT plans. A leave-one-out predictive model was used to examine lung dosimetric tradeoffs necessary to achieve a MHD reduction.
RESULTS:Of all 232 patients, 91 patients (39%) had RT plan matches showing potential MHD reductions of >4 to 8 Gy without violating the upper limit of lung dose constraints (lung volume [V] receiving 20 Gy (V20 Gy) <37%, V5 Gy <70%, and mean lung dose [MLD] <20 Gy). When switching to IMRT, 75 of 103 patients (72.8%) had plan matches demonstrating improved MHD (average 2.0 Gy reduction, P < .0001) without violating lung constraints. Examining specific lung dose tradeoffs, a mean ≥3.7 Gy MHD reduction was achieved with corresponding absolute increases in lung V20 Gy, V5 Gy, and MLD of 3.3%, 5.0%, and 1.0 Gy, respectively.
CONCLUSIONS:Nearly 40% of RT plans overall, and 73% when switched to IMRT, were predicted to have reductions in MHD >4 Gy with potentially clinically acceptable tradeoffs in lung dose. These observations demonstrate that decision support software for optimizing heart-lung dosimetric tradeoffs is feasible and may identify patients who might benefit most from more advanced RT technologies.
更多查看译文
AI 理解论文
溯源树
样例
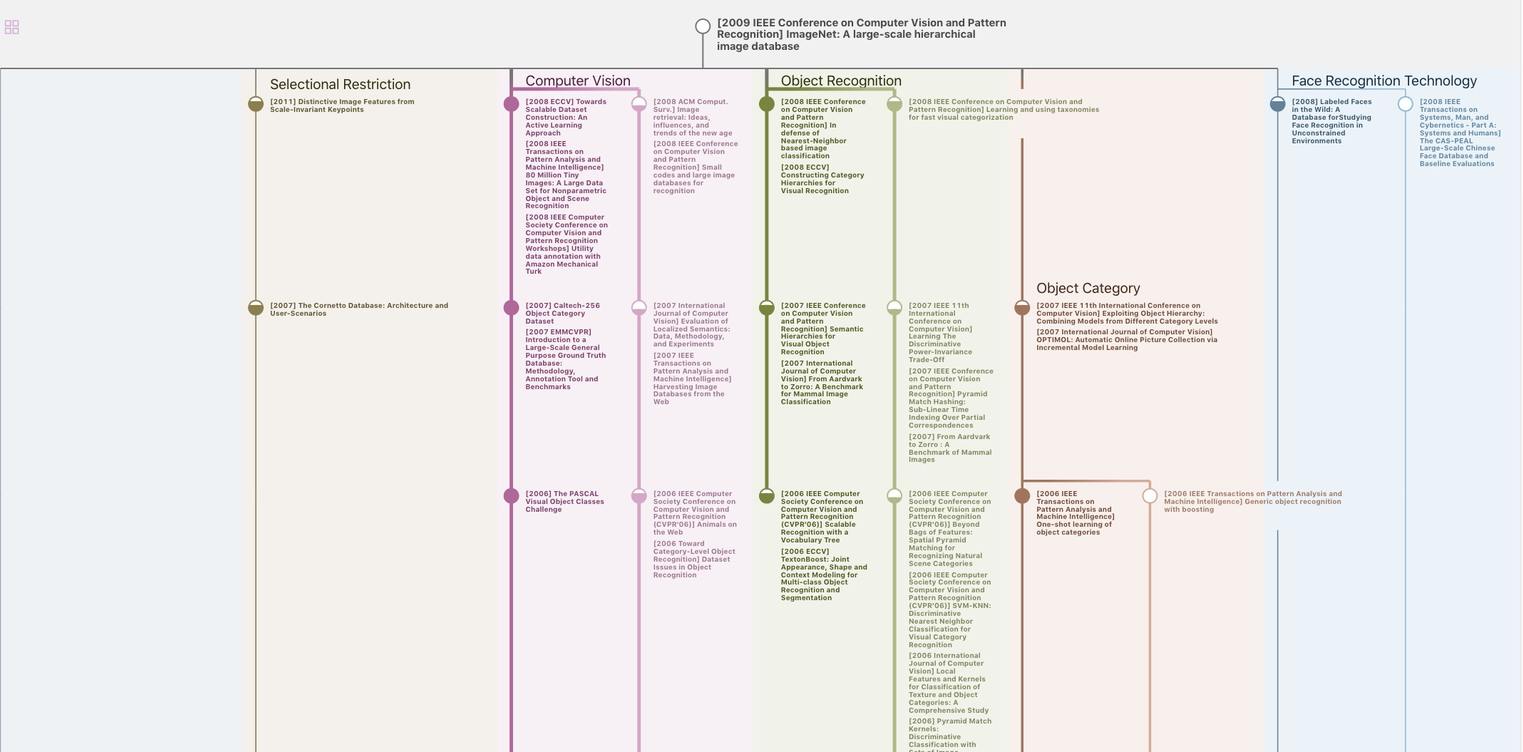
生成溯源树,研究论文发展脉络
Chat Paper
正在生成论文摘要