Modelling vegetation understory cover using LiDAR metrics
biorxiv(2019)
摘要
Forest understory vegetation is an important feature of wildlife habitat among other things. Predicting and mapping understory is a critical need for forest management and conservation planning, but it has proved difficult. LiDAR has the potential to generate remotely sensed forest understory structure data, yet this potential has to be fully validated. Our objective was to examine the capacity of LiDAR point cloud data to predict forest understory cover. We modeled ground-based observations of understory structure in three vertical strata (0.5 m to < 1.5 m, 1.5 m to < 2.5 m, 2.5 m to < 3.5 m) as a function of a variety of LiDAR metrics using both mixed-effects and Random Forest models. We compared four understory LiDAR metrics designed to control for the spatial heterogeneity of sampling density. The four metrics were highly correlated and they all produced high values of variance explained in mixed-effects models. The top-ranked model used a voxel-based understory metric along with vertical stratum (Akaike weight = 1, explained variance = 87%, SMAPE=15.6%). We found evidence of occlusion of LiDAR pulses in the lowest stratum but no evidence that the occlusion influenced the predictability of understory structure. The Random Forest model results were consistent with those of the mixed-effects models, in that all four understory LiDAR metrics were identified as important, along with vertical stratum. The Random Forest model explained 74.4% of the variance, but had a lower cross-validation error of 12.9%. Based on these results, we conclude that the best approach to predict understory structure is using the mixed-effects model with the voxel-based understory LiDAR metric along with vertical stratum, but that other understory LiDAR metrics (fractional cover, normalized cover and leaf area density) would still be effective in mixed-effects and Random Forest modelling approaches.
更多查看译文
AI 理解论文
溯源树
样例
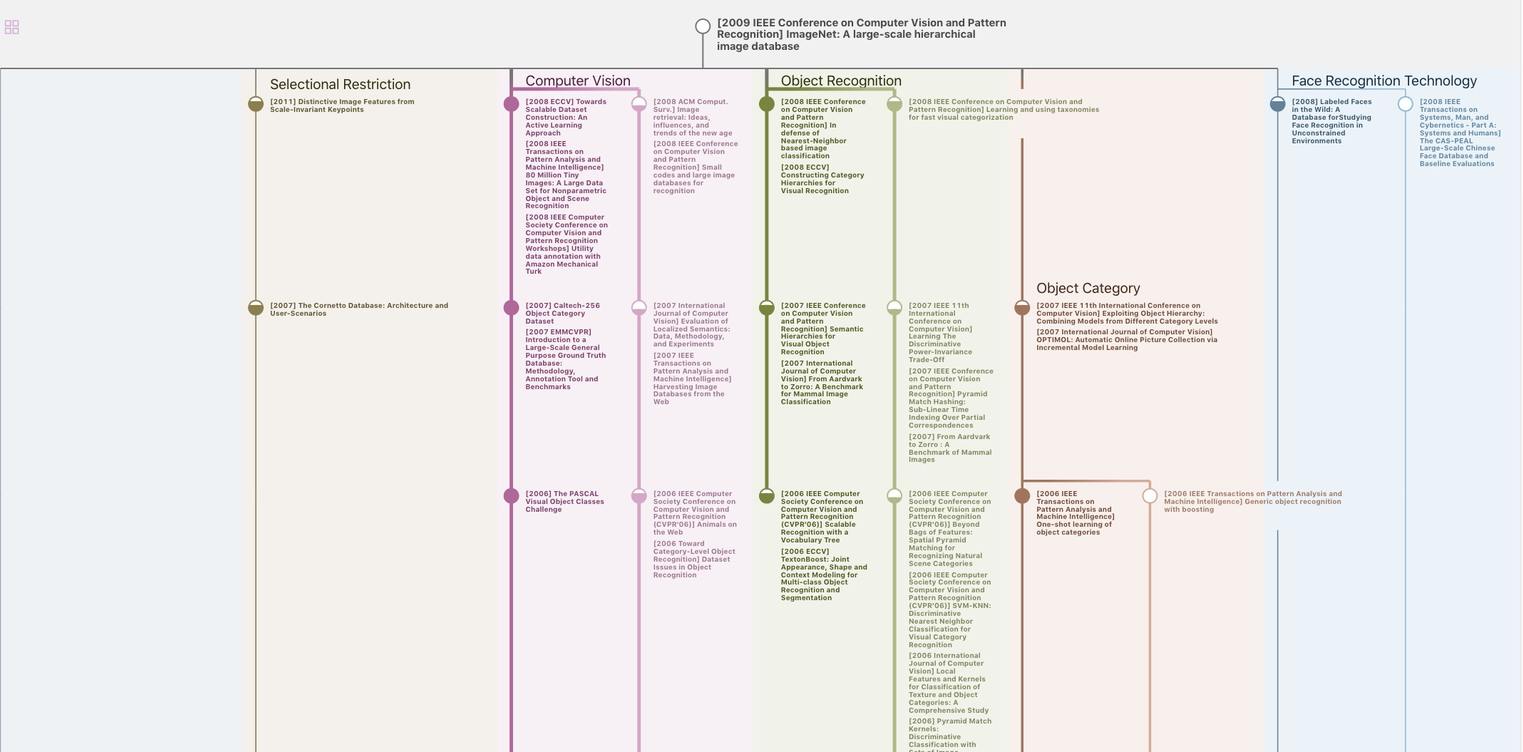
生成溯源树,研究论文发展脉络
Chat Paper
正在生成论文摘要