Practical multi-party private collaborative k-means clustering
Neurocomputing(2022)
摘要
k-means clustering is widely used in many fields such as data mining, machine learning, and information retrieval. In many cases, users need to cooperate to perform k-means clustering tasks. How to perform clustering without revealing privacy has become a hot research topic. However, the existing k-means scheme based on secure multi-party computation cannot effectively protect the privacy of the output results. The multi-party k-means scheme based on differential privacy may lead to loss of data availability. In this article, we propose a practical protocol for k-means clustering in a collaborative manner, while protecting the privacy of each data record. Our protocol is the first to combine secure multi-party computing and differential privacy technology to train a privacy-preserving k-means clustering model. We design a novel algorithm, which is suitable for multi-party collaboration to update cluster centers without leaking data privacy. The algorithm guarantees that noise is added only once in each iteration, regardless of the number of participants. The protocol achieve the ”best of both worlds”, which can simultaneously achieves both the input privacy and the output privacy in the k-means clustering scheme. Evaluation of real data sets shows that our scheme has comparable running time compared with the k-means clustering scheme without privacy protection.
更多查看译文
关键词
k-means clustering,Secure multi-party computation,Differential privacy,Privacy preserving
AI 理解论文
溯源树
样例
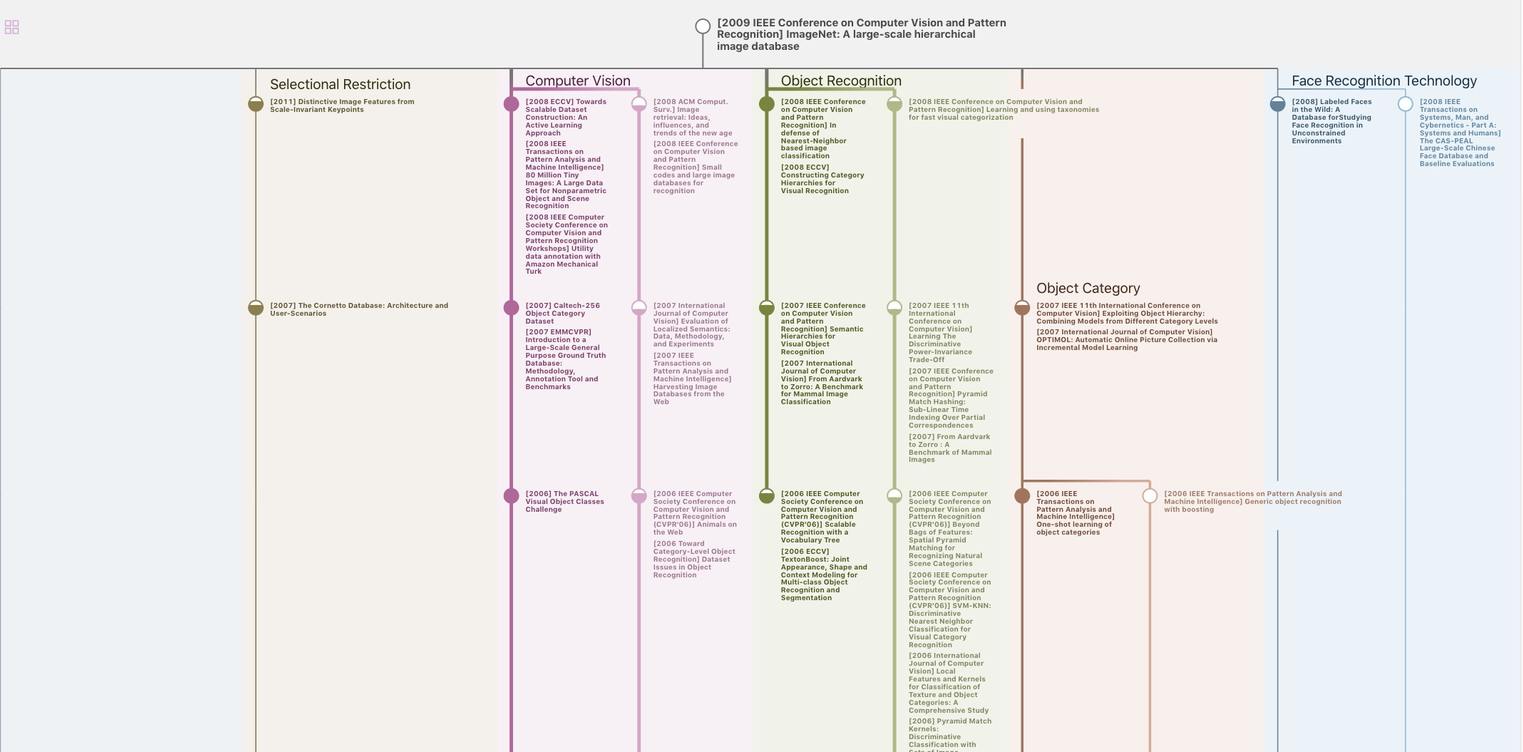
生成溯源树,研究论文发展脉络
Chat Paper
正在生成论文摘要