Neighbor Reweighted Local Centroid for Geometric Feature Identification
IEEE Transactions on Visualization and Computer Graphics(2023)
摘要
Identifying geometric features from sampled surfaces is a significant and fundamental task. The existing curvature-based methods that can identify ridge and valley features are generally sensitive to noise. Without requiring high-order differential operators, most statistics-based methods sacrifice certain extents of the feature descriptive powers in exchange for robustness. However, neither of these types of methods can treat the surface boundary features simultaneously. In this paper, we propose a novel neighbor reweighted local centroid (NRLC) computational algorithm to identify geometric features for point cloud models. It constructs a feature descriptor for the considered point via decomposing each of its neighboring vectors into two orthogonal directions. A neighboring vector starts from the considered point and ends with the corresponding neighbor. The decomposed neighboring vectors are then accumulated with different weights to generate the NRLC. With the defined NRLC, we design a probability set for each candidate feature point so that the convex, concave and surface boundary points can be recognized concurrently. In addition, we introduce a pair of feature operators, including assimilation and dissimilation, to further strengthen the identified geometric features. Finally, we test NRLC on a large body of point cloud models derived from different data sources. Several groups of the comparison experiments are conducted, and the results verify the validity and efficiency of our NRLC method.
更多查看译文
关键词
Feature identification,local centroid,convexity and concavity,surface boundary points,assimilation and dissimilation
AI 理解论文
溯源树
样例
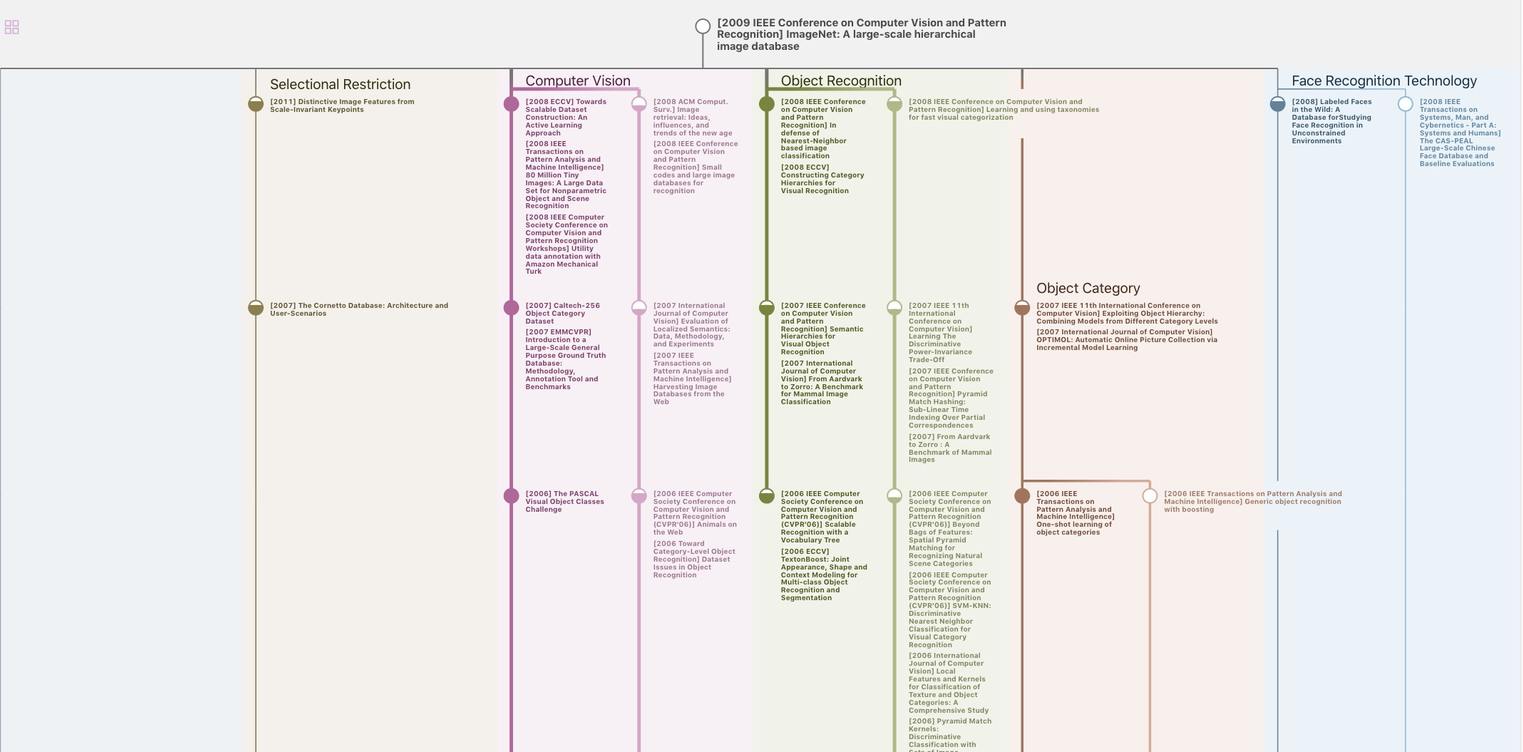
生成溯源树,研究论文发展脉络
Chat Paper
正在生成论文摘要