Hunter in the Dark: Deep Ensemble Networks for Discovering Anomalous Activity from Smart Networks
user-5dd52aee530c701191bf1b99(2021)
摘要
In modern networked society, smart networks are indispensable to offer intelligent communications and automated services to end-users and organizations. Machine learning (ML)-based network intrusion detection system (NIDS) plays a critical role in safeguarding smart networks against novel cyber threats. However, there are two challenges in the existing designs: 1) achieving an outstanding performance of threat detection often produces high false positives, leading to alert fatigue and 2) the interpretability of detection results is low, making a difficulty of understanding cyber threats and taking prompt actions against them. To tackle these challenges, in this paper, we propose a cyber defense mechanism, namely DarkHunter, which includes three new components: stream processor, detection engine and incident analyzer. The stream processor converts raw network packets into data records, including statistical features, which involve latent patterns of legitimates or anomalies to be effectively discovered using the detection engine. In this essence, the detection engine leverages an efficient ensemble neural network (EnsembleNet) to accurately identify anomalous traffic. Finally, the incident analyzer applies a correlation analysis to filter out the mispredictions from EnsembleNet, traces each detected threat from its statistical representation back to its source traffic flow to enhance its intelligibility and prioritizes the threats to be processed to minimize security risks. Our evaluations, based on the UNSW-NB15 dataset, show that DarkHunter significantly outperforms some state-of-the-art ML-based NIDSs by accomplishing higher accuracy, higher detection rate, higher precision, higher F1 score while keeping a lower false alarm rate.
更多查看译文
关键词
Network packet,Traffic flow (computer networking),Constant false alarm rate,False positive paradox,Artificial neural network,Stream processing,F1 score,Data mining,Interpretability,Computer science
AI 理解论文
溯源树
样例
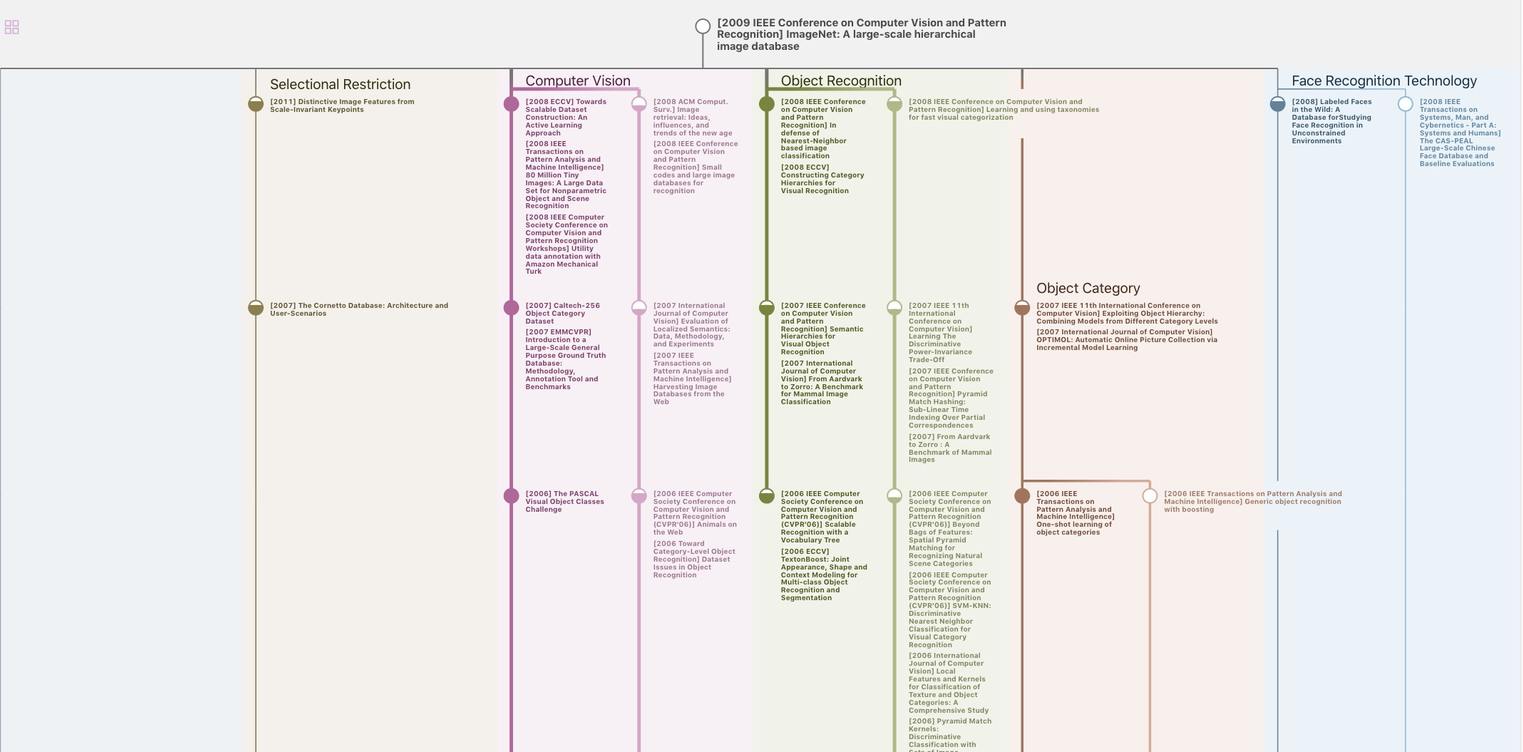
生成溯源树,研究论文发展脉络
Chat Paper
正在生成论文摘要