Pairwise interactions for potential energy surfaces and atomic forces using deep neural networks
Computational Materials Science(2022)
摘要
Molecular dynamics simulation, which is considered an important tool for studying physical and chemical processes at the atomic scale, requires accurate calculations of energies and forces. Although reliable energies and forces can be obtained by electronic structure calculations such as those based on density functional theory this approach is computationally expensive. In this study, we propose a full-stack model using a deep neural network (NN) to enhance the calculation of force and energy. The NN is designed to extract the embedding feature of pairwise interactions of an atom and its neighbors. These are aggregated to obtain its feature vector for predicting atomic force and potential energy. By designing the features of the pairwise interactions, we can control the performance of models. We also consider the many-body effects and other physics of the atomic interactions. Moreover, using the Coulomb matrix of the local structures in complement to the pairwise information, we can improve the prediction of force and energy for silicon systems. Furthermore, the transferability of our models to larger systems is confirmed with high accuracy.
更多查看译文
关键词
Force field,Deep learning,Materials informatics
AI 理解论文
溯源树
样例
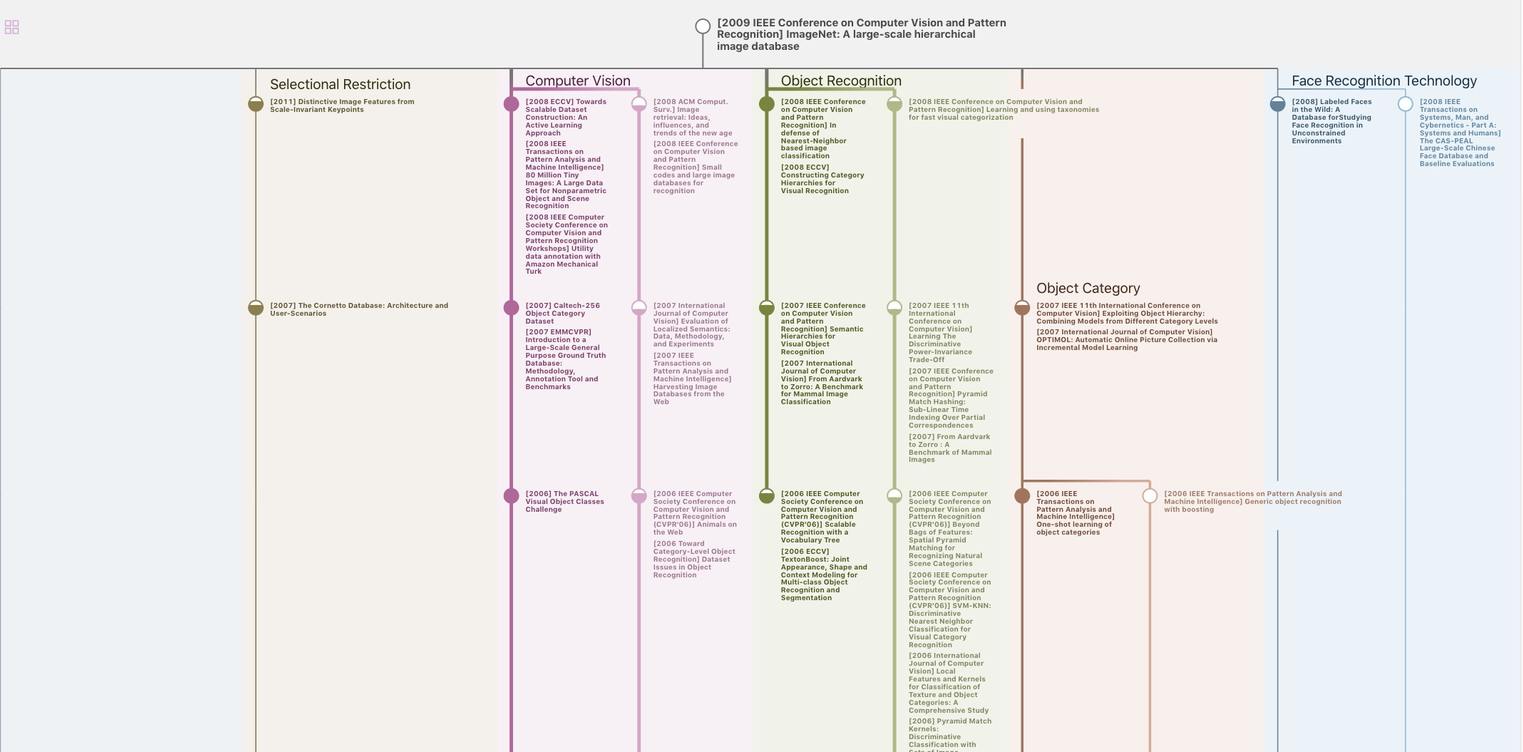
生成溯源树,研究论文发展脉络
Chat Paper
正在生成论文摘要